Adaptive Joint Optimization for 3D Reconstruction With Differentiable Rendering
IEEE transactions on visualization and computer graphics(2023)
摘要
Due to inevitable noises introduced during scanning and quantization, 3D reconstruction via RGB-D sensors suffers from errors both in geometry and texture, leading to artifacts such as camera drifting, mesh distortion, texture ghosting, and blurriness. Given an imperfect reconstructed 3D model, most previous methods have focused on refining either geometry, texture, or camera pose. Consequently, different optimization schemes and objectives for optimizing each component have been used in previous joint optimization methods, forming a complicated system. In this paper, we propose a novel optimization approach based on differentiable rendering, which integrates the optimization of camera pose, geometry, and texture into a unified framework by enforcing consistency between the rendered results and the corresponding RGB-D inputs. Based on the unified framework, we introduce a joint optimization approach to fully exploit the inter-relationships among the three objective components, and describe an adaptive interleaving strategy to improve optimization stability and efficiency. Using differentiable rendering, an image-level adversarial loss is applied to further improve the 3D model, making it more photorealistic. Experiments on synthetic and real data using quantitative and qualitative evaluation demonstrated the superiority of our approach in recovering both fine-scale geometry and high-fidelity texture.
更多查看译文
关键词
Texture optimization,geometry refinement,3D reconstruction,adaptive interleaving strategy,differentiable rendering
AI 理解论文
溯源树
样例
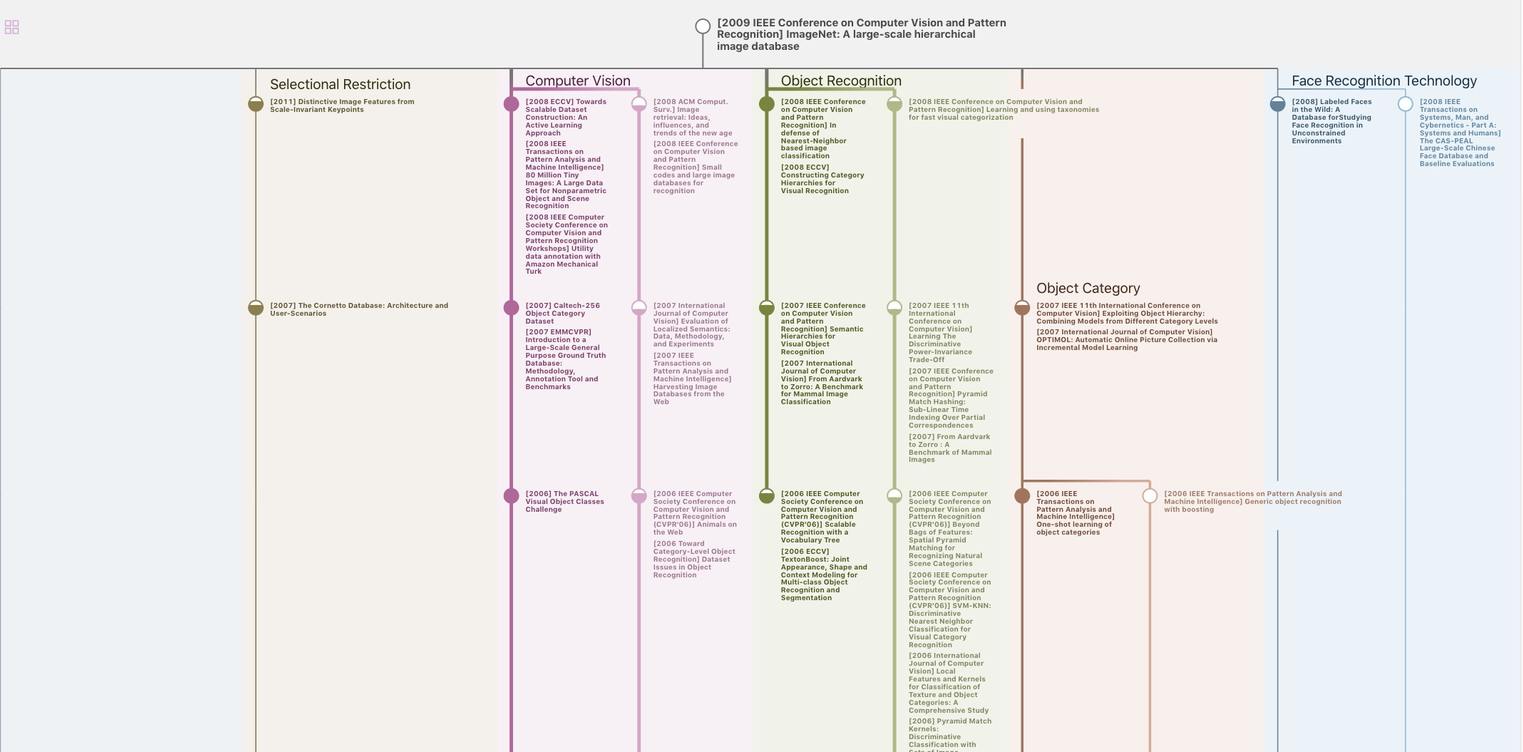
生成溯源树,研究论文发展脉络
Chat Paper
正在生成论文摘要