Non-Volatile Memory Accelerated Posterior Estimation
arXiv (Cornell University)(2022)
Abstract
Bayesian inference allows machine learning models to express uncertainty. Current machine learning models use only a single learnable parameter combination when making predictions, and as a result are highly overconfident when their predictions are wrong. To use more learnable parameter combinations efficiently, these samples must be drawn from the posterior distribution. Unfortunately computing the posterior directly is infeasible, so often researchers approximate it with a well known distribution such as a Gaussian. In this paper, we show that through the use of high-capacity persistent storage, models whose posterior distribution was too big to approximate are now feasible, leading to improved predictions in downstream tasks.
MoreTranslated text
Key words
Robust Learning,Variational Inference,Bayesian Inference,Semi-Supervised Learning,Probabilistic Models
AI Read Science
Must-Reading Tree
Example
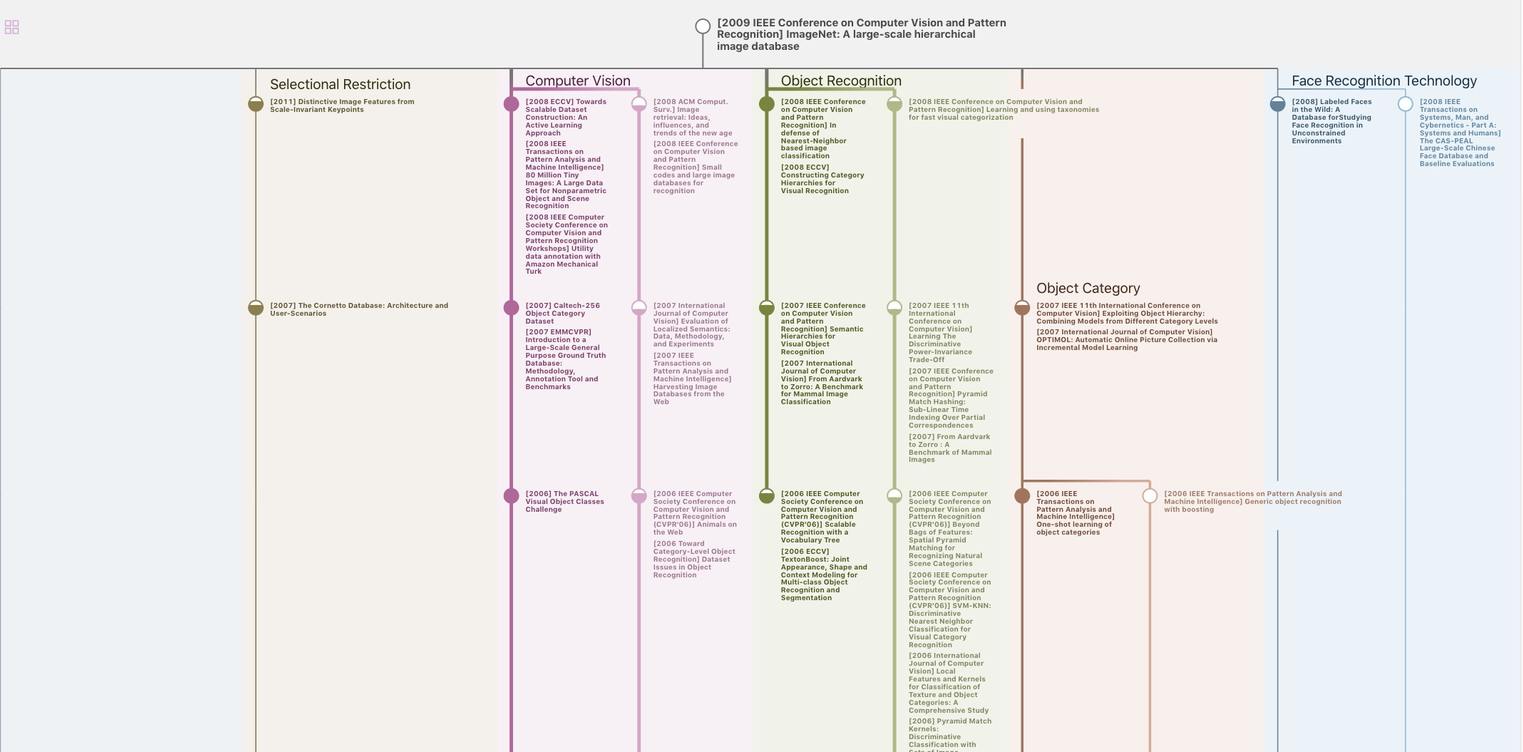
Generate MRT to find the research sequence of this paper
Chat Paper
Summary is being generated by the instructions you defined