Transferring Domain Knowledge with an Adviser in Continuous Tasks
Advances in Knowledge Discovery and Data Mining 25th Pacific-Asia Conference, PAKDD 2021, Virtual Event, May 11–14, 2021, Proceedings, Part III(2021)
摘要
Recent advances in Reinforcement Learning (RL) have surpassed human-level performance in many simulated environments. However, existing reinforcement learning techniques are incapable of explicitly incorporating already known domain-specific knowledge into the learning process. Therefore, the agents have to explore and learn the domain knowledge independently through a trial and error approach, which consumes both time and resources to make valid responses. Hence, we adapt the Deep Deterministic Policy Gradient (DDPG) algorithm to incorporate an adviser, which allows integrating domain knowledge in the form of pre-learned policies or pre-defined relationships to enhance the agent’s learning process. Our experiments on OpenAi Gym benchmark tasks show that integrating domain knowledge through advisers expedites the learning and improves the policy towards better optima.
更多查看译文
关键词
Actor-critic architecture,Deterministic policy gradient,Reinforcement learning,Transferring domain knowledge
AI 理解论文
溯源树
样例
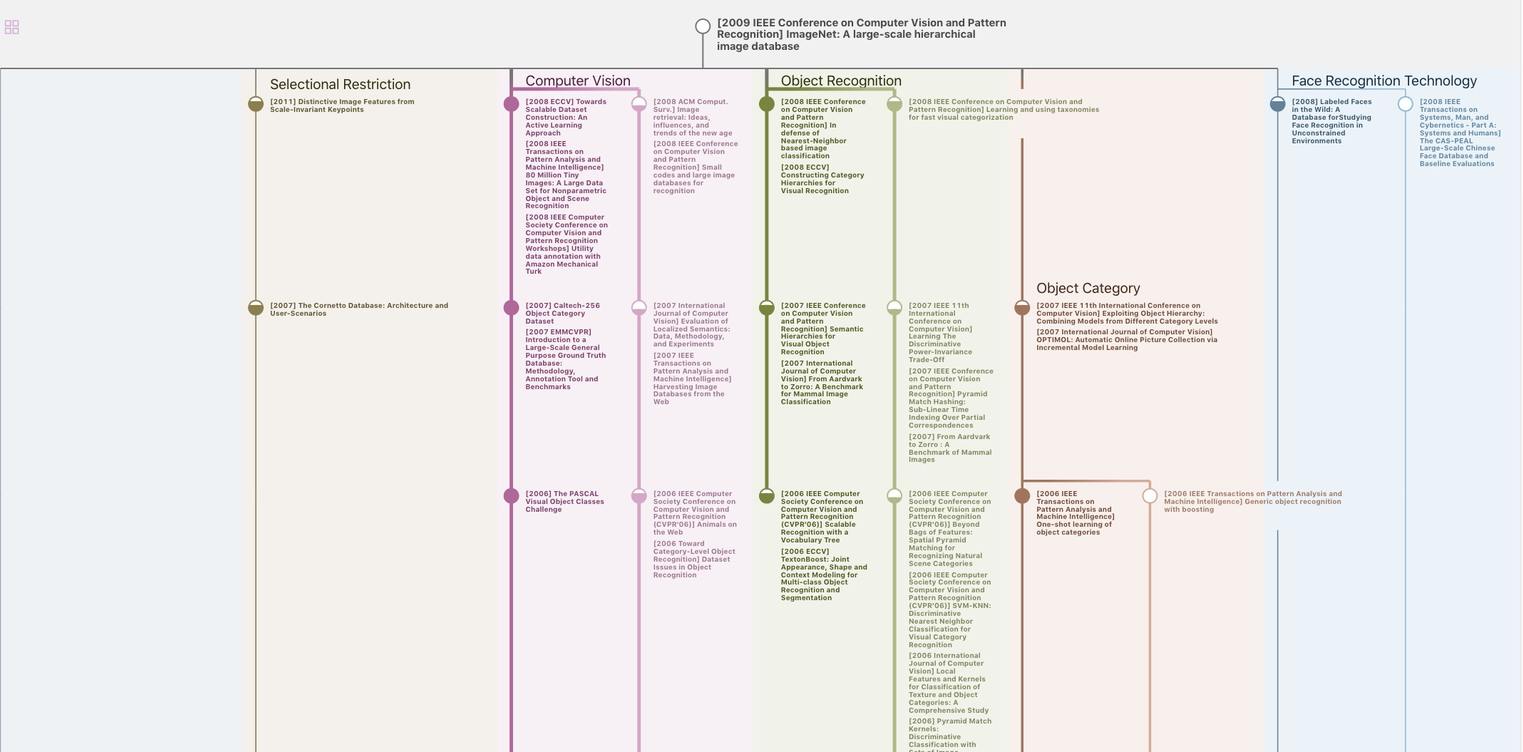
生成溯源树,研究论文发展脉络
Chat Paper
正在生成论文摘要