Supplemental Material for Differentiable Diffusion for Dense Depth Estimation from Multi-view Images
semanticscholar(2021)
摘要
In Table 1, we compare performance on the real-world Stanford and EPFL light fields, with the methods of Zhang at al. [6], Li et al [4], Jiang et al. [1], Shi et al. [5], and the central-view results [2] of Khan et al. [3]. No ground truth depth data exists for these scenes. As a proxy for depth accuracy we use reprojection error×10−2 in RGB color space induced by warping the central view onto the corner views using the estimated disparity map. Our approach is competitive or better than other methods except on the Chess light field, which exhibits strong specular effects due to polished metal materials. However, featureless backgrounds cause our edges to be diffuse (Figure 2). We also include error maps for all light field datasets, along with depth maps for all example light fields listed in the main and supplemental documents. Please see Figures 3 and 4 for synthetic scenes, Figures 5 and 6 for the Stanford scenes, and Figures 7 and 8 for the EPFL scenes. For the additional results presented here, we only run a single pass over each parameter.
更多查看译文
AI 理解论文
溯源树
样例
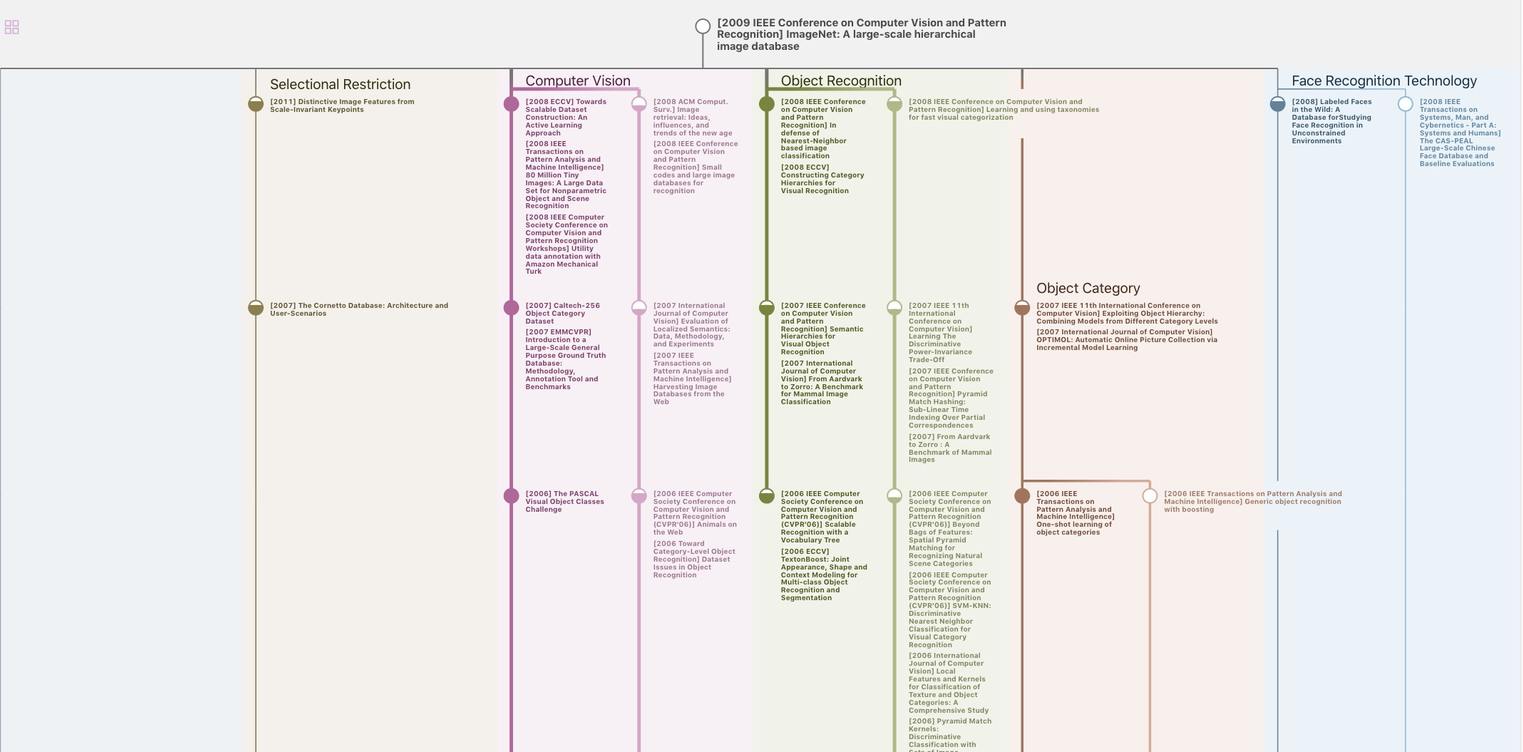
生成溯源树,研究论文发展脉络
Chat Paper
正在生成论文摘要