Causal Inference Through the Structural Causal Marginal Problem
INTERNATIONAL CONFERENCE ON MACHINE LEARNING, VOL 162(2022)
Abstract
We introduce an approach to counterfactual inference based on merging information from multiple datasets. We consider a causal reformulation of the statistical marginal problem: given a collection of marginal structural causal models (SCMs) over distinct but overlapping sets of variables, determine the set of joint SCMs that are counterfactually consistent with the marginal ones. We formalise this approach for categorical SCMs using the response function formulation and show that it reduces the space of allowed marginal and joint SCMs. Our work thus highlights a new mode of falsifiability through additional variables, in contrast to the statistical one via additional data.
MoreTranslated text
AI Read Science
Must-Reading Tree
Example
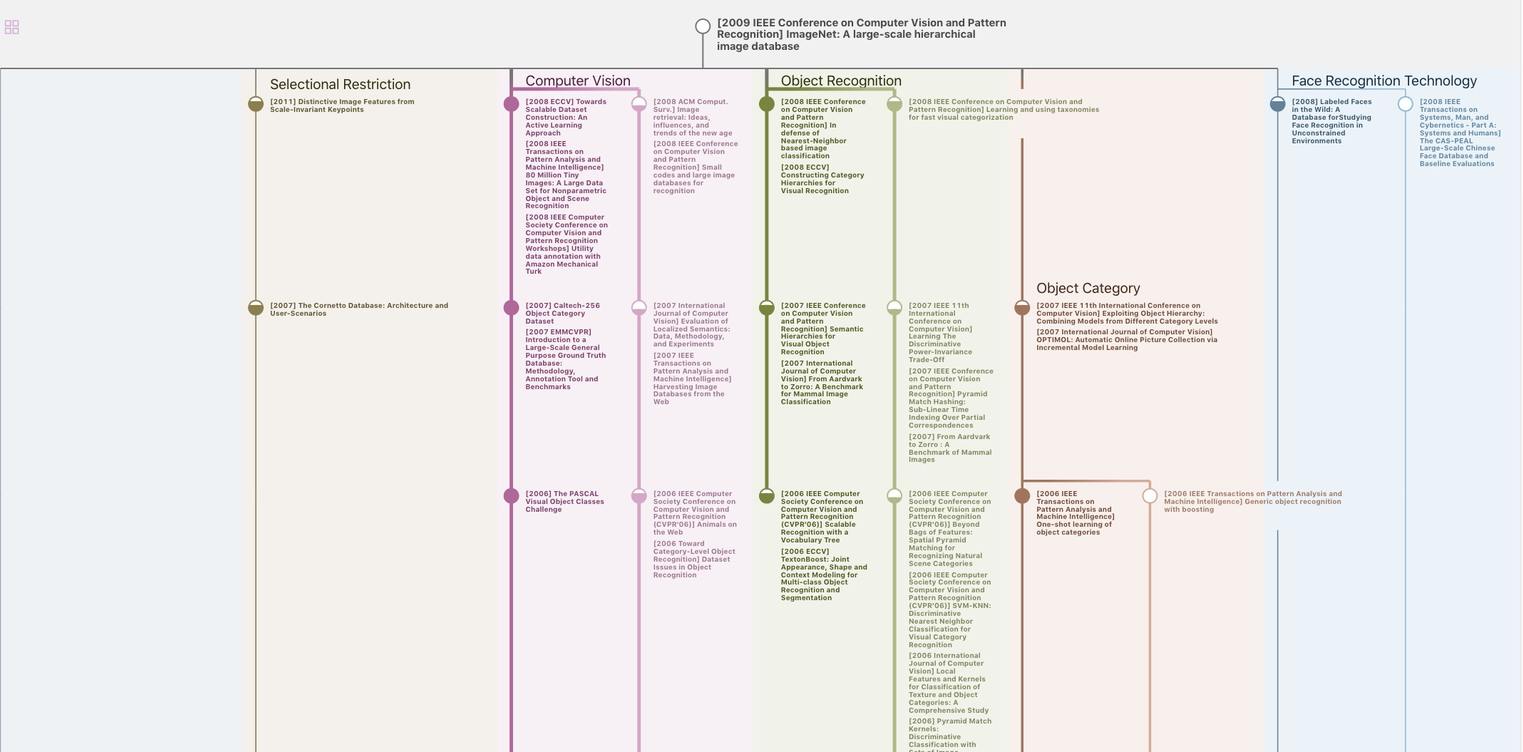
Generate MRT to find the research sequence of this paper
Chat Paper
Summary is being generated by the instructions you defined