Copy Number Alterations Predict the Survival of Early Colorectal Cancer with Depressed Morphology
SSRN Electronic Journal(2019)
Abstract
Background: Depressed colorectal neoplasms exhibit a high malignant potential and may become invasive rapidly but their genomic alterations remain unknown. We investigated the genomic alterations of depressed neoplasms and potential implications on long-term survival. Methods: We examined 20 depressed neoplasms and 15 polypoid neoplasms by genome-wide copy number analysis. Subsequently, we validated the identified copy number alterations in an independent sample of 37 depressed and 42 polypoid neoplasms. Specific copy number alterations were tested in 530 colorectal cancers in order to clarify the clinical outcome of depressed colorectal neoplasms. Findings: Copy number alterations in MYC, CCNA1, and BIRC7 were significantly enriched in depressed neoplasms and designated as the D-marker panel. In the validation set, the panel presented a positive predictive value, sensitivity and accuracy of 80·0%, 75·7% and 79·7% respectively for discriminating the depressed neoplasms. colorectal cancers developing from depressed neoplasms account for 22·1% of all colorectal cancers and have a significantly more unfavorable progression-free survival compared with colorectal cancer without those biomarkers, especially in stage I, T1+2, and proximal cancers. Interpretation: In this article, we characterize the genome-wide landscape in the depressed neoplasm and its specific biosignature. The D-marker panel may help to optimize the treatment of depressed neoplasms and surveillance strategies, and develop more effective molecular screening tests in the future. Funding Statement: Funding by the National Taiwan University Hospital (Grand Number: NTUH 107-M3990, NTUH 108-M4209), Ministry of Science and Technology, Taiwan (Grant Number: MOST-105-2314-B-002-047, MOST106-2319-B-002-002, MOST107-2319-B-002-002), and as well as the “Center of Precision Medicine” from The Featured Areas Research Center Program within the framework of the Higher Education Sprout Project by the Ministry of Education (MOE) in Taiwan.Declaration of Interests: The authors declare that they have no conflicts of interest.Ethics Approval Statement: Before initiation, this study received approval (No. 201712033RINC) from the Institutional Review Board and Ethical Committee of National Taiwan University Hospital.
MoreTranslated text
求助PDF
上传PDF
View via Publisher
AI Read Science
AI Summary
AI Summary is the key point extracted automatically understanding the full text of the paper, including the background, methods, results, conclusions, icons and other key content, so that you can get the outline of the paper at a glance.
Example
Background
Key content
Introduction
Methods
Results
Related work
Fund
Key content
- Pretraining has recently greatly promoted the development of natural language processing (NLP)
- We show that M6 outperforms the baselines in multimodal downstream tasks, and the large M6 with 10 parameters can reach a better performance
- We propose a method called M6 that is able to process information of multiple modalities and perform both single-modal and cross-modal understanding and generation
- The model is scaled to large model with 10 billion parameters with sophisticated deployment, and the 10 -parameter M6-large is the largest pretrained model in Chinese
- Experimental results show that our proposed M6 outperforms the baseline in a number of downstream tasks concerning both single modality and multiple modalities We will continue the pretraining of extremely large models by increasing data to explore the limit of its performance
Upload PDF to Generate Summary
Must-Reading Tree
Example
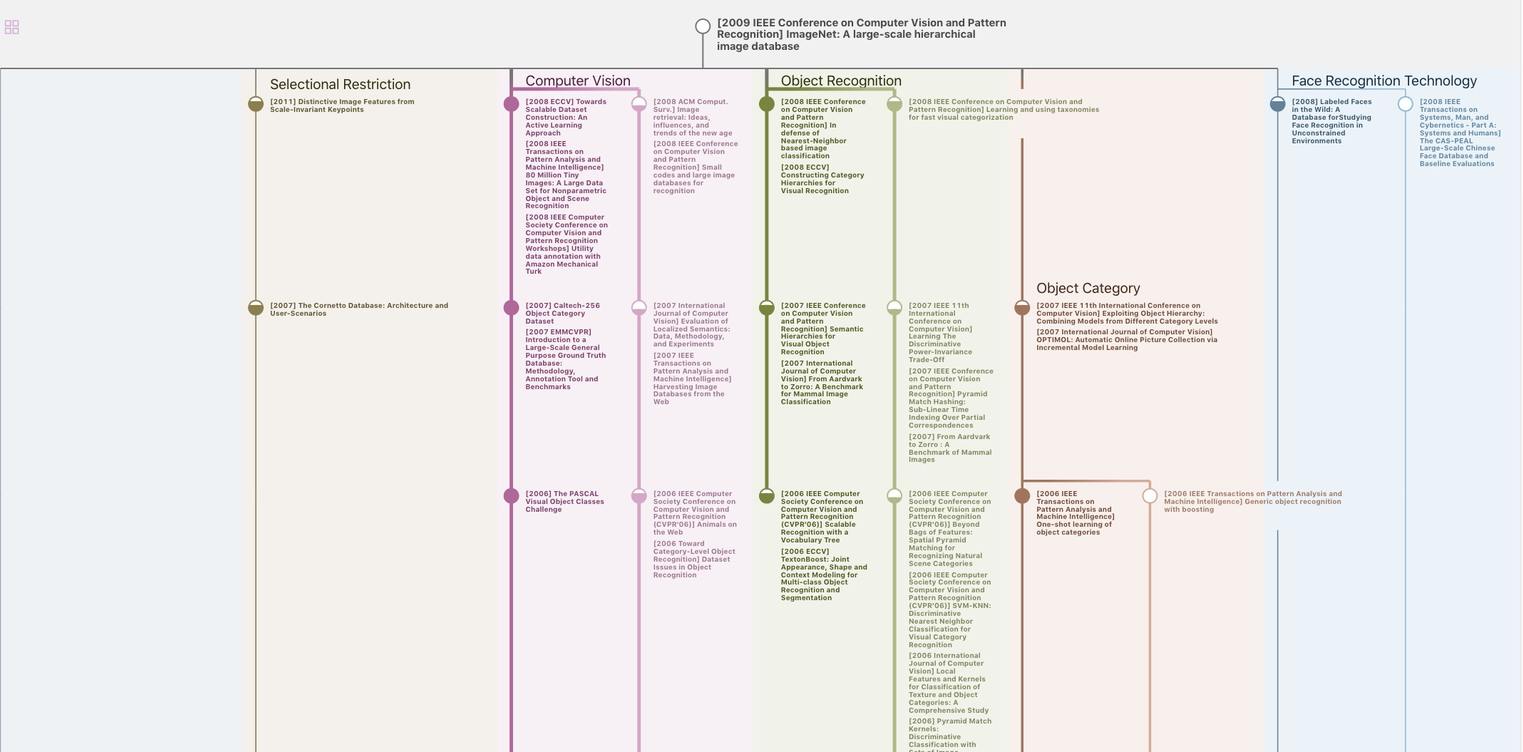
Generate MRT to find the research sequence of this paper
Data Disclaimer
The page data are from open Internet sources, cooperative publishers and automatic analysis results through AI technology. We do not make any commitments and guarantees for the validity, accuracy, correctness, reliability, completeness and timeliness of the page data. If you have any questions, please contact us by email: report@aminer.cn
Chat Paper
GPU is busy, summary generation fails
Rerequest