FAME: Fragment-based Conditional Molecular Generation for Phenotypic Drug Discovery
bioRxiv (Cold Spring Harbor Laboratory)(2022)
摘要
De novo molecular design is a key challenge in drug discovery due to the complexity of chemical space. With the availability of molecular datasets and advances in machine learning, many deep generative models are proposed for generating novel molecules with desired properties. However, most of the existing models focus only on molecular distribution learning and target-based molecular design, thereby hindering their potentials in real-world applications. In drug discovery, phenotypic molecular design has advantages over target-based molecular design, especially in first-in-class drug discovery. In this work, we propose the first deep graph generative model (FAME) targeting phenotypic molecular design, in particular gene expression-based molecular design. FAME leverages a conditional variational autoencoder framework to learn the conditional distribution generating molecules from gene expression profiles. However, this distribution is difficult to learn due to the complexity of the molecular space and the noisy phenomenon in gene expression data. To tackle these issues, a gene expression denoising (GED) model that employs contrastive objective function is first proposed to reduce noise from gene expression data. FAME is then designed to treat molecules as the sequences of fragments and learn to generate these fragments in autoregressive manner. By leveraging this fragment-based generation strategy and the denoised gene expression profiles, FAME can generate novel molecules with a high validity rate and desired biological activity. The experimental results show that FAME outperforms existing methods including both SMILES-based and graph-based deep generative models for phenotypic molecular design. Furthermore, the effective mechanism for reducing noise in gene expression data proposed in our study can be applied to omics data modeling in general for facilitating phenotypic drug discovery.### Competing Interest StatementThe authors have declared no competing interest.
更多查看译文
关键词
Homology Modeling,Materials Discovery,Drug Target Identification,Computational Chemistry,Molecular Docking
AI 理解论文
溯源树
样例
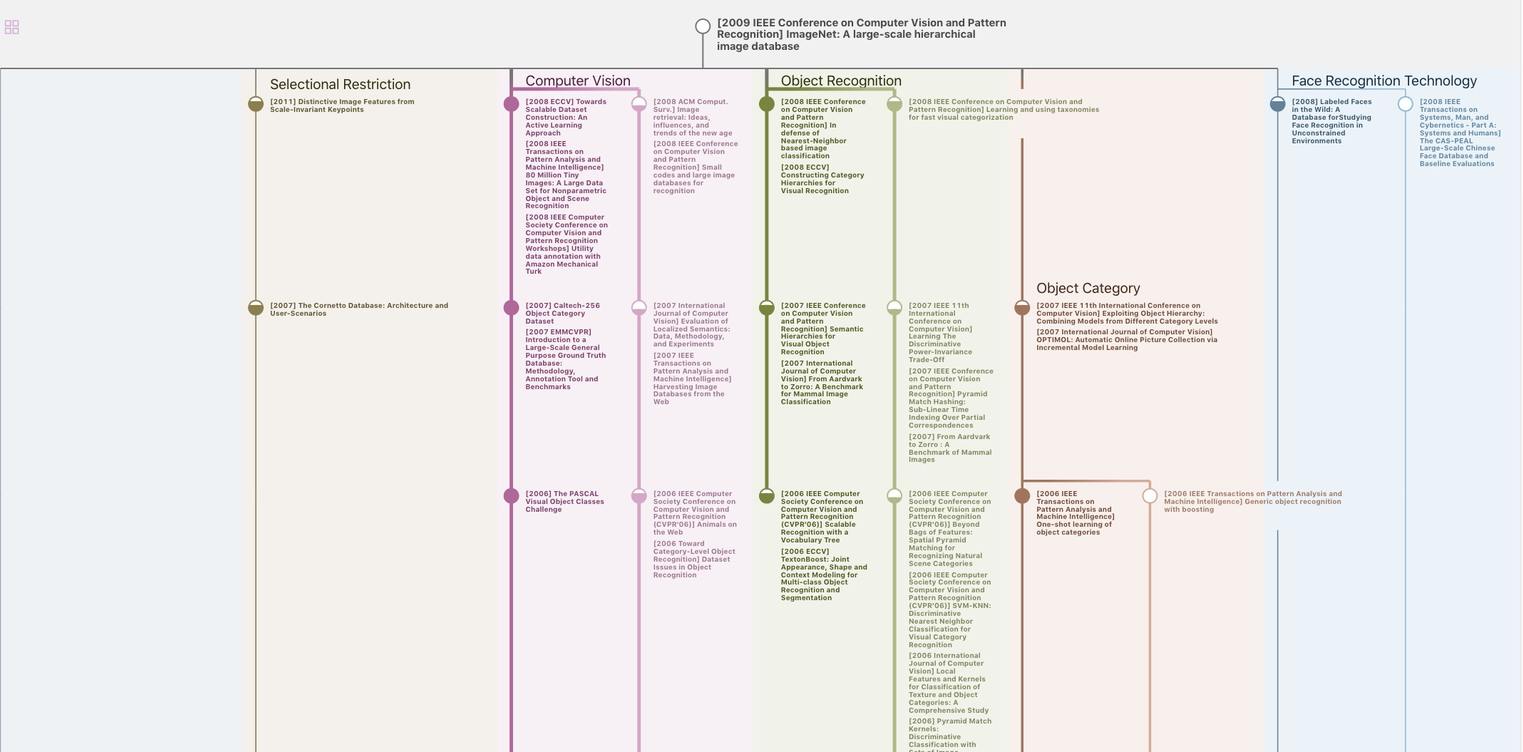
生成溯源树,研究论文发展脉络
Chat Paper
正在生成论文摘要