A novel orthogonal self-attentive variational autoencoder method for interpretable chemical process fault detection and identification
PROCESS SAFETY AND ENVIRONMENTAL PROTECTION(2021)
摘要
Industrial processes are becoming increasingly large and complex, thus introducing potential safety risks and requiring an effective approach to maintain safe production. Intelligent process monitoring is critical to prevent losses and avoid casualties in modern industry. As the digitalization of process industry deepens, data-driven methods offer an exciting avenue to address the demands for monitoring complex systems. Nevertheless, many of these methods still suffer from low accuracy and slow response. Besides, most blackbox models based on deep learning can only predict the existence of faults, but cannot provide further interpretable analysis, which greatly confines their usage in decision-critical scenarios. In this paper, we propose a novel orthogonal self-attentive variational autoencoder (OSAVA) model for process monitoring, consisting of two components, orthogonal attention (OA) and variational self-attentive autoencoder (VSAE). Specifically, OA is utilized to extract the correlations between different variables and the temporal dependency among different timesteps; VSAE is trained to detect faults through a reconstruction-based method, which employs self-attention mechanisms to comprehensively consider information from all timesteps and enhance detection performance. By jointly leveraging these two models, the OSAVA model can effectively perform fault detection and identification tasks simultaneously and deliver interpretable results. Finally, extensive evaluation on the Tennessee Eastman process (TEP) demonstrates that the proposed OSAVA-based fault detection and identification method shows promising fault detection rate as well as low detection delay and can provide interpretable identification of the abnormal variables, compared with representative statistical methods and state-of-the-art deep learning methods. (c) 2021 Institution of Chemical Engineers. Published by Elsevier B.V. All rights reserved.
更多查看译文
关键词
Process monitoring,Orthogonal attention,Variational self-attentive autoencoder,Fault detection,Fault identification
AI 理解论文
溯源树
样例
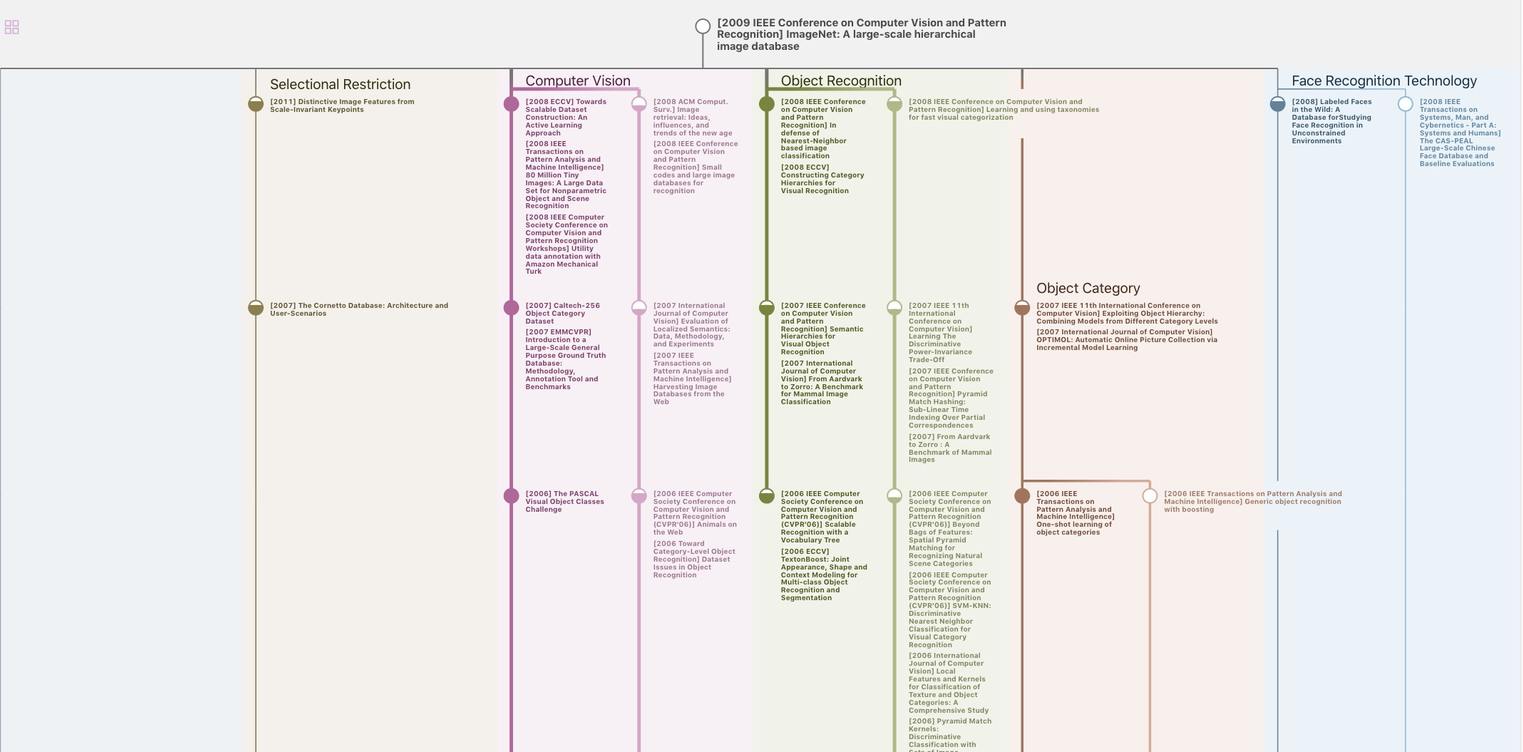
生成溯源树,研究论文发展脉络
Chat Paper
正在生成论文摘要