Human Reliability Assessment
Risk Assessment and Management for Ships and Offshore Structures(2024)
关键词
Human Reliability Analysis,Uncertainty Handling
AI 理解论文
溯源树
样例
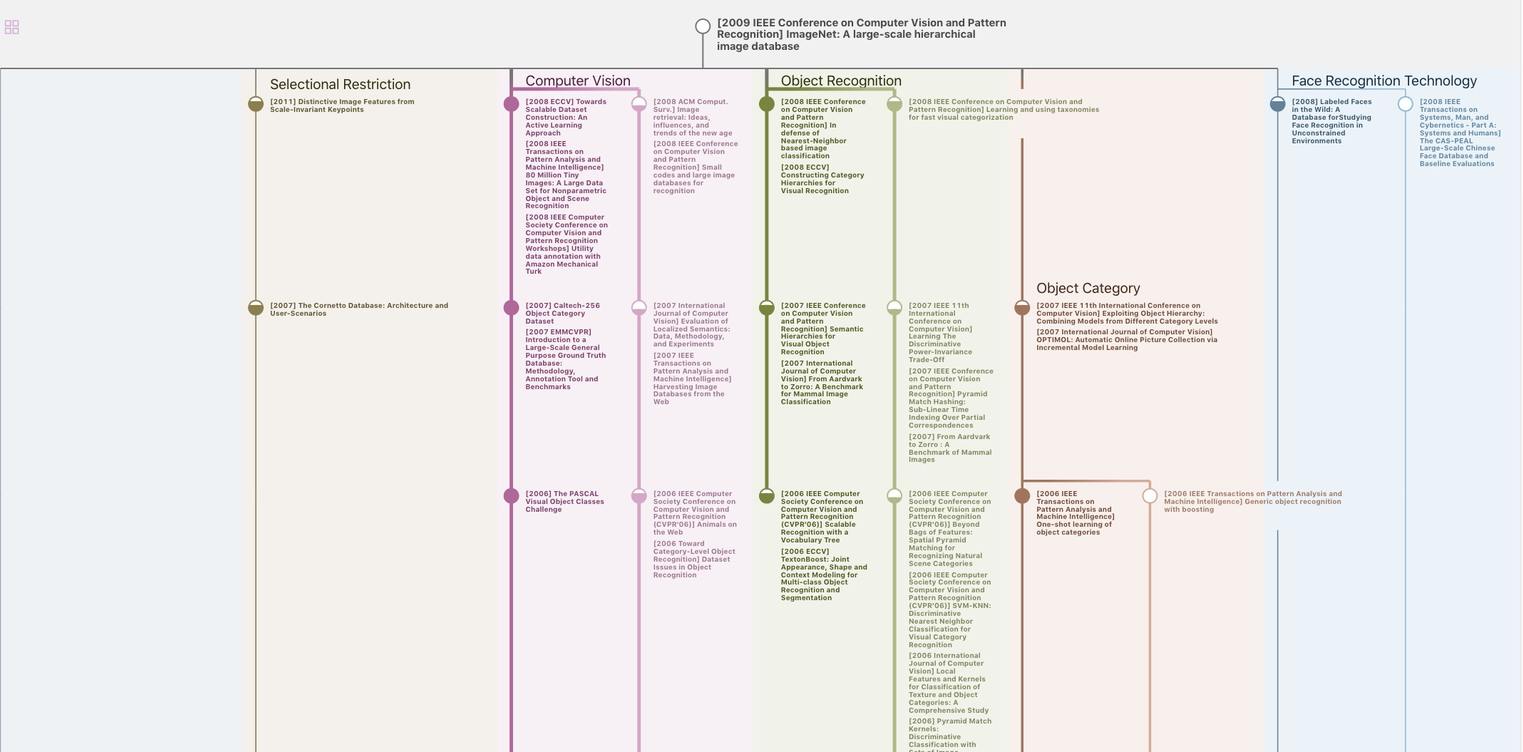
生成溯源树,研究论文发展脉络
Chat Paper
正在生成论文摘要
Risk Assessment and Management for Ships and Offshore Structures(2024)