Gradient-guided Unsupervised Text Style Transfer via Contrastive Learning
arxiv(2022)
摘要
Text style transfer is a challenging text generation problem, which aims at altering the style of a given sentence to a target one while keeping its content unchanged. Since there is a natural scarcity of parallel datasets, recent works mainly focus on solving the problem in an unsupervised manner. However, previous gradient-based works generally suffer from the deficiencies as follows, namely: (1) Content migration. Previous approaches lack explicit modeling of content invariance and are thus susceptible to content shift between the original sentence and the transferred one. (2) Style misclassification. A natural drawback of the gradient-guided approaches is that the inference process is homogeneous with a line of adversarial attack, making latent optimization easily becomes an attack to the classifier due to misclassification. This leads to difficulties in achieving high transfer accuracy. To address the problems, we propose a novel gradient-guided model through a contrastive paradigm for text style transfer, to explicitly gather similar semantic sentences, and to design a siamese-structure based style classifier for alleviating such two issues, respectively. Experiments on two datasets show the effectiveness of our proposed approach, as compared to the state-of-the-arts.
更多查看译文
关键词
unsupervised text style transfer,contrastive
AI 理解论文
溯源树
样例
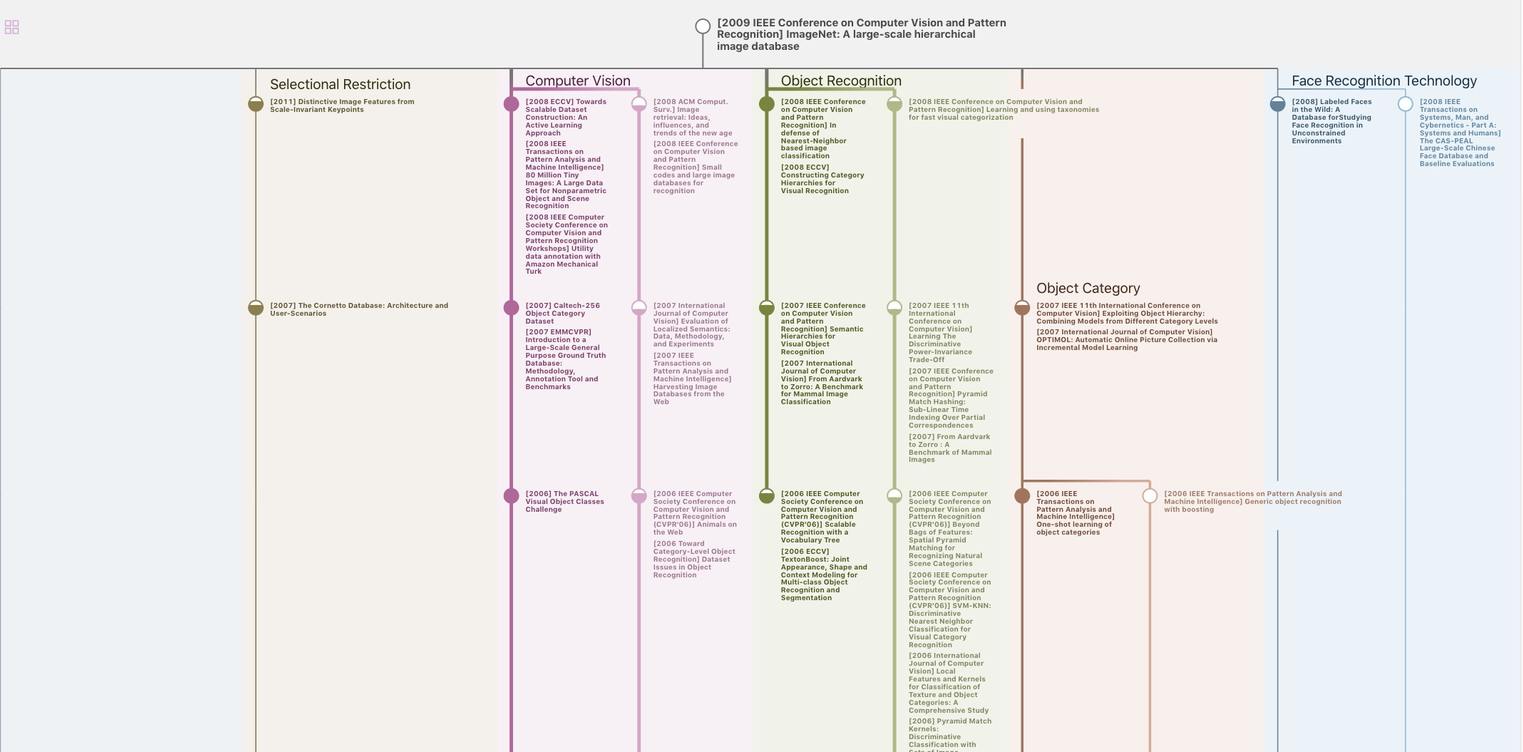
生成溯源树,研究论文发展脉络
Chat Paper
正在生成论文摘要