Robust and Task-Aware Training of Deep Residual Networks for Varying-Lead ECG Classification
CinC(2021)
摘要
In PhysioNet/Computing in Cardiology Challenge 2021, we developed an ensemble model by combining different epochs of ResNet to classify cardiac abnormalities from 12, 6, 4, 3, 2 lead electrocardiogram (ECG) signals, where epochs are chosen based on validation performance on China Physiological Signal Challenge (CPSC) dataset and Georgia dataset. In order to adapt to the specially designed Challenge score, we designed a multi-task loss to combine the benefit of binary cross-entropy loss and Challenge loss. Besides, we also integrated a subsample frequency feature into the model to learn from the signals. To gain a better generalization ability, mixup and weighted loss are introduced. We submitted our model in the official phase with team name DataLA NUS, and our final selected model achieved a Challenge score of 0.51, 0.51, 0.51, 0.50, 0.52 (ranked 8th, 5th, 6th, 8th, 5th) on the 12-lead, 6-lead, 4-lead, 3-lead, and 2-lead setting on the final hidden test set with the Challenge evaluation metric.
更多查看译文
关键词
deep residual networks,ecg,training,task-aware,varying-lead
AI 理解论文
溯源树
样例
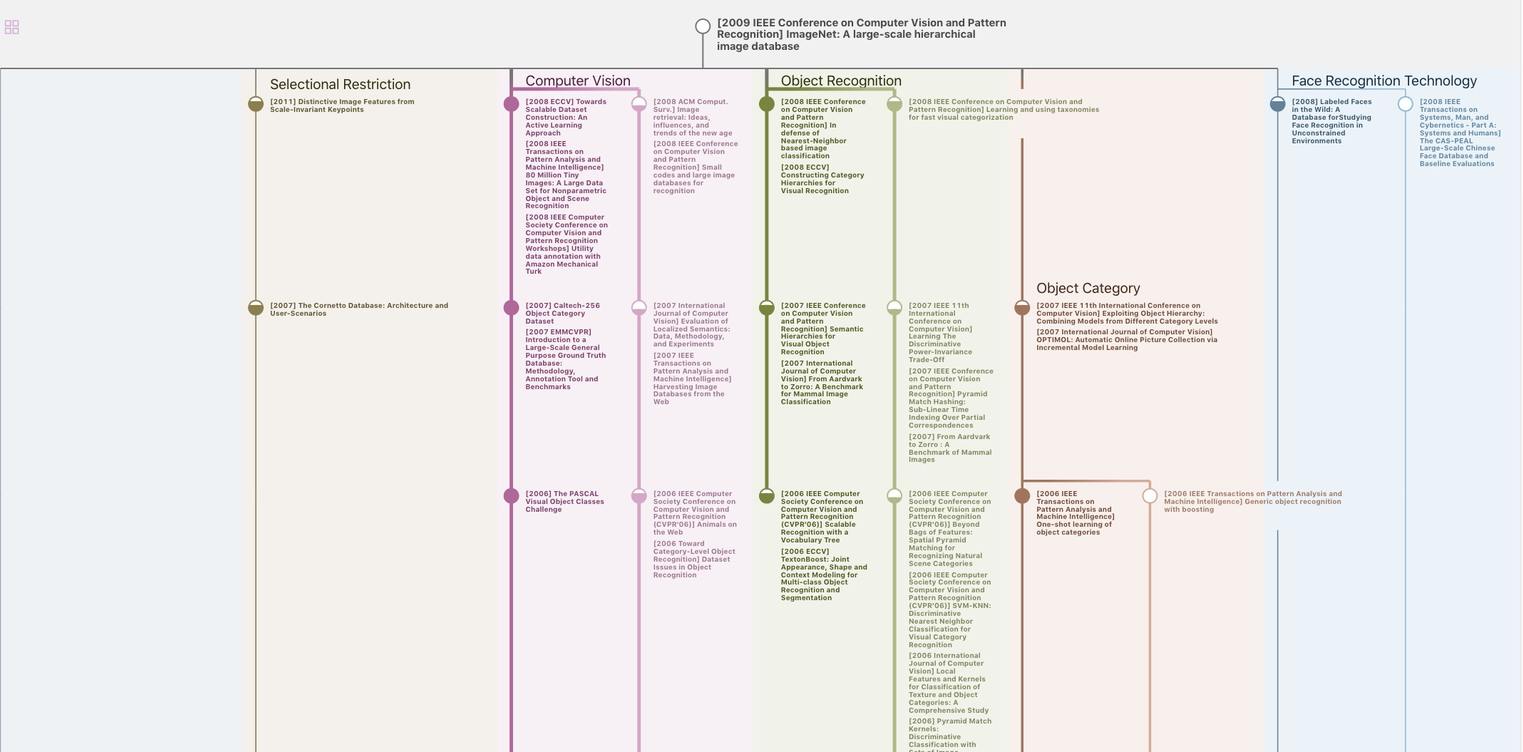
生成溯源树,研究论文发展脉络
Chat Paper
正在生成论文摘要