Clonal spheroids capture functional and genetic heterogeneity of head and neck cancer
crossref(2024)
School of Biotechnology | Cancer Biology Laboratory | Special Centre for Molecular Medicine | Cancer Biology Research and Training Program | Department of Cell Development & Cancer Biology | Division of Radiation Oncology | All India Institute of Medical Sciences
Abstract
Head and neck cancer squamous cell carcinoma (HNSCC) cells exhibit both structural and functional diversity, making them valuable models for understanding tumor heterogeneity at clinical levels. In this study, we generated single-cell-derived spheroids (SCDS) from HNSCC cell lines and patient tumor cells using scaffold- and non-scaffold-based methods to assess this variability. A distinct structural variability among these SCDS, categorized as hypo- and hyperproliferative spheroids based on size, was observed. Hyperproliferative spheroids demonstrated heightened proliferative and tumorigenic potential and increased sensitivity to cisplatin and radiation, while hypoproliferative spheroids exhibited enhanced migratory capabilities. Single-cell RNA sequencing (scRNA-seq) of hypo- and hyperproliferative spheroids provided insights into the transcriptional landscape of HNSCC cells, validating the observed structural and functional heterogeneities within primary tumors. These functionally and genetically characterized spheroids offer valuable tools for the development of next-generation therapeutics.
Statement of Significance Establishment and characterization of single-cell-derived spheroids from head and neck cancer cells, employing scaffold and non-scaffold materials, demonstrate functional and genetic heterogeneity. Single-cell analysis reveals correlations between genetic diversity and spheroid functionality. These characterized spheroids offer potential for advancing therapeutics development.
### Competing Interest Statement
The authors have declared no competing interest.
MoreTranslated text
求助PDF
上传PDF
View via Publisher
AI Read Science
AI Summary
AI Summary is the key point extracted automatically understanding the full text of the paper, including the background, methods, results, conclusions, icons and other key content, so that you can get the outline of the paper at a glance.
Example
Background
Key content
Introduction
Methods
Results
Related work
Fund
Key content
- Pretraining has recently greatly promoted the development of natural language processing (NLP)
- We show that M6 outperforms the baselines in multimodal downstream tasks, and the large M6 with 10 parameters can reach a better performance
- We propose a method called M6 that is able to process information of multiple modalities and perform both single-modal and cross-modal understanding and generation
- The model is scaled to large model with 10 billion parameters with sophisticated deployment, and the 10 -parameter M6-large is the largest pretrained model in Chinese
- Experimental results show that our proposed M6 outperforms the baseline in a number of downstream tasks concerning both single modality and multiple modalities We will continue the pretraining of extremely large models by increasing data to explore the limit of its performance
Upload PDF to Generate Summary
Must-Reading Tree
Example
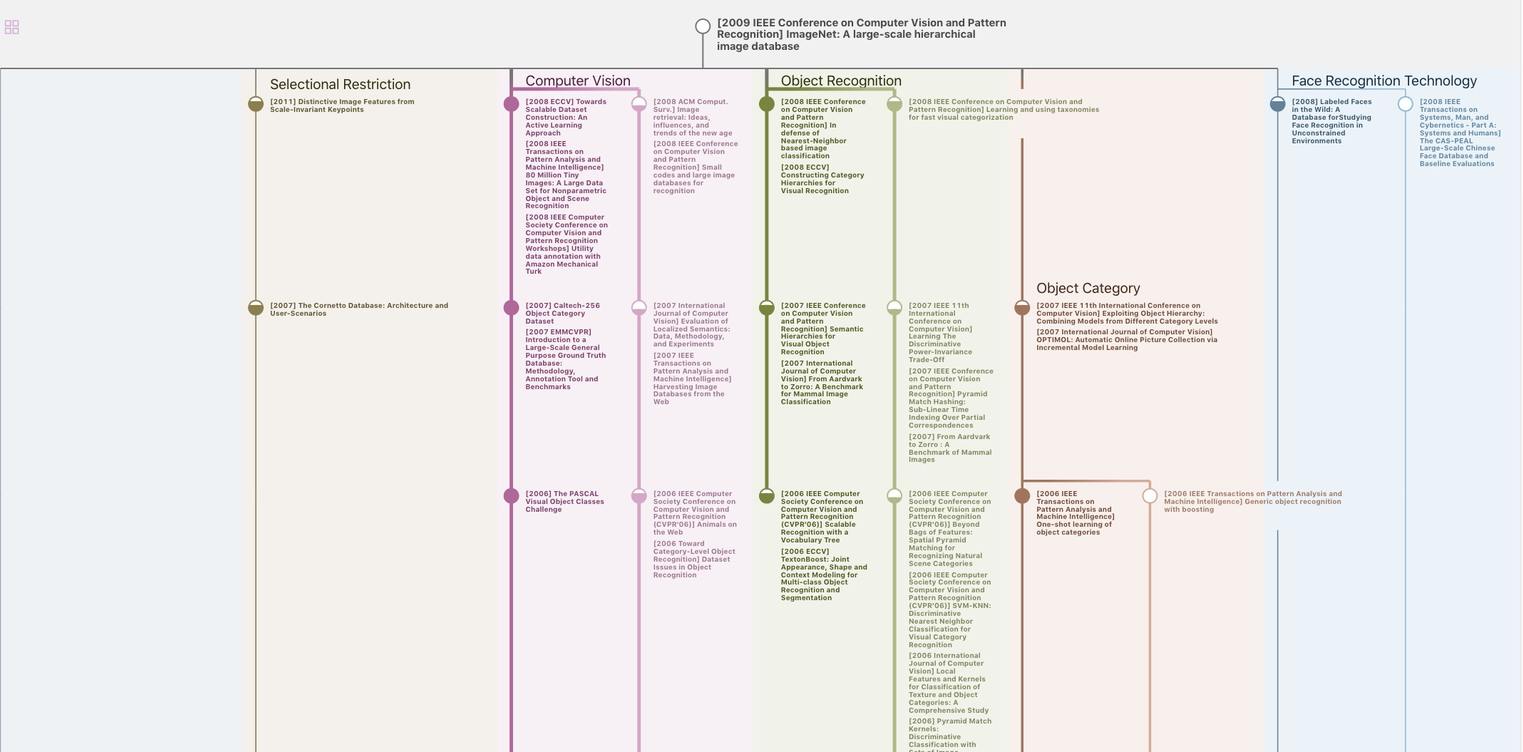
Generate MRT to find the research sequence of this paper
Data Disclaimer
The page data are from open Internet sources, cooperative publishers and automatic analysis results through AI technology. We do not make any commitments and guarantees for the validity, accuracy, correctness, reliability, completeness and timeliness of the page data. If you have any questions, please contact us by email: report@aminer.cn
Chat Paper
GPU is busy, summary generation fails
Rerequest