Model-driven Cluster Resource Management for AI Workloads in Edge Clouds
arxiv(2022)
摘要
Since emerging edge applications such as Internet of Things (IoT) analytics and augmented reality have tight latency constraints, hardware AI accelerators have been recently proposed to speed up deep neural network (DNN) inference run by these applications. Resource-constrained edge servers and accelerators tend to be multiplexed across multiple IoT applications, introducing the potential for performance interference between latency-sensitive workloads. In this paper, we design analytic models to capture the performance of DNN inference workloads on shared edge accelerators, such as GPU and edgeTPU, under different multiplexing and concurrency behaviors. After validating our models using extensive experiments, we use them to design various cluster resource management algorithms to intelligently manage multiple applications on edge accelerators while respecting their latency constraints. We implement a prototype of our system in Kubernetes and show that our system can host 2.3X more DNN applications in heterogeneous multi-tenant edge clusters with no latency violations when compared to traditional knapsack hosting algorithms.
更多查看译文
关键词
Cloud computing,edge computing,resource management,analytics modeling,ML inference
AI 理解论文
溯源树
样例
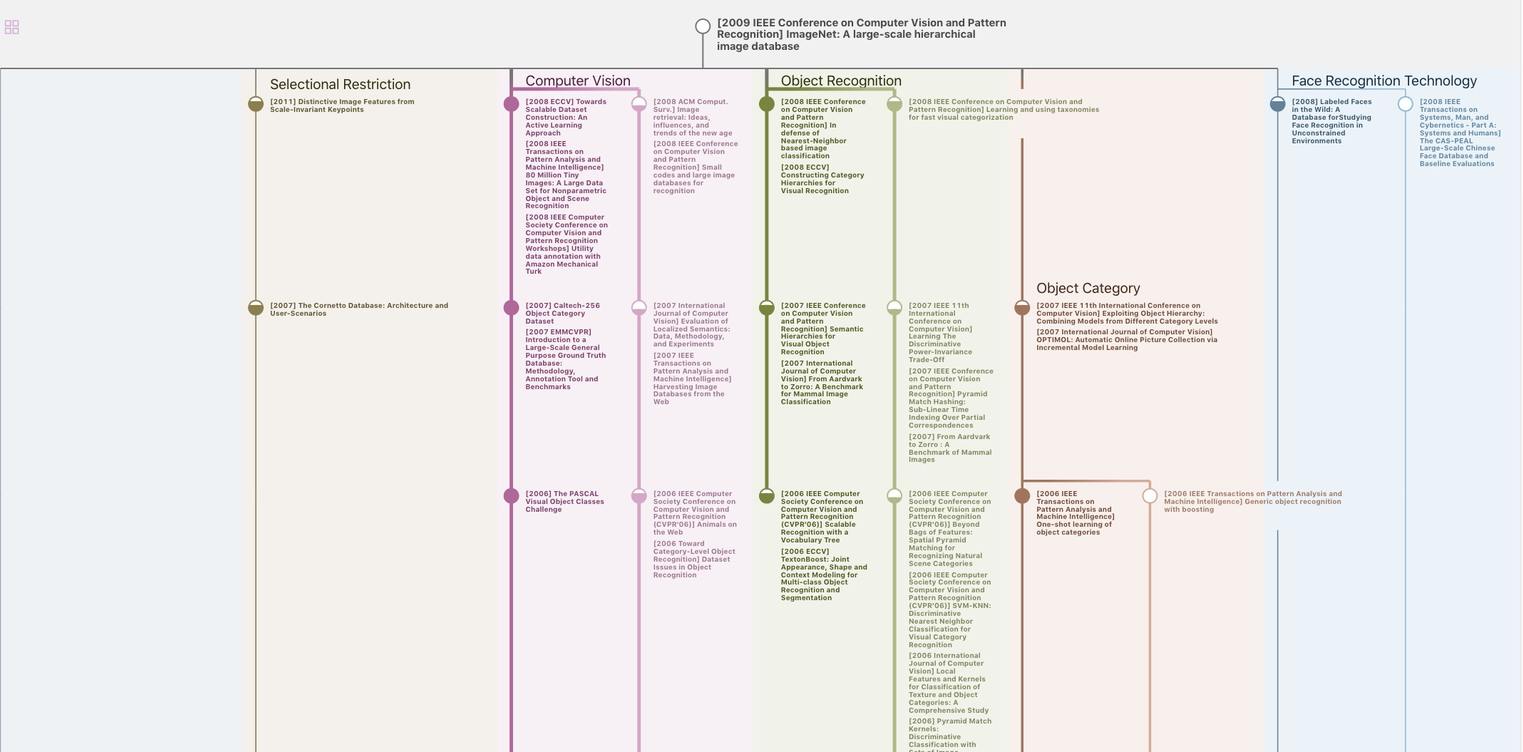
生成溯源树,研究论文发展脉络
Chat Paper
正在生成论文摘要