Transfer Learning for Convolutional Indoor Positioning Systems
INTERNATIONAL CONFERENCE ON INDOOR POSITIONING AND INDOOR NAVIGATION (IPIN 2021)(2021)
摘要
Fingerprinting is a widely used technique in indoor positioning, mainly due to its simplicity. Usually, this technique is used with the deterministic k - Nearest Neighbors (k-NN) algorithm. Utilizing a neural network model for fingerprinting positioning purposes can greatly improve the prediction speed compared to the k-NN approach, but requires a voluminous training dataset to achieve comparable performance. In many indoor positioning datasets, the number of samples is only at a level of hundreds, which results in poor performance of the neural network solution. In this work, we develop a novel algorithm based on a transfer learning approach, which combines samples from 15 different Wi-Fi RSS indoor positioning datasets, to train a single convolutional neural network model, which learns the common patterns in the combined data. The proposed model is then fine-tuned to optimally fit the individual databases. We show that the proposed solution reduces the positioning error by up to 25% compared to the benchmark model while reducing the number of outlier predictions.
更多查看译文
关键词
artificial neural network,convolutional neural network,deep learning,fingerprinting,indoor positioning,machine learning,transfer learning,WLAN
AI 理解论文
溯源树
样例
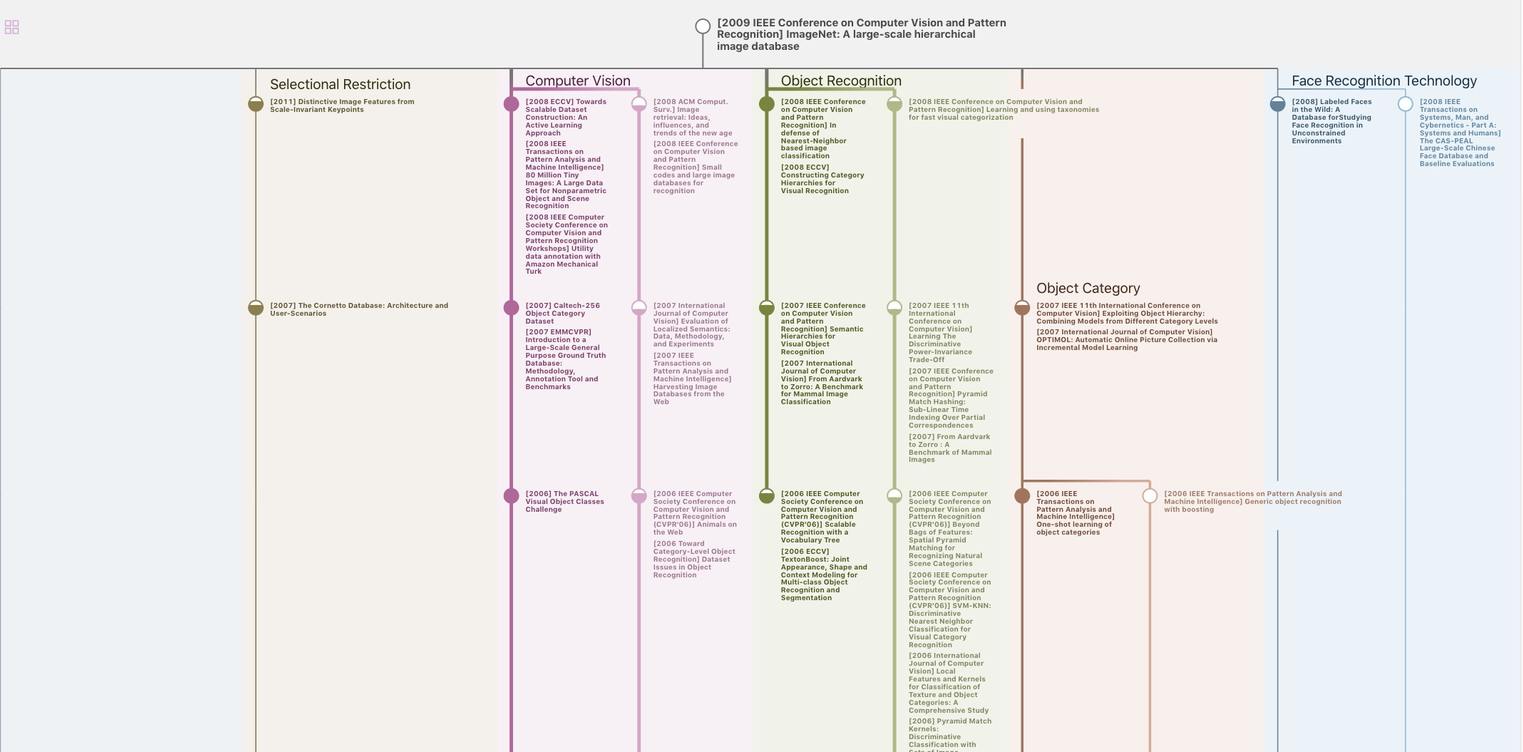
生成溯源树,研究论文发展脉络
Chat Paper
正在生成论文摘要