Improving Robustness and Calibration in Ensembles with Diversity Regularization
PATTERN RECOGNITION, DAGM GCPR 2022(2022)
摘要
Calibration and uncertainty estimation are crucial topics in high-risk environments. Following the recent interest in the diversity of ensembles, we systematically evaluate the viability of explicitly regularizing ensemble diversity to improve robustness and calibration on in-distribution data as well as under dataset shift. We introduce a new diversity regularizer for classification tasks that uses out-of-distribution samples and increases the overall accuracy, calibration and out-of-distribution detection capabilities of ensembles. We demonstrate that diversity regularization is highly beneficial in architectures where weights are partially shared between the individual members and even allows to use fewer ensemble members to reach the same level of robustness. Experiments on CIFAR-10, CIFAR-100, and SVHN show that regularizing diversity can have a significant impact on calibration and robustness, as well as out-of-distribution detection.
更多查看译文
关键词
Diversity, Ensembles, Robustness, Calibration
AI 理解论文
溯源树
样例
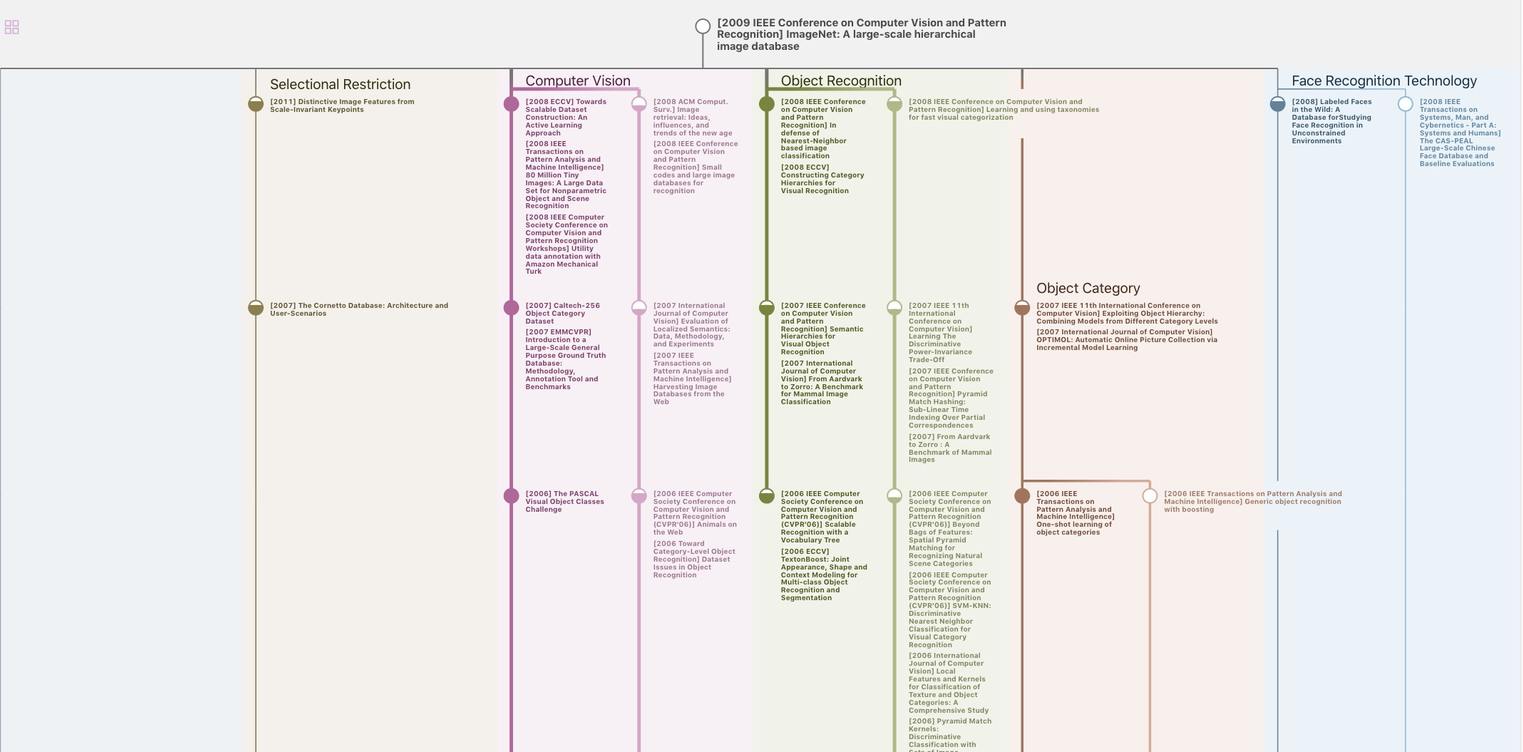
生成溯源树,研究论文发展脉络
Chat Paper
正在生成论文摘要