Multiplayer Performative Prediction: Learning in Decision-Dependent Games
JOURNAL OF MACHINE LEARNING RESEARCH(2023)
摘要
Learning problems commonly exhibit an interesting feedback mechanism wherein the population data reacts to competing decision makers' actions. This paper formulates a new game theoretic framework for this phenomenon, called multi-player performative prediction. We focus on two distinct solution concepts, namely (i) performatively stable equilibria and (ii) Nash equilibria of the game. The latter equilibria are arguably more informative, but are generally computationally difficult to find since they are solutions of nonmonotone games. We show that under mild assumptions, the performatively stable equilibria can be found efficiently by a variety of algorithms, including repeated retraining and the repeated (stochastic) gradient method. We then establish transparent sufficient conditions for strong monotonicity of the game and use them to develop algorithms for finding Nash equilibria. We investigate derivative free methods and adaptive gradient algorithms wherein each player alternates between learning a parametric description of their distribution and gradient steps on the empirical risk. Synthetic and semi-synthetic numerical experiments illustrate the results.
更多查看译文
关键词
performative prediction,stochastic games,stochastic optimization,distribu-tional shift,stochastic gradient method
AI 理解论文
溯源树
样例
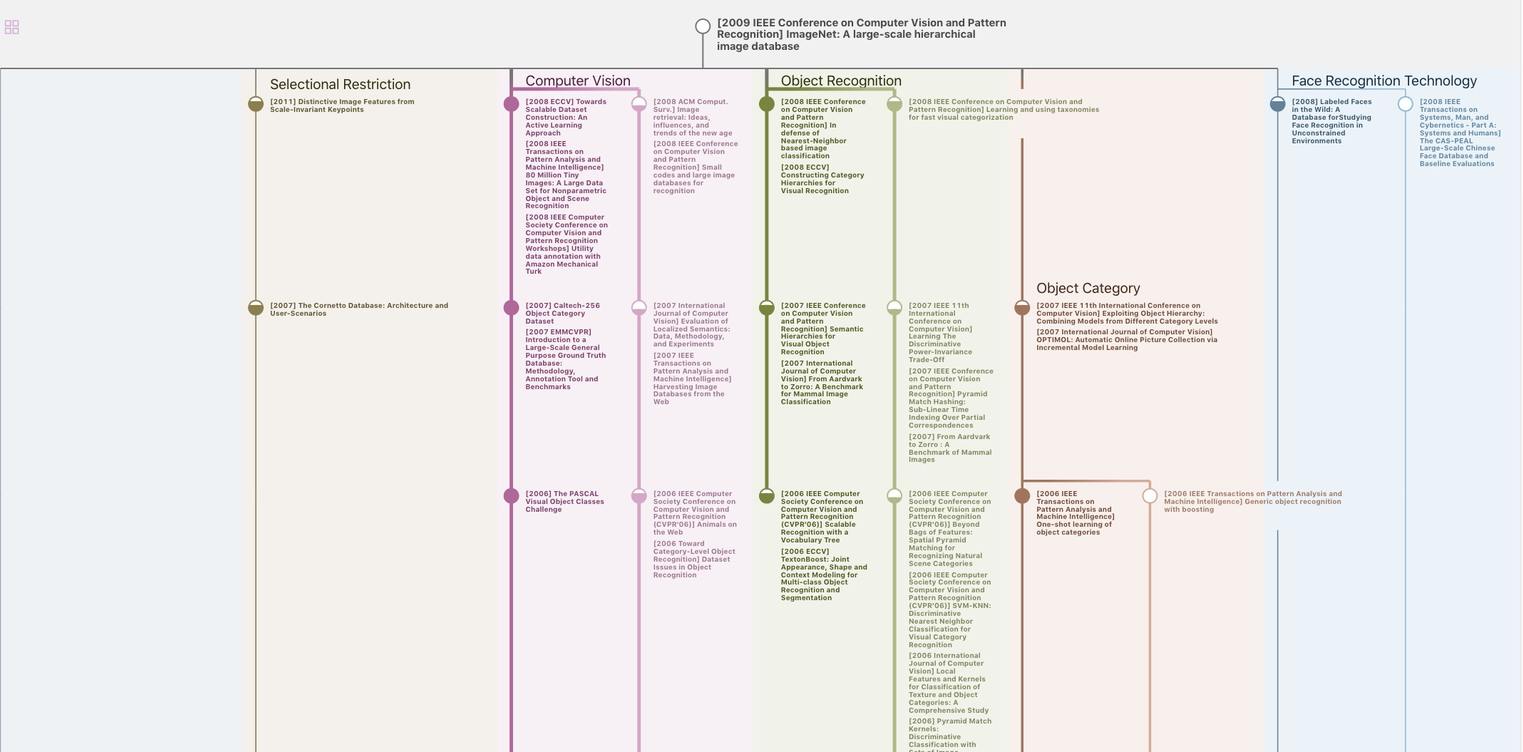
生成溯源树,研究论文发展脉络
Chat Paper
正在生成论文摘要