Deep Learning-based Retrieval of Daily 500-m Soil Moisture for South Korea
Journal of the Korean Cartographic Association(2017)
摘要
As soil moisture is an important hydro-meteorological factor which affects Earth’s energy budget and surface-atmosphere interaction, accurate observation of soil moisture and the understanding of spatio-temporal change is very important for Earth environmental studies. Point-based in-situ observation of soil moisture has favorable accuracy, but it does not have spatial continuity. Meanwhile, satellite observation provides spatially continuous dataset, but its accuracy and spatial resolution are not satisfactory. For quality improvement of soil moisture data in South Korea, we developed a deep learning-based retrieval model to produce daily 500-m soil moisture content by using satellite and meteorological dataset. Through 200 iterations of training-validation experiment, our deep learning model yielded more favorable results than NASA’s target accuracy and showed a good agreement with the in-situ observations. We built maps for soil moisture distribution of South Korea, which can support applications in agriculture, forest, hydrology, and disaster.
更多查看译文
关键词
Soil Moisture
AI 理解论文
溯源树
样例
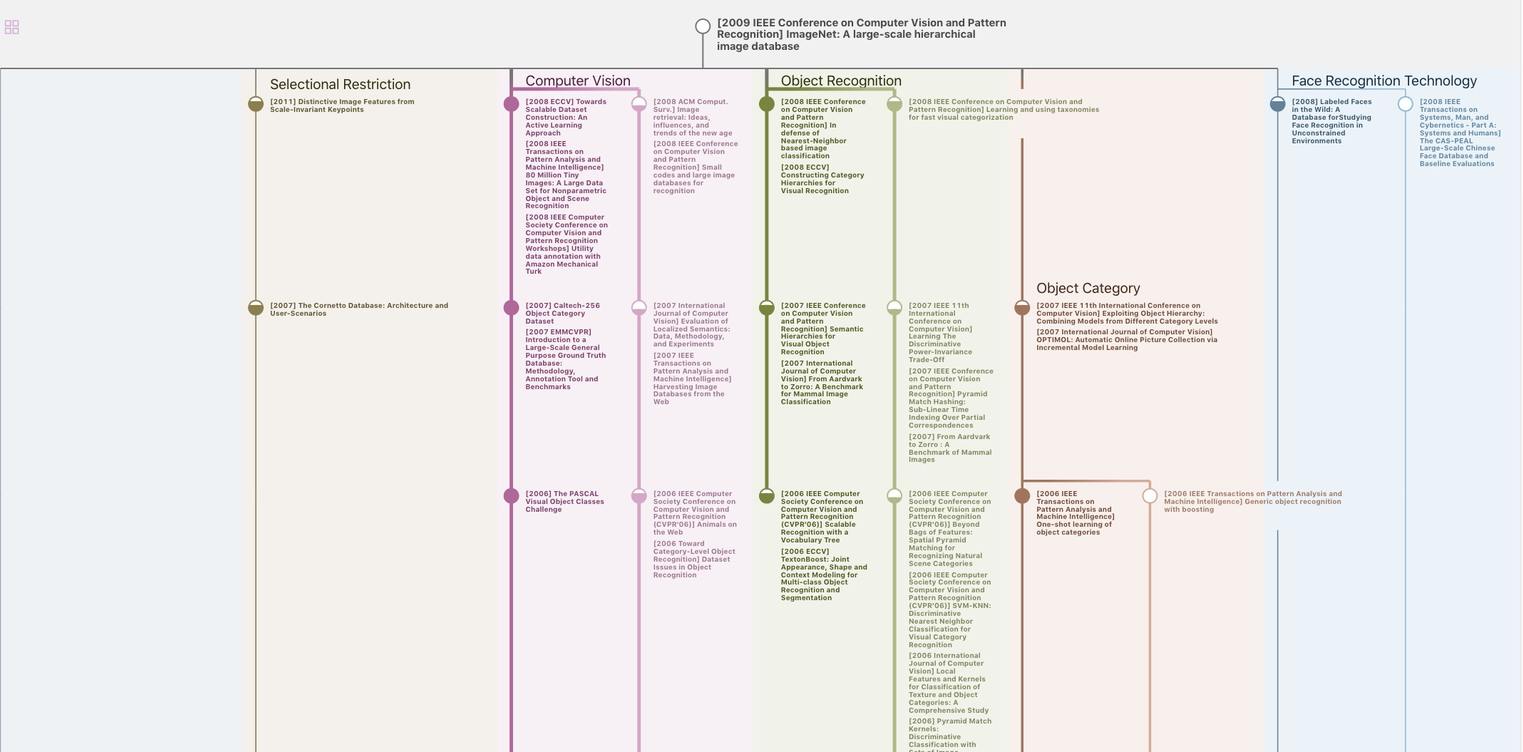
生成溯源树,研究论文发展脉络
Chat Paper
正在生成论文摘要