FIBER-ML, an Open-Source Supervised Machine Learning Tool for Quantification of Fibrosis in Tissue Sections.
American Journal Of Pathology(2022)
关键词
Accepted for publication,Address correspondence to,Cardiovascular Research Center
AI 理解论文
溯源树
样例
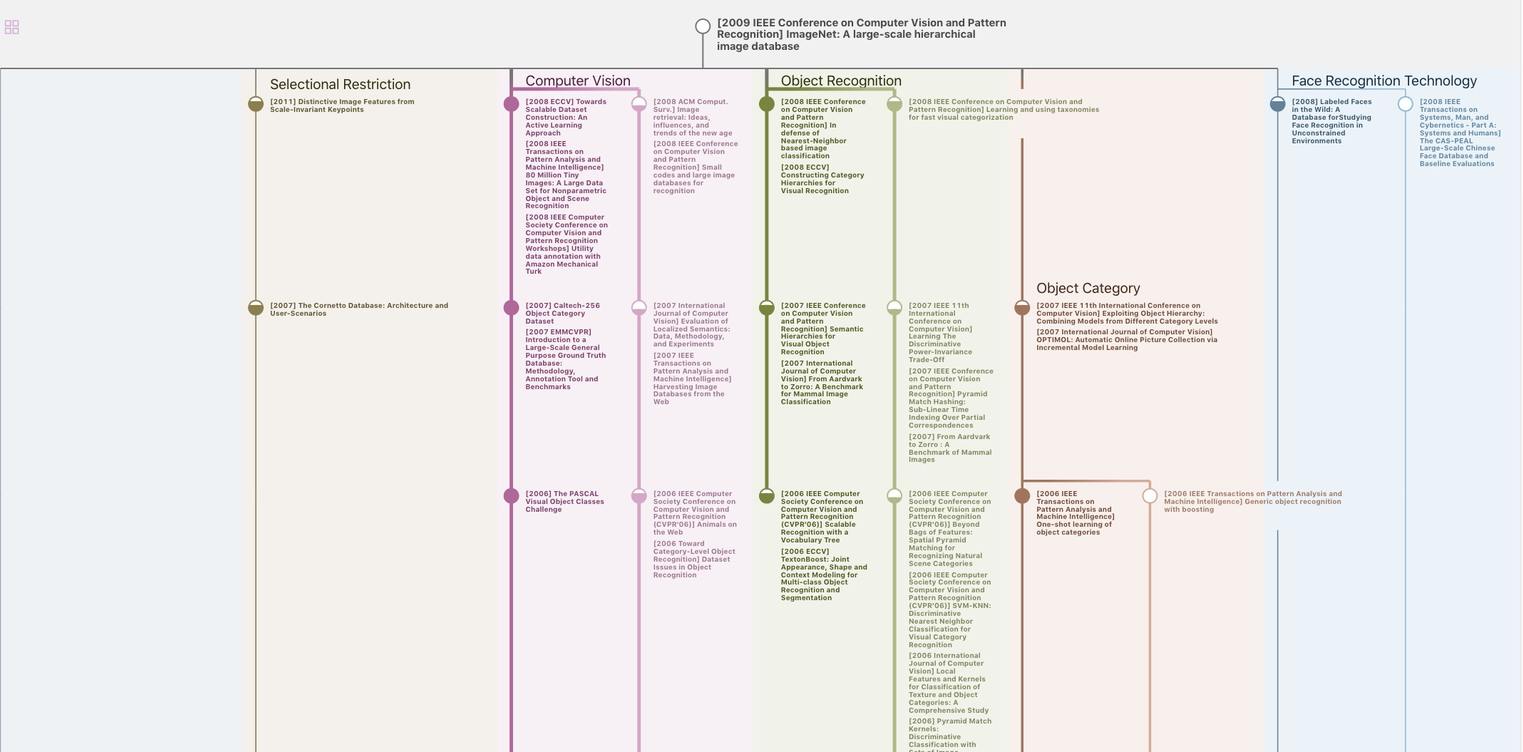
生成溯源树,研究论文发展脉络
Chat Paper
正在生成论文摘要