Deep Polar Residual Network for Identifying Myocardial Infarction in Mce Imaging
semanticscholar(2020)
摘要
Background: Ultrasonic diagnosis of myocardial infarction (MI) varies markedly among radiologists, and is also time-consuming. In addition, there is a high level of inter-observer variation in diagnosis of MI. The purpose of this study is to develop a deep learning-based model that automatically recognizes MI in myocardial contrast echocardiography(MCE) images, and to compare the model performance against radiologists with varying experience in echocardiography.Methods: In this study, a polar residual network (PResNet) model was employed to recognize MI segments in MCE images of Sprague-Dawley (SD) rats. All SD rats were randomly divided into the MI model group (n=15) and the sham group (n=10). Rats in MI model group were infarcted via left anterior descending coronary artery ligation. Ultimately, MCE was performed on SD rats to observe their myocardium. Left ventricle (LV) short-axis views of MCE at the basal and midpapillary muscle levels for each SD rat were divided into 6 segments separately. The PResNet model and six radiologists each diagnosed 276 MCE image segments. Masson’s trichrome staining was used as the gold standard for MI presence or absence. The PResNet model and radiologist diagnostic performance were evaluated using sensitivity, specificity, and area under the receiver operating characteristic curve (AUC). Furthermore, analysis duration per rat and interobserver variability were also compared. Results: Masson’s trichrome stain revealed 30 infarcted segments out of the 276 total segments. The PResNet model achieved an AUC of 0.89 (0.85-0.93), which was comparable to that of senior radiologists (0.89; 0.85-0.93). Relative to senior radiologists, the PResNet model presented slightly lower sensitivity but significantly higher specificity. The mean sensitivity and specificity for the junior radiologists were significantly lower than both the PResNet model and the senior radiologists. In terms of analysis duration, the PResNet model (148-169sec) was markedly faster than both junior (314-366sec) and senior (193-235sec). Moreover, the intrasample agreement for the PResNet model (k=0.96) was better than the interobserver agreement for the junior (k=0.81) and senior radiologists (k=0.86). Conclusion: The PResNet model not only improved diagnostic performance and observer consensus but also improve the diagnose speed. It is a promising tool for identifying MI.
更多查看译文
AI 理解论文
溯源树
样例
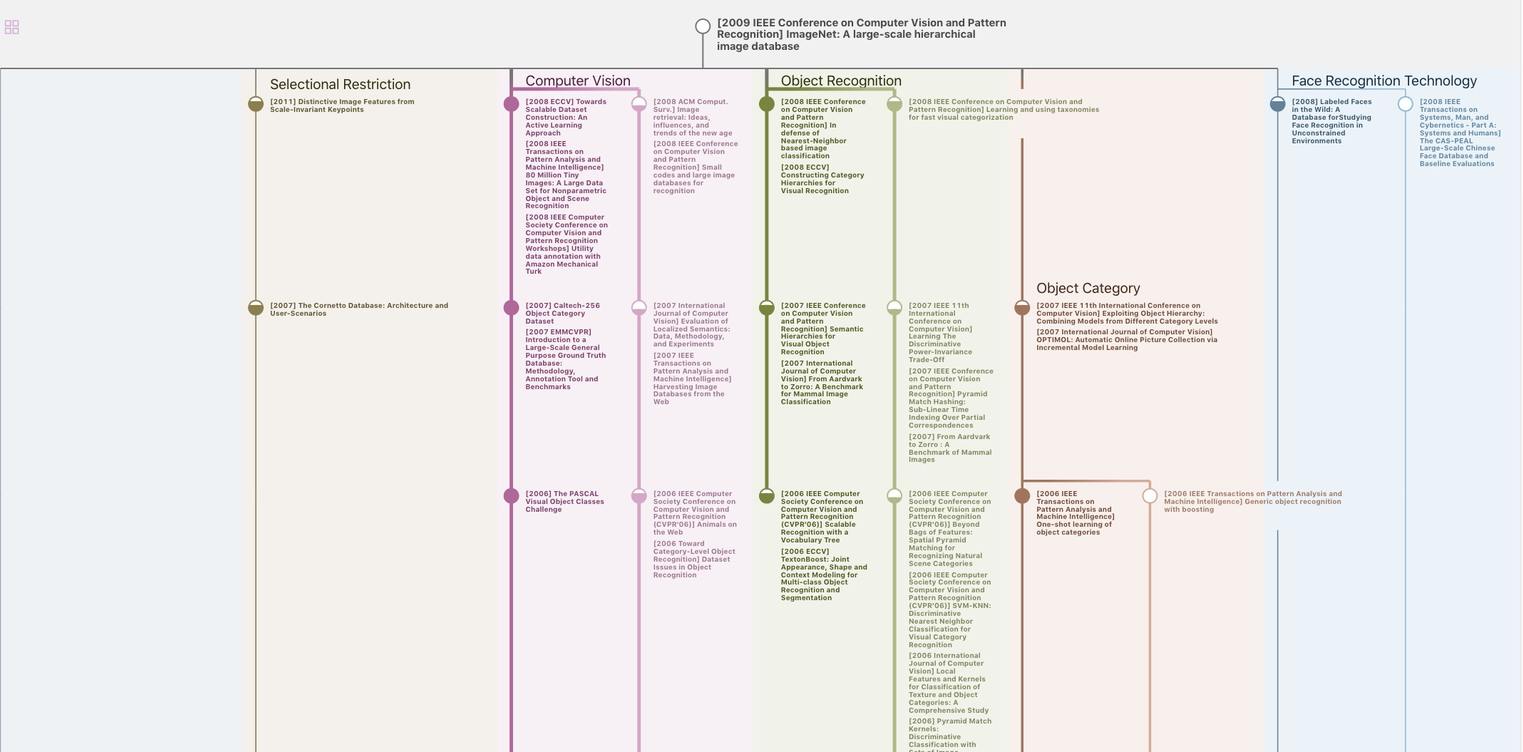
生成溯源树,研究论文发展脉络
Chat Paper
正在生成论文摘要