Is young-onset esophageal adenocarcinoma increasing in Japan? An analysis of population-based cancer registries
CANCER MEDICINE(2022)
摘要
Background While esophageal squamous cell carcinoma (ESCC) is the predominant histological type in Japan, concern has been expressed over an increase in the proportion of esophageal adenocarcinoma (EAC), especially in middle-aged populations. This study aimed to assess long-term trends in esophageal cancer incidence by histological type. Methods We used data from three population-based cancer registries in Japan with 10,642 esophageal cancer cases diagnosed between 1993 and 2014. The multiple imputation approach was used to impute a specific histological type (ESCC, EAC, and others) for cases with "Unknown" or missing status. We calculated the age-standardized incidence rates by histological type from 1993 to 2014 and fitted age-period-cohort models to estimate the annual percent changes (APCs) and adjusted incidence rate ratios (IRRs). Results After imputation of missing data, the largest mean APC increase was seen in the incidence of EAC in men aged 40-49 years (7.1%) followed by those aged 50-59 years (5.5%). The age-period-cohort analysis showed that men who were born in the 1960s and later were more likely to develop EAC relative to men who were born in 1950-1959 (1960-1969 cohort, IRR: 1.42; 1970-1974 cohort, IRR: 2.23), with a period effect indicating a constant increase after 2003. For women, no significant trend in EAC incidence was observed. Conclusions The incidence of EAC has increased more prominently compared with that of ESCC, especially in men aged 40-59 years, suggesting the impact of increasing obesity in men and a reduction in H. pylori prevalence in Japan.
更多查看译文
关键词
age-period-cohort model, cancer registry, esophageal cancer, incidence, missing data, multiple imputation, neoplasms
AI 理解论文
溯源树
样例
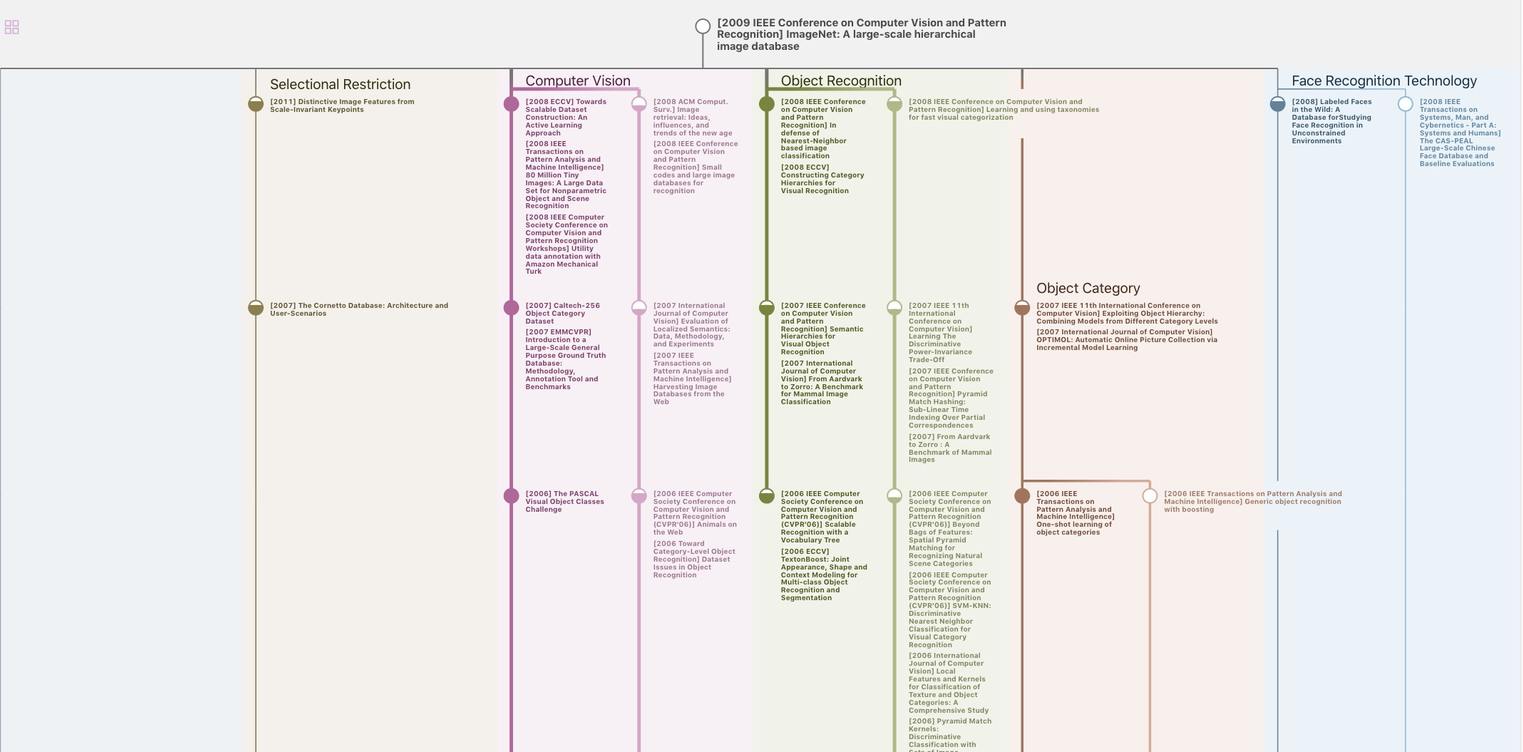
生成溯源树,研究论文发展脉络
Chat Paper
正在生成论文摘要