Generating and Controlling Diversity in Image Search
IEEE Workshop/Winter Conference on Applications of Computer Vision(2022)
Univ Calif San Diego | Adobe Res | Adobe Appl ML
Abstract
In our society, generations of systemic biases have led to some professions being more common among certain genders and races. This bias is also reflected in image search on stock image repositories and search engines, e.g., a query like “male Asian administrative assistant” may produce limited results. The pursuit of a utopian world demands providing content users with an opportunity to present any profession with diverse racial and gender characteristics. The limited choice of existing content for certain combinations of profession, race, and gender presents a challenge to content providers. Current research dealing with bias in search mostly focuses on re-ranking algorithms. However, these methods cannot create new content or change the overall distribution of protected attributes in photos. To remedy these problems, we propose a new task of high-fidelity image generation conditioning on multiple attributes from imbalanced datasets. Our proposed task poses new sets of challenges for the state-of-the-art Generative Adversarial Networks (GANs). In this paper, we also propose a new training framework to better address the challenges. We evaluate our framework rigorously on a real-world dataset and perform user studies that show our model is preferable to the alternatives.
MoreTranslated text
Key words
Explainable AI,Fairness,Accountability,Privacy and Ethics in Vision Datasets,Evaluation and Comparison of Vision Algorithms,Deep Learning -> Neural Generative Models,Autoencoders,GANs,Large-scale Vision Applications
求助PDF
上传PDF
View via Publisher
AI Read Science
AI Summary
AI Summary is the key point extracted automatically understanding the full text of the paper, including the background, methods, results, conclusions, icons and other key content, so that you can get the outline of the paper at a glance.
Example
Background
Key content
Introduction
Methods
Results
Related work
Fund
Key content
- Pretraining has recently greatly promoted the development of natural language processing (NLP)
- We show that M6 outperforms the baselines in multimodal downstream tasks, and the large M6 with 10 parameters can reach a better performance
- We propose a method called M6 that is able to process information of multiple modalities and perform both single-modal and cross-modal understanding and generation
- The model is scaled to large model with 10 billion parameters with sophisticated deployment, and the 10 -parameter M6-large is the largest pretrained model in Chinese
- Experimental results show that our proposed M6 outperforms the baseline in a number of downstream tasks concerning both single modality and multiple modalities We will continue the pretraining of extremely large models by increasing data to explore the limit of its performance
Upload PDF to Generate Summary
Must-Reading Tree
Example
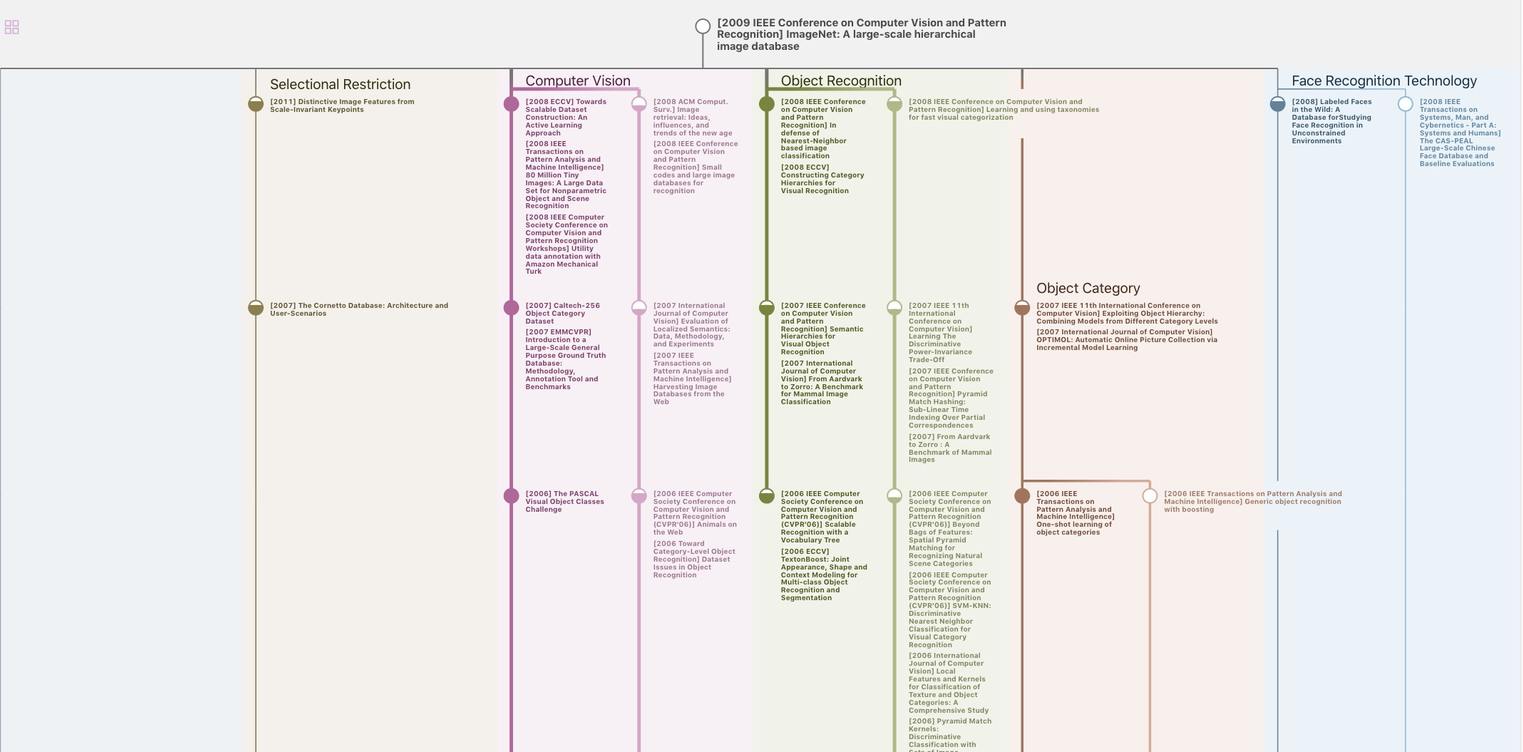
Generate MRT to find the research sequence of this paper
Related Papers
Inspecting the Geographical Representativeness of Images from Text-to-Image Models
Proceedings of the IEEE/CVF International Conference on Computer Vision (ICCV) 2023
被引用35
Debiasing Pretrained Generative Models by Uniformly Sampling Semantic Attributes.
NeurIPS 2023 2023
被引用3
Data Disclaimer
The page data are from open Internet sources, cooperative publishers and automatic analysis results through AI technology. We do not make any commitments and guarantees for the validity, accuracy, correctness, reliability, completeness and timeliness of the page data. If you have any questions, please contact us by email: report@aminer.cn
Chat Paper