Graph Transfer Learning
KNOWLEDGE AND INFORMATION SYSTEMS(2023)
摘要
Graph embeddings have been tremendously successful at producing node representations that are discriminative for downstream tasks. In this paper, we study the problem of graph transfer learning: given two graphs and labels in the nodes of the first graph, we wish to predict the labels on the second graph. We propose a tractable, noncombinatorial method for solving the graph transfer learning problem by combining classification and embedding losses with a continuous, convex penalty motivated by tractable graph distances. We demonstrate that our method successfully predicts labels across graphs with almost perfect accuracy; in the same scenarios, training embeddings through standard methods leads to predictions that are no better than random.
更多查看译文
关键词
Graph mining,Transfer learning,Graph distance
AI 理解论文
溯源树
样例
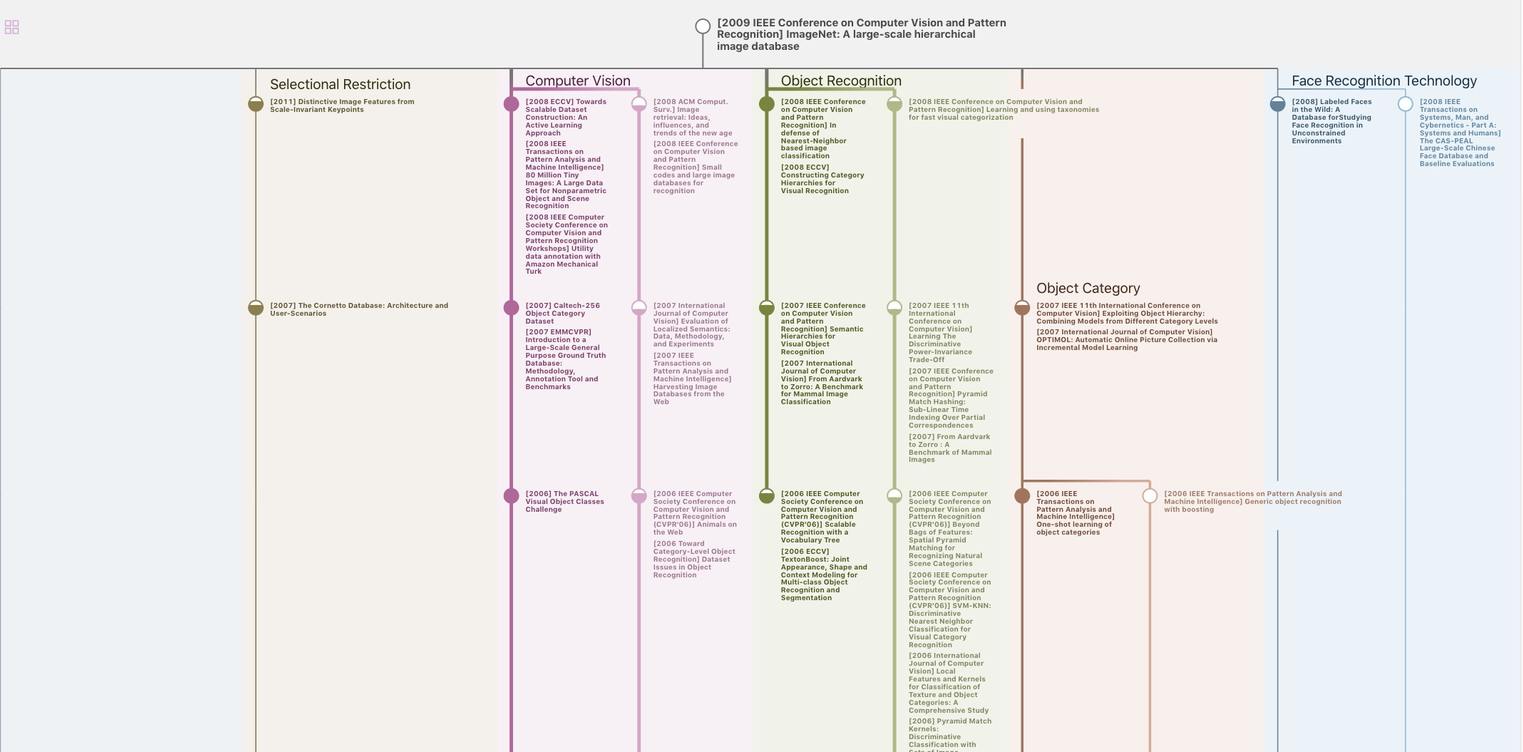
生成溯源树,研究论文发展脉络
Chat Paper
正在生成论文摘要