Adversarial Classification under Gaussian Mechanism: Calibrating the Attack to Sensitivity
CoRR(2022)
摘要
This work studies anomaly detection under differential privacy with Gaussian perturbation using both statistical and information-theoretic tools. In our setting, the adversary aims to modify the differentially private information of a statistical dataset by inserting additional data without being detected by using the differential privacy to her/his own benefit. To this end, firstly via hypothesis testing, we characterize a statistical threshold for the adversary, which balances the privacy budget and the induced bias (the impact of the attack) in order to remain undetected. In addition, we establish the privacy-distortion tradeoff in the sense of the well-known rate-distortion function for the Gaussian mechanism by using an information-theoretic approach and present an upper bound on the variance of the attacker's additional data as a function of the sensitivity and the original data's second-order statistics. Lastly, we introduce a new privacy metric based on Chernoff information for classifying adversaries under differential privacy as a stronger alternative for the Gaussian mechanism. Analytical results are supported by numerical evaluations.
更多查看译文
关键词
adversarial classification,gaussian mechanism,sensitivity,attack
AI 理解论文
溯源树
样例
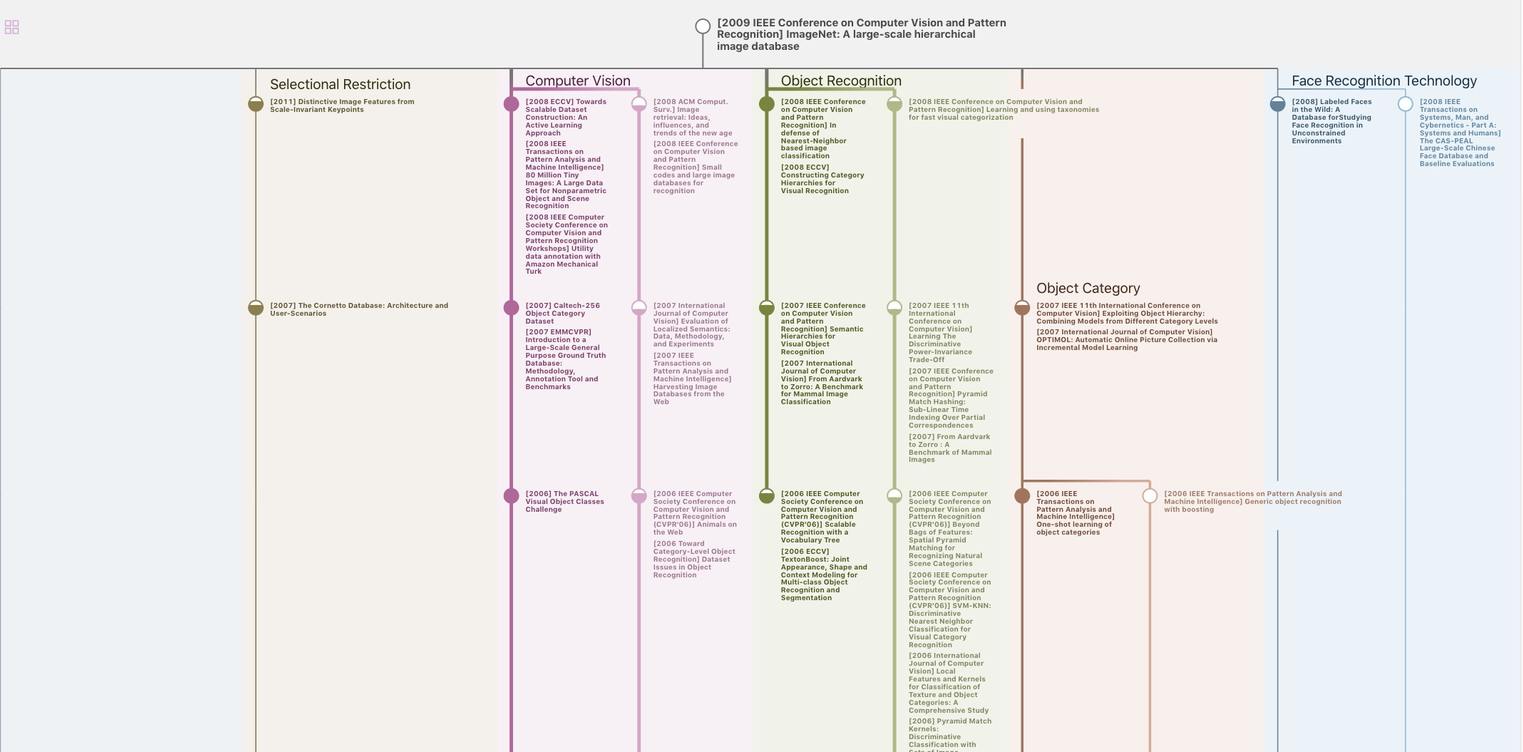
生成溯源树,研究论文发展脉络
Chat Paper
正在生成论文摘要