Fault Prognostics Using LSTM Networks: Application to Marine Diesel Engine
IEEE sensors journal(2021)
摘要
Maintenance is the key to ensuring the safe and efficient operation of marine vessels. Currently, reactive maintenance and preventive maintenance are two main approaches used onboard. These approaches are either cost-intensive or labor-intensive. Recently, Prognostics and Health Management has emerged as an optimal way to manage maintenance operations. In such a system, fault prognostics aims to predict the remaining useful life based on the sensor measurements. In this paper, the feasibility of applying data-driven fault prognostics to marine diesel engines is investigated. Real-world run-to-failure data of two independent fault-types in two different engine load profiles are collected from a hybrid power lab. The first profile is used for training and validation, while the second is used for testing. The LSTM networks are used to construct the fault prognostics model. Experiments and comparisons are performed to obtain the optimal structure of the networks. Results show that the proposed method generalizes well on the second profile and provides remaining useful life predictions with high accuracy.
更多查看译文
关键词
Sensors,Degradation,Training,Prognostics and health management,Engines,Signal to noise ratio,Diesel engines,Fault prognostics,remaining useful life,marine diesel engine,ship autonomy,prognostic and health management
AI 理解论文
溯源树
样例
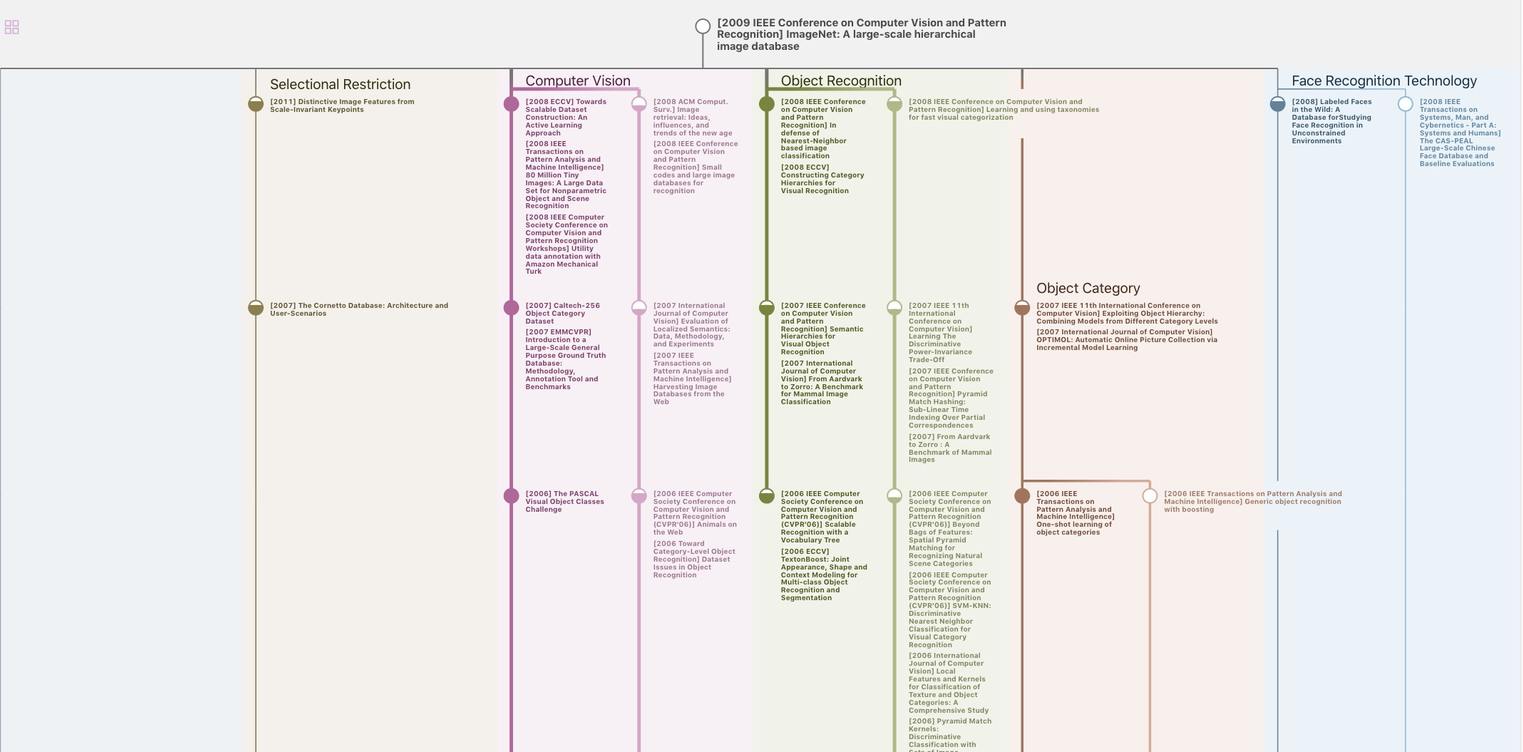
生成溯源树,研究论文发展脉络
Chat Paper
正在生成论文摘要