Analysis of Neural Network Predictions for Entanglement Self-Catalysis
Brazilian Journal of Physics(2022)
摘要
Machine learning techniques have been successfully applied to classifying an extensive range of phenomena in quantum theory. From detecting quantum phase transitions to identifying Bell non-locality, it has been established that classical machines can learn genuine quantum features via classical data. Quantum entanglement is one of the uniquely quantum phenomena in that range, as it has been shown that neural networks can be used to classify different types of entanglement. Our work builds on this topic. We investigate whether distinct models of neural networks can learn how to detect catalysis and self-catalysis of entanglement for pure states. Additionally, we also study whether a trained machine can detect another related phenomenon - which we dub transfer knowledge. As we build our models from scratch, besides making all the codes available, we can study a whole gamut of paradigmatic measures, including accuracy, execution time, training time, bias in the training data set and so on.
更多查看译文
关键词
Entanglement transformations,Supervised learning,Catalysis,Machine learning applications
AI 理解论文
溯源树
样例
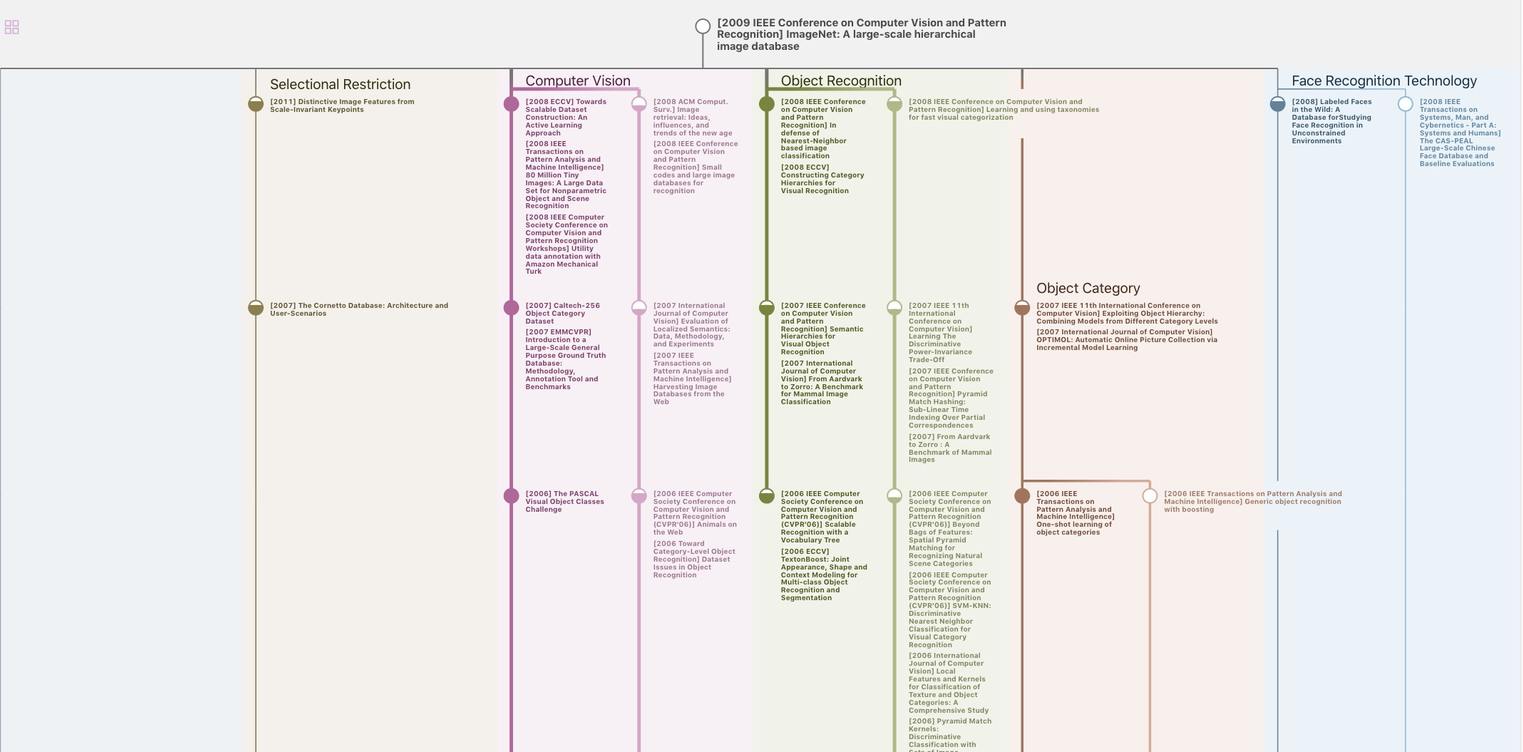
生成溯源树,研究论文发展脉络
Chat Paper
正在生成论文摘要