Distributed Randomized Sketching Kernel Learning.
AAAI Conference on Artificial Intelligence(2022)
摘要
We investigate the statistical and computational requirements for distributed kernel ridge regression with randomized sketching (DKRR-RS) and successfully achieve the optimal learning rates with only a fraction of computations. More precisely, the proposed DKRR-RS combines sparse randomized sketching, divide-and-conquer and KRR to scale up kernel methods and successfully derives the same learning rate as the exact KRR with greatly reducing computational costs in expectation, at the basic setting, which outperforms previous state of the art solutions. Then, for the sake of the gap between theory and experiments, we derive the optimal learning rate in probability for DKRR-RS to reflect its generalization performance. Finally, to further improve the learning performance, we construct an efficient communication strategy for DKRR-RS and demonstrate the power of communications via theoretical assessment. An extensive experiment validates the effectiveness of DKRR-RS and the communication strategy on real datasets.
更多查看译文
关键词
Machine Learning (ML)
AI 理解论文
溯源树
样例
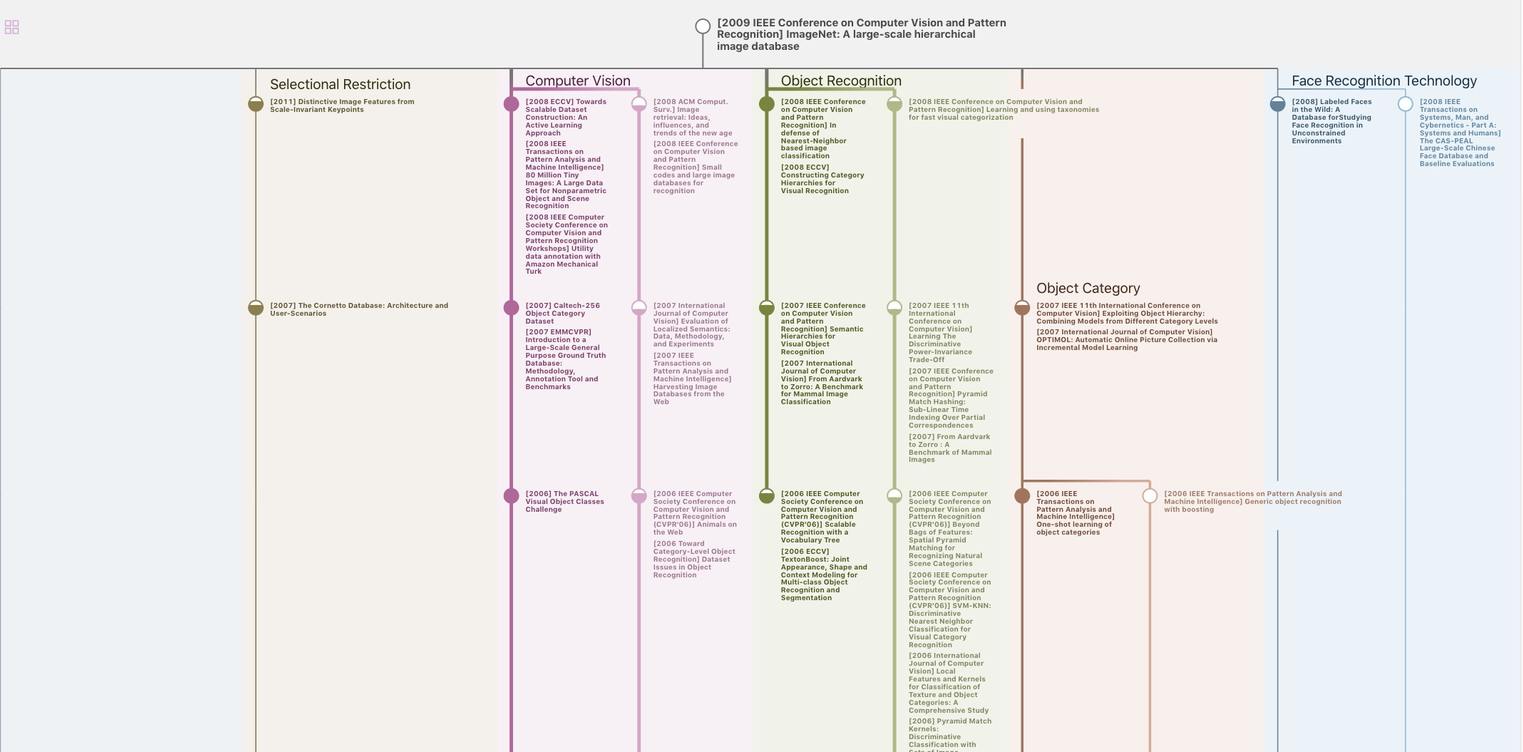
生成溯源树,研究论文发展脉络
Chat Paper
正在生成论文摘要