High Accuracy Real-Time Multi-Gas Identification by a Batch-Uniform Gas Sensor Array and Deep Learning Algorithm.
ACS sensors(2022)
摘要
Semiconductor metal oxide (SMO) gas sensors are attracting great attention as next-generation environmental monitoring sensors. However, there are limitations to the actual application of SMO gas sensors due to their low selectivity. Although the electronic nose (E-nose) systems based on a sensor array are regarded as a solution for the selectivity issue, poor accuracy caused by the nonuniformity of the fabricated gas sensors and difficulty of real-time gas detection have yet to be resolved. In this study, these problems have been solved by fabricating uniform gas sensor arrays and applying the deep learning algorithm to the data from the sensor arrays. Nanocolumnar films of metal oxides (SnO2, In2O3, WO3, and CuO) with a high batch uniformity deposited through glancing angle deposition were used as the sensing materials. The convolutional neural network (CNN) using the input data as a matrix form was adopted as a learning algorithm, which could conduct pattern recognition of the sensor responses. Finally, real-time selective gas detection for CO, NH3, NO2, CH4, and acetone (C3H6O) gas was achieved (minimum response time of 1, 8, 5, 19, and 2 s, respectively) with an accuracy of 98% by applying preprocessed response data to the CNN.
更多查看译文
关键词
gas sensor array,electronic nose,glancing angle deposition,deep learning,real-time gas identification
AI 理解论文
溯源树
样例
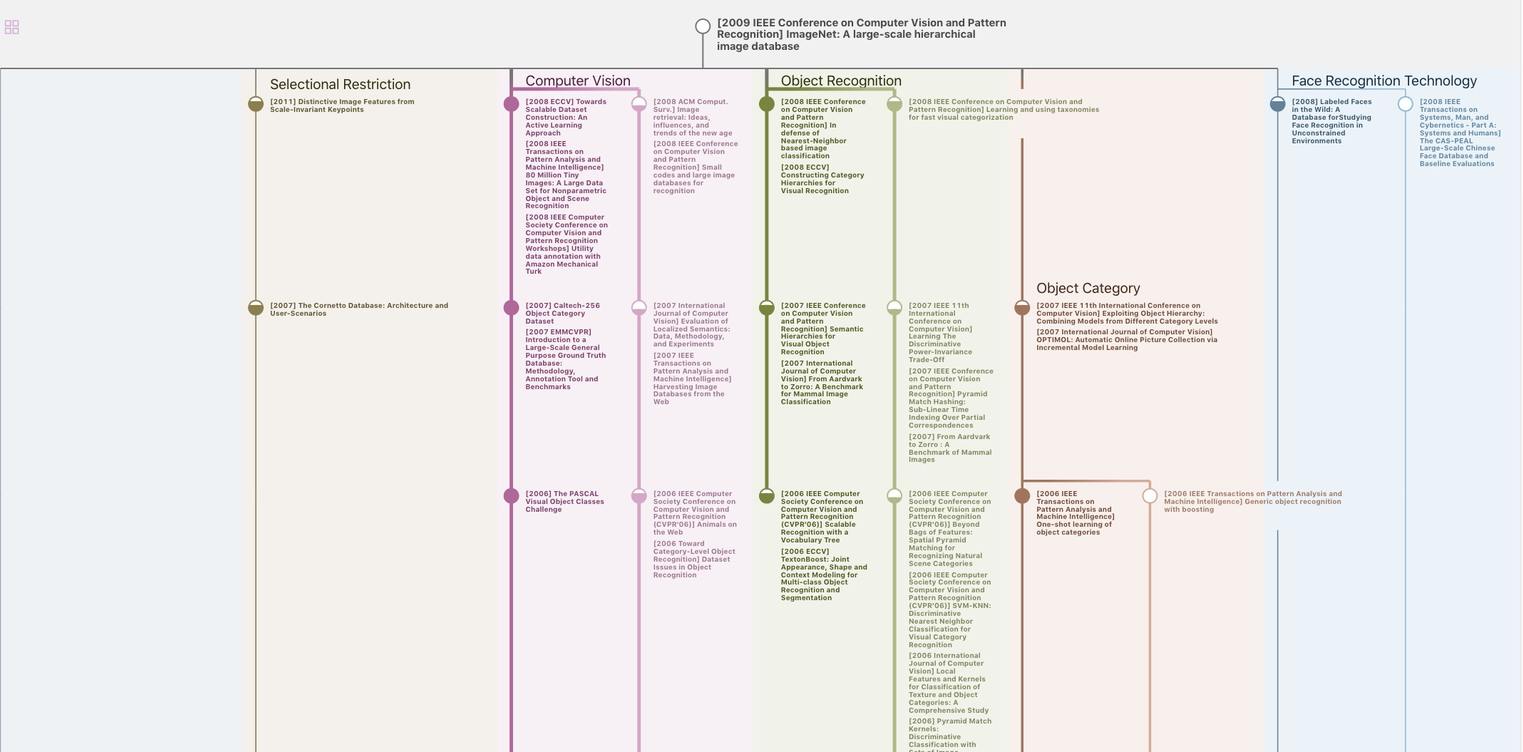
生成溯源树,研究论文发展脉络
Chat Paper
正在生成论文摘要