Mobile Traffic Forecasting for Green 5G Networks
2021 IEEE Global Communications Conference (GLOBECOM)(2021)
摘要
The energy consumption and carbon footprint of the fifth-generation (5G) of mobile technology is a current concern to mobile network operators (MNOs). These are currently attempting to lower both their carbon emissions and electricity bills by investigating new schemes that allow adapting the network transmission capabilities to the end-users' quality of service (QoS) requirements. Many of such schemes rely on accurate traffic forecasting, and as a consequence, there is a large effort on investigating novel machine learning (ML) algorithms, which fed by network measurement data and empowered by the computing capabilities of dedicated hardware, can help modelling and predicting users' behaviours. Most of the works in the literature, however, focus on predicting the traffic when energy saving features, e.g. carrier shutdown, are not implemented or activated. However, the prediction task becomes much more challenging when energy saving features are adopted due to their impact to the actual measured traffic. In this paper, we consider a scenario in which part of the base stations implement energy saving schemes, which allow them to dynamically switch off part of their hardware to reduce their power consumption. Then, we present a ML framework based on graph convolutional networks (GCNs) for traffic forecasting in such dynamic scenarios, and compare its performance with other statistical and ML prediction algorithms. The proposed GCN framework provides significant accuracy gains. Moreover, we provide an analysis of the impact of spatial correlation-captured by the GCN model-on the achieved performance.
更多查看译文
关键词
mobile traffic forecasting,green 5G networks,energy consumption,carbon footprint,mobile technology,mobile network operators,MNOs,carbon emissions,electricity bills,network transmission capabilities,end-users,quality of service requirements,QoS,accurate traffic forecasting,machine learning algorithms,network measurement data,carrier shutdown,actual measured traffic,base stations,power consumption,ML framework,graph convolutional networks,dynamic scenarios,statistical ML prediction algorithms
AI 理解论文
溯源树
样例
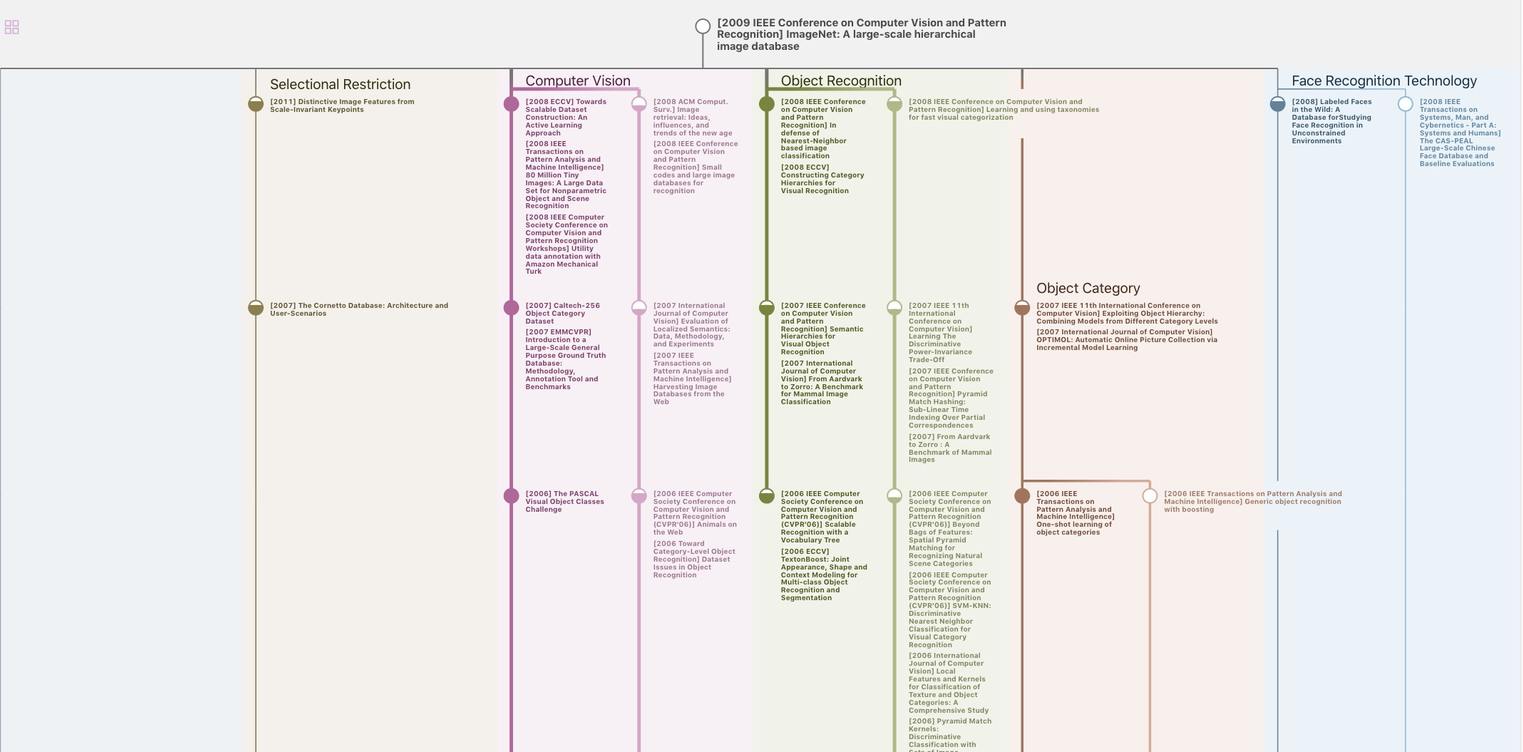
生成溯源树,研究论文发展脉络
Chat Paper
正在生成论文摘要