Using Knowledge Base to Refine Data Augmentation for Biomedical Relation Extraction KU-AZ team at the BioCreative 7 DrugProt challenge
semanticscholar(2021)
摘要
This paper describes our participation in the BioCreative7 DrugProt challenge. We augmented the DrugProt dataset by predicting labels with transformer models and built a large-scale dataset to expose our model to diverse relation expression patterns. To alleviate the problem of noise inherited to the augmented dataset from the original dataset, we utilized a knowledge base to refine the augmented data points. Our experimental results on the development dataset and the result on the large track test dataset showed that models pre-trained on our augmented dataset produce slightly more accurate predictions. The effects of pretraining models on the augmented dataset varied between relationship types. Performances on rare types (i.e. relation types with smaller populations in the training dataset) benefitted more from the data augmentation method, and recall seemed to improve more than precision. Keywords— Biomedical Relation Extraction; Data Augmentation; Knowledge Base; Transformer; Language Models (key words)
更多查看译文
AI 理解论文
溯源树
样例
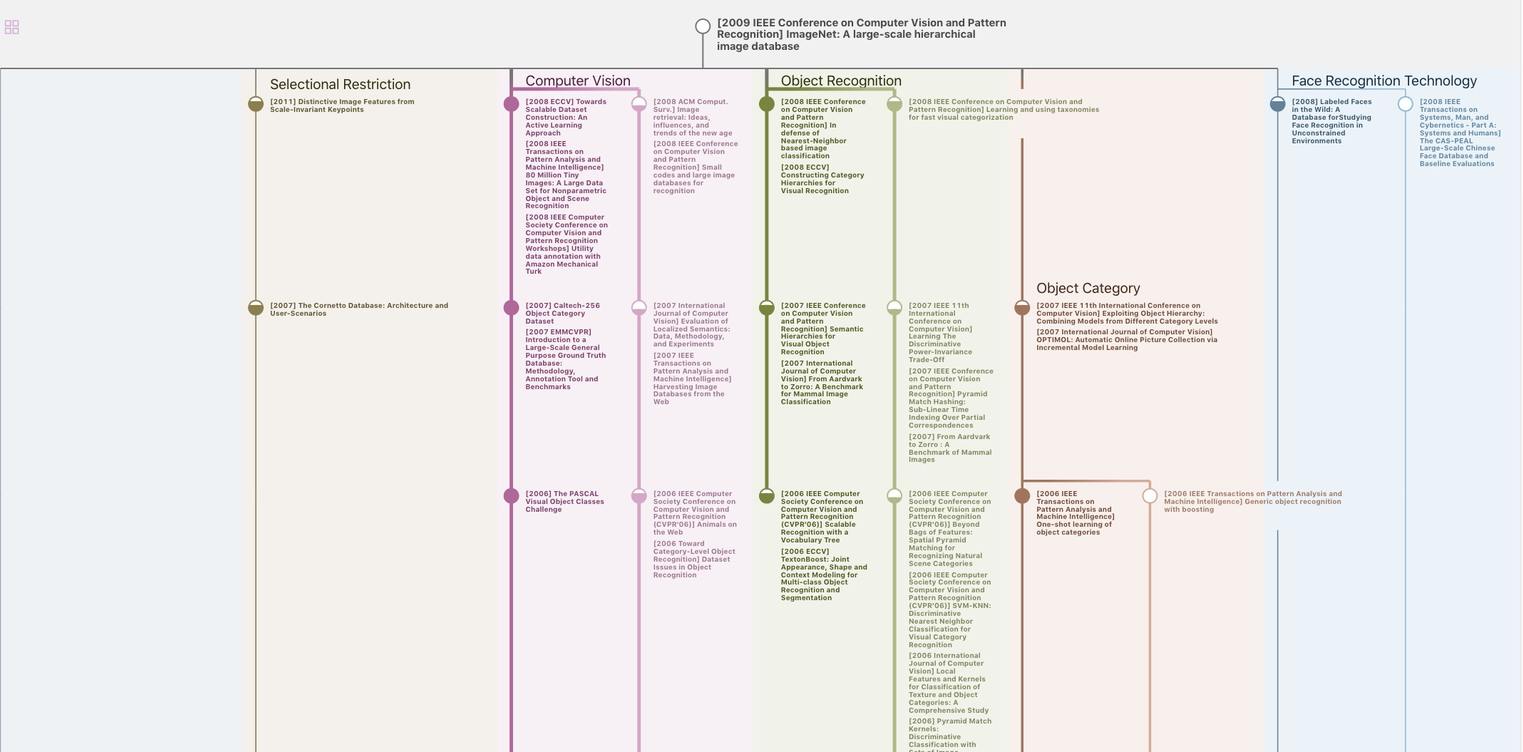
生成溯源树,研究论文发展脉络
Chat Paper
正在生成论文摘要