Learning to Ground Objects for Robot Task andMotion Planning
arxiv(2022)
摘要
Task and motion planning (TAMP) algorithms have been developed to help robots plan behaviors in discrete and continuous spaces. Robots face complex real-world scenarios, where it is hardly possible to model all objects or their physical properties for robot planning (e.g., in kitchens or shopping centers). In this paper, we define a new object-centric TAMP problem, where the TAMP robot does not know object properties (e.g., size and weight of blocks). We then introduce Task-Motion Object-Centric planning ({\bf TMOC}), a grounded TAMP algorithm that learns to ground objects and their physical properties with a physics engine. TMOC is particularly useful for those tasks that involve dynamic complex robot-multi-object interactions that can hardly be modeled beforehand. We have demonstrated and evaluated TMOC in simulation and using a real robot. Results show that TMOC outperforms competitive baselines from the literature in cumulative utility.
更多查看译文
关键词
Grounded planning, integrated planning and learning, robot manipulation, task and motion planning
AI 理解论文
溯源树
样例
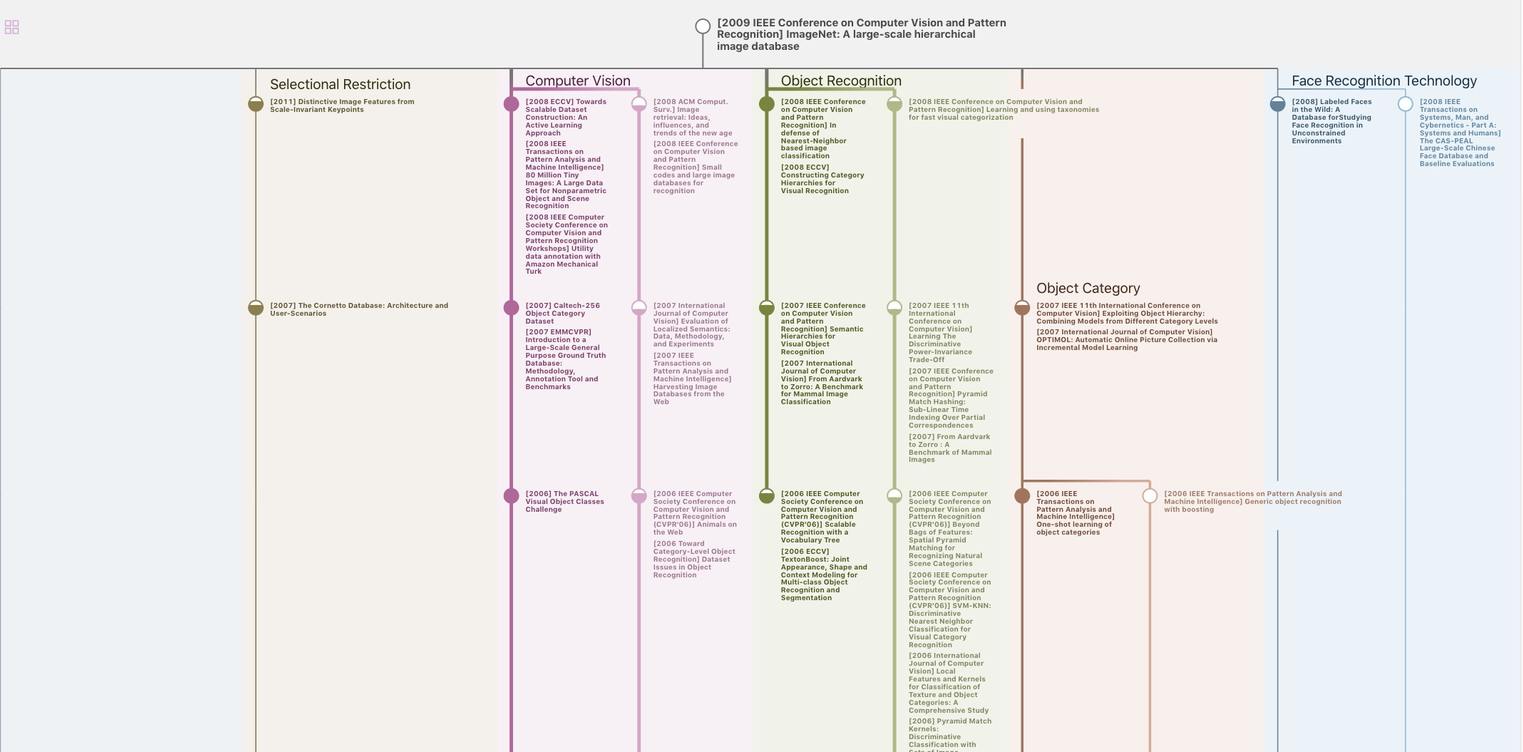
生成溯源树,研究论文发展脉络
Chat Paper
正在生成论文摘要