Tighter Expected Generalization Error Bounds Via Convexity of Information Measures
2022 IEEE International Symposium on Information Theory (ISIT)(2022)
摘要
Generalization error bounds are essential to understanding machine learning algorithms. This paper presents novel expected generalization error upper bounds based on the average joint distribution between the output hypothesis and each input training sample. Multiple generalization error upper bounds based on different information measures are provided, including Wasserstein distance, total variation distance, KL divergence, and Jensen-Shannon divergence. Due to the convexity of the information measures, the proposed bounds in terms of Wasserstein distance and total variation distance are shown to be tighter than their counterparts based on individual samples in the literature. An example is provided to demonstrate the tightness of the proposed generalization error bounds.
更多查看译文
关键词
expected multiple generalization error upper bounds,information measures,machine learning algorithms,total variation distance,Wasserstein distance
AI 理解论文
溯源树
样例
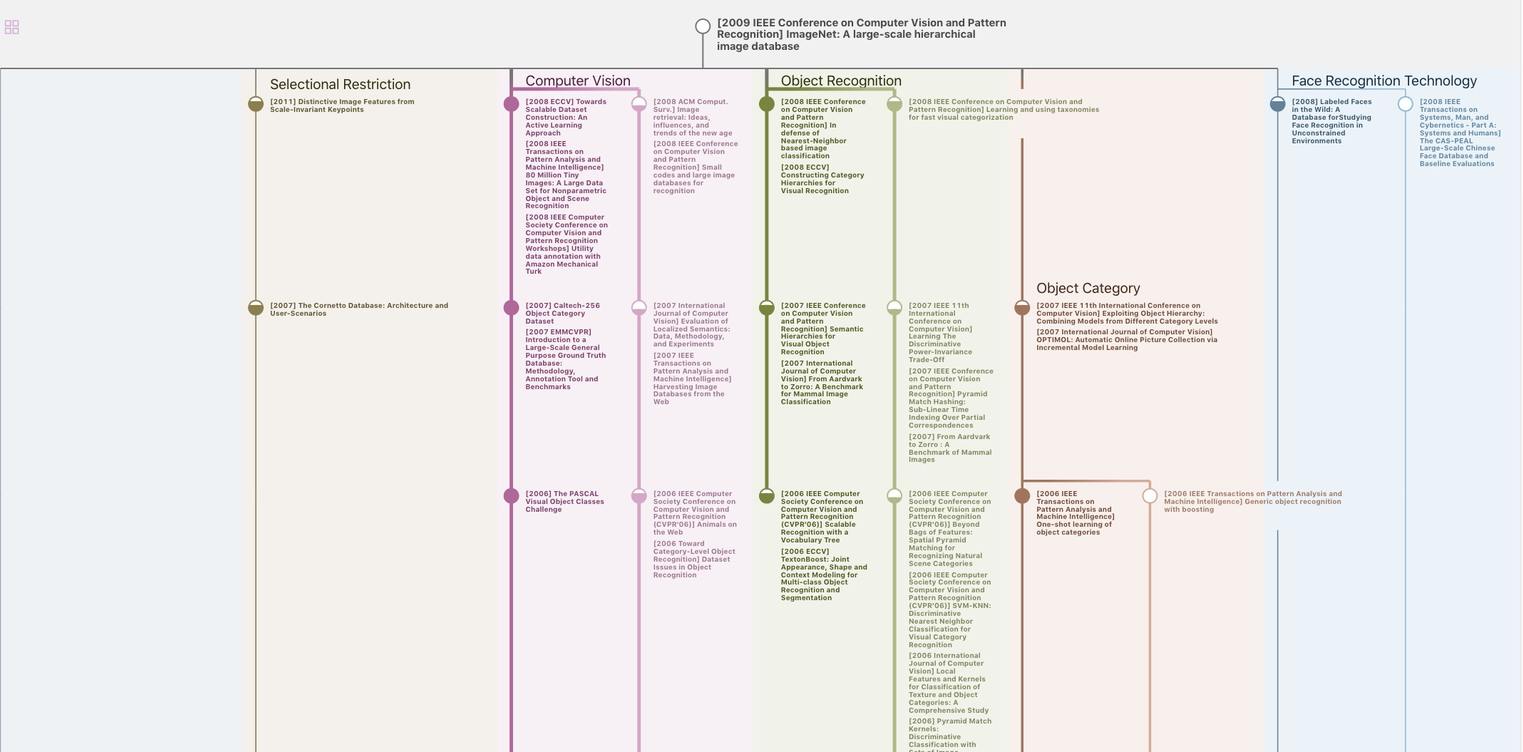
生成溯源树,研究论文发展脉络
Chat Paper
正在生成论文摘要