Distance-Ratio-Based Formulation for Metric Learning
CoRR(2022)
摘要
In metric learning, the goal is to learn an embedding so that data points with the same class are close to each other and data points with different classes are far apart. We propose a distance-ratio-based (DR) formulation for metric learning. Like softmax-based formulation for metric learning, it models $p(y=c|x')$, which is a probability that a query point $x'$ belongs to a class $c$. The DR formulation has two useful properties. First, the corresponding loss is not affected by scale changes of an embedding. Second, it outputs the optimal (maximum or minimum) classification confidence scores on representing points for classes. To demonstrate the effectiveness of our formulation, we conduct few-shot classification experiments using softmax-based and DR formulations on CUB and mini-ImageNet datasets. The results show that DR formulation generally enables faster and more stable metric learning than the softmax-based formulation. As a result, using DR formulation achieves improved or comparable generalization performances.
更多查看译文
关键词
learning,formulation,distance-ratio-based
AI 理解论文
溯源树
样例
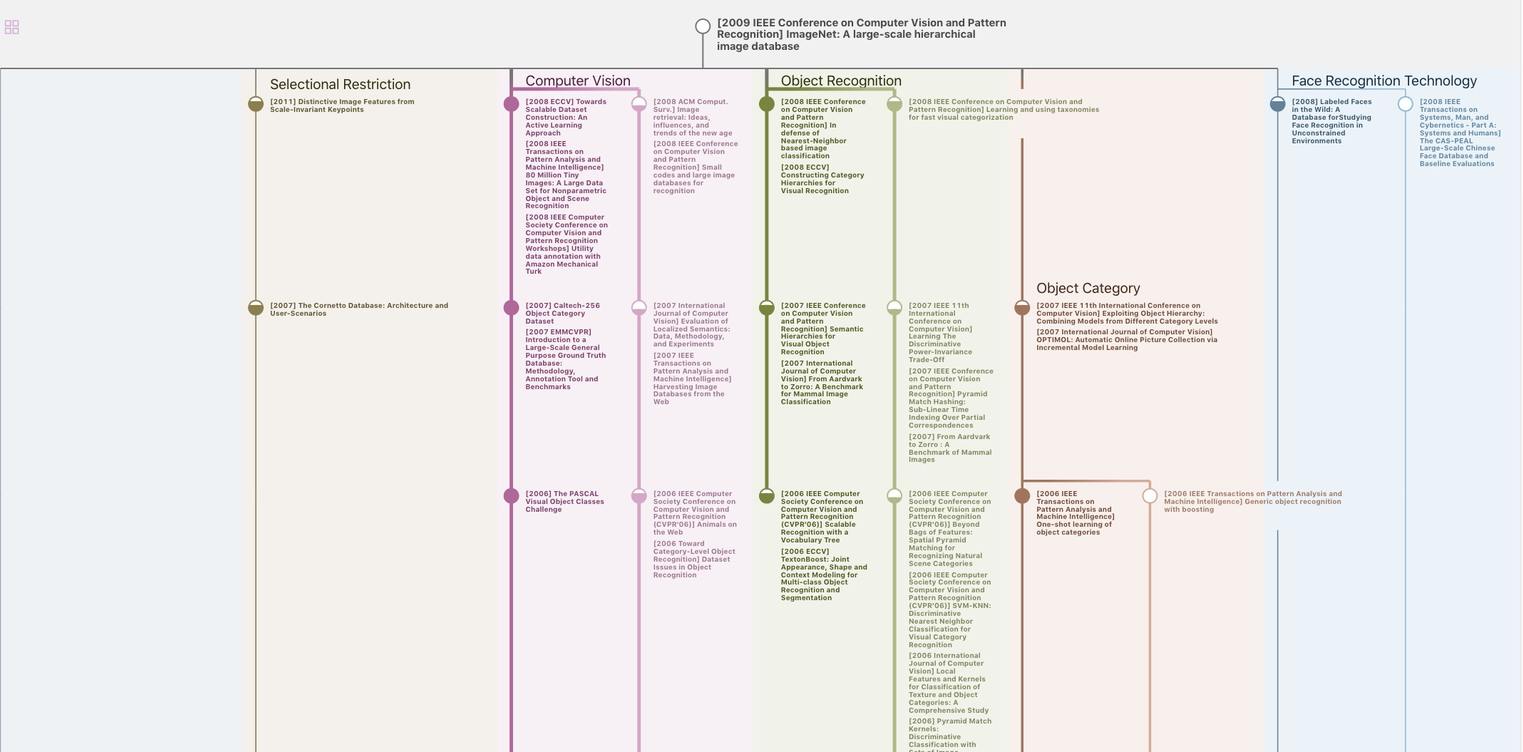
生成溯源树,研究论文发展脉络
Chat Paper
正在生成论文摘要