Moving Obstacle Segmentation with an Optical Flow-based DNN: an Implementation Case Study
2021 IEEE 25th International Conference on Intelligent Engineering Systems (INES)(2021)
摘要
Moving object detection is a crucial component of automotive systems' safety functions. AI-based approaches for object detection are the most common solutions in the case of self-driving vehicles. For autonomous navigation in an industrial setting, a deep learning model that relies on stronger assumptions regarding the environment, can be implemented. This is due to the fact that an industrial environment is more strictly controlled than an urban area. However, the detection of moving obstacles is still a challenging task and its solution can serve as the basis for more advanced models. In this paper, we introduce an optical flow-based deep neural network approach for moving object segmentation and state of motion estimation in industrial environment as an implementation case study. The algorithm is based on our earlier optical flow egomotion filtering method and optical flow-based Deep Neural Network, called OFSNet. The aim of this paper is to introduce the main hardware and software modules of the moving object segmentation system, the integration process, the communication considerations and the logistics management system. The proposed system was installed and tested on a mobile robot platform in a mock warehouse environment. All of the program codes, documentations and installation steps are publicly available at GitHub.
更多查看译文
关键词
self-driving systems,warehouse automation,object detection,optical flow,deep neural network
AI 理解论文
溯源树
样例
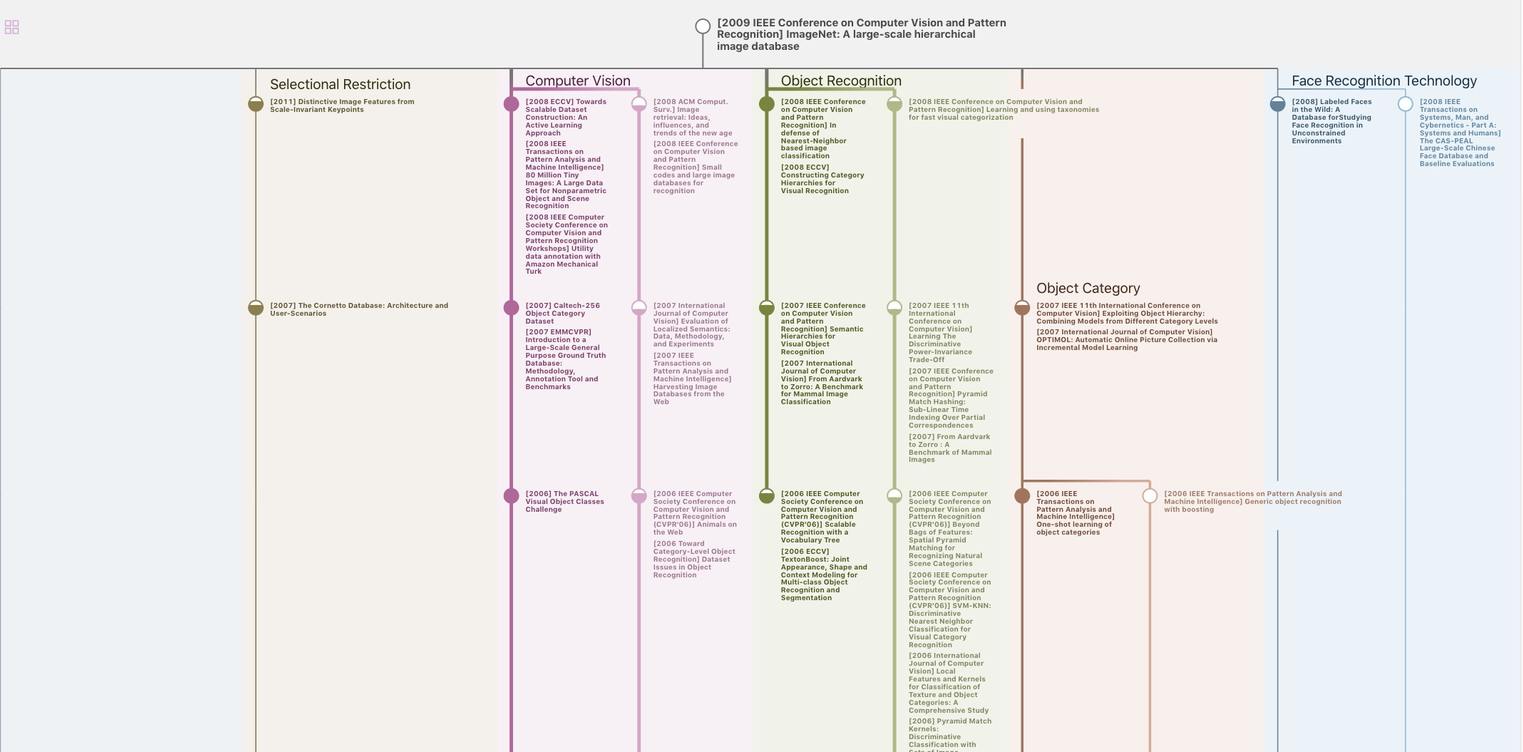
生成溯源树,研究论文发展脉络
Chat Paper
正在生成论文摘要