亚热带典型人工林凋落物地表和空中分解过程中溶解性有机质数量和光谱特征
Chinese Journal of Ecology(2021)
Abstract
以江西亚热带地区主要造林树种枫香(Liquidambar formosana)、木荷(Schima su-perba)、马尾松(Pinus massoniana)和湿地松(Pinus elliottii)作为研究对象,分别选取空中和地表分解150和360 d的叶片凋落物,通过模拟淋溶试验探究分解位置对凋落物来源的溶解性有机质(DOM)数量、C∶N∶P化学计量比和紫外-可见吸收光谱特征的影响.结果表明:(1)分解150 d后,除木荷外,地表分解的枫香、马尾松和湿地松凋落物溶解性有机碳(DOC)产量显著高于空中分解的凋落物,但分解360 d后,分解位置对凋落物源DOC产量的影响因树种而异;(2)所有树种空中分解150 d的凋落物浸提液中C∶N、C∶P和N∶P比均高于地表分解的凋落物,但在分解360 d后,分解位置对C∶N∶P化学计量比的影响随着凋落物类型的变化而变化;(3)分解150 d后,与地表分解的凋落物相比,空中分解的凋落物淋溶液中的SUVA254、SUVA280和SUVA350值较低,而S275-295和S350-400值较高,即DOM芳香化程度和分子质量较低;分解360 d后,阔叶树种(枫香和木荷)维持这种空间变化趋势,而针叶树种(马尾松和湿地松)则呈现相反的格局.综上,分解位置是影响亚热带人工林植物凋落物来源DOM数量和质量的重要因素,但其调控格局受凋落物类型和分解阶段的影响.
More求助PDF
上传PDF
View via Publisher
AI Read Science
AI Summary
AI Summary is the key point extracted automatically understanding the full text of the paper, including the background, methods, results, conclusions, icons and other key content, so that you can get the outline of the paper at a glance.
Example
Background
Key content
Introduction
Methods
Results
Related work
Fund
Key content
- Pretraining has recently greatly promoted the development of natural language processing (NLP)
- We show that M6 outperforms the baselines in multimodal downstream tasks, and the large M6 with 10 parameters can reach a better performance
- We propose a method called M6 that is able to process information of multiple modalities and perform both single-modal and cross-modal understanding and generation
- The model is scaled to large model with 10 billion parameters with sophisticated deployment, and the 10 -parameter M6-large is the largest pretrained model in Chinese
- Experimental results show that our proposed M6 outperforms the baseline in a number of downstream tasks concerning both single modality and multiple modalities We will continue the pretraining of extremely large models by increasing data to explore the limit of its performance
Upload PDF to Generate Summary
Must-Reading Tree
Example
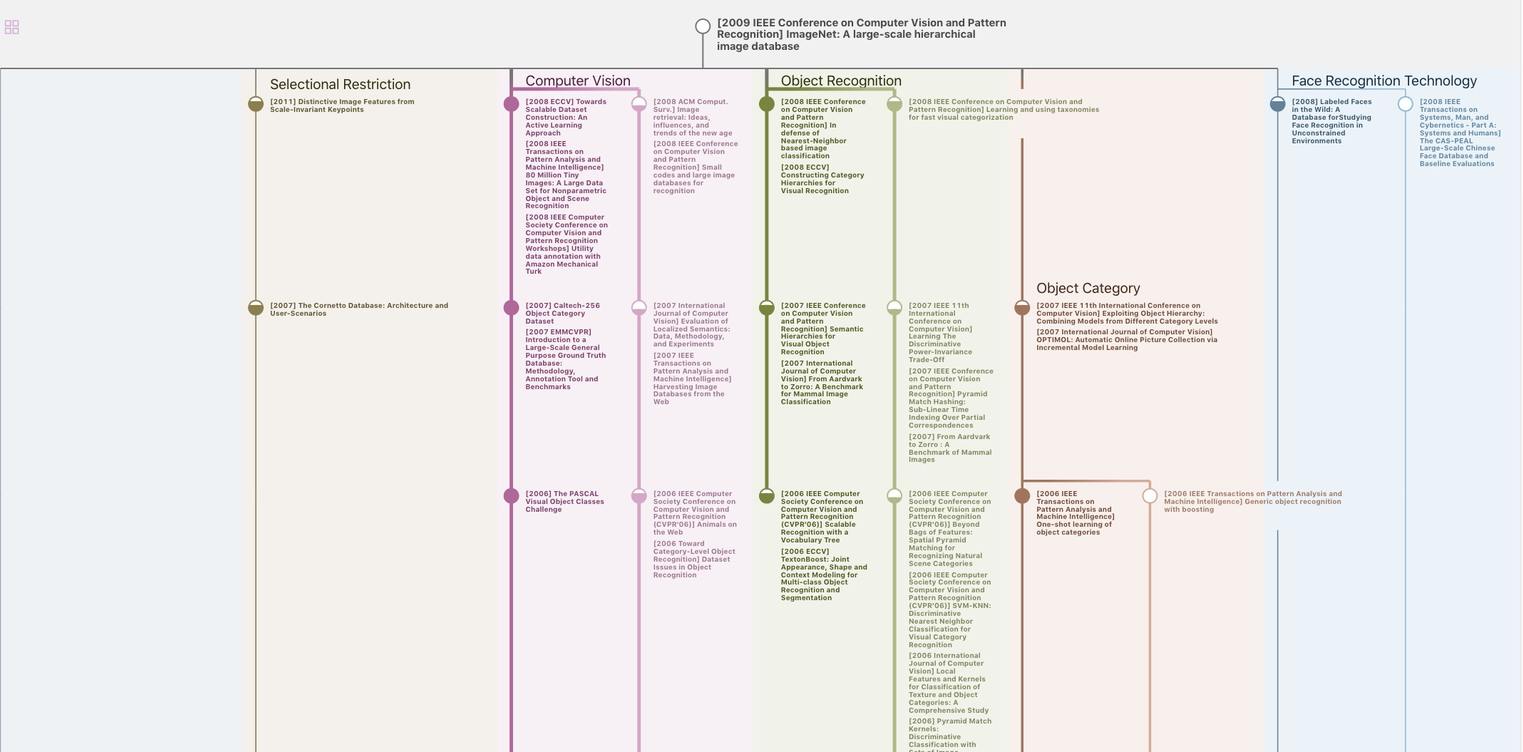
Generate MRT to find the research sequence of this paper
Related Papers
Chinese Journal of Ecology 2022
被引用0
Data Disclaimer
The page data are from open Internet sources, cooperative publishers and automatic analysis results through AI technology. We do not make any commitments and guarantees for the validity, accuracy, correctness, reliability, completeness and timeliness of the page data. If you have any questions, please contact us by email: report@aminer.cn
Chat Paper
GPU is busy, summary generation fails
Rerequest