G-OWL: A Complete Visual Syntax for OWL 2 Ontology Modeling and Communication
semanticscholar
Abstract
Semantic web ontologies are usually modeled using standard text-based syntaxes such as OWL/XML, Functional, Manchester or Turtle. Over recent years, there has been an increasing need for representing ontologies visually to help ontological engineers or modelers represent elicited knowledge from domain expert, big data, model data structures or simply present data schemas and metadata to general users. We believe a visual representation is an essential way for understanding knowledge and to help elaborate formal ontologies for their communication and their use by humans. In this paper, we present the Graphical Ontology Web Language (G-OWL), a visual syntax for the graphical modeling and visualization of OWL 2 or RDFS ontologies. In line with previous research in cognitive science, G-OWL uses syntactic and semantic principles that simplify both its use and its interpretation. Indeed, the use of typology and polymorphism makes it possible to minimize the number of visual signs in a grammar, thus reducing the cognitive load on users, while preserving the formal character of the ontology. This G-OWL visual syntax is integrated in a software tool called OntoCASE4G-OWL to support the elaboration of ontologies and their translation to standard text-based syntaxes such as Turtle. This paper aims to present the definition of the G-OWL visual syntax and to demonstrate its highly readable character through an objective assessment of criteria such as: semiotic clarity, semantic transparency and graphic complexity. The G-OWL visual syntax will also be compared with other visual syntaxes and will be evaluated in order to measure its highly human-readability in reading activities, modeling or new knowledge deduction by novice, intermediate and expert in ontology modeling.
MoreTranslated text
求助PDF
上传PDF
AI Read Science
AI Summary
AI Summary is the key point extracted automatically understanding the full text of the paper, including the background, methods, results, conclusions, icons and other key content, so that you can get the outline of the paper at a glance.
Example
Background
Key content
Introduction
Methods
Results
Related work
Fund
Key content
- Pretraining has recently greatly promoted the development of natural language processing (NLP)
- We show that M6 outperforms the baselines in multimodal downstream tasks, and the large M6 with 10 parameters can reach a better performance
- We propose a method called M6 that is able to process information of multiple modalities and perform both single-modal and cross-modal understanding and generation
- The model is scaled to large model with 10 billion parameters with sophisticated deployment, and the 10 -parameter M6-large is the largest pretrained model in Chinese
- Experimental results show that our proposed M6 outperforms the baseline in a number of downstream tasks concerning both single modality and multiple modalities We will continue the pretraining of extremely large models by increasing data to explore the limit of its performance
Upload PDF to Generate Summary
Must-Reading Tree
Example
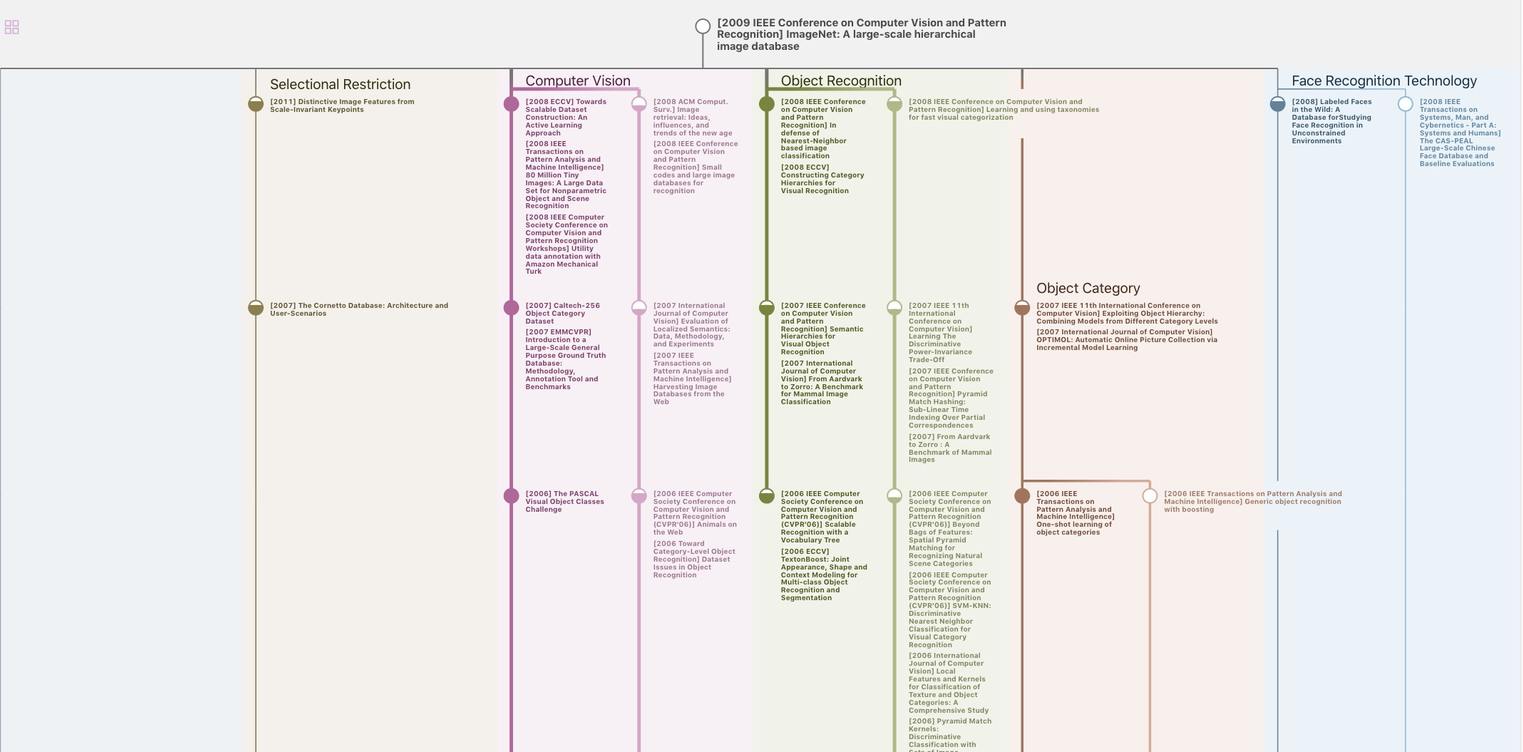
Generate MRT to find the research sequence of this paper
Related Papers
Problem Definition of Modelling and Simulation of Industrial DC Microgrids
2023 IEEE Fifth International Conference on DC Microgrids (ICDCM) 2023
被引用0
Data Disclaimer
The page data are from open Internet sources, cooperative publishers and automatic analysis results through AI technology. We do not make any commitments and guarantees for the validity, accuracy, correctness, reliability, completeness and timeliness of the page data. If you have any questions, please contact us by email: report@aminer.cn
Chat Paper
GPU is busy, summary generation fails
Rerequest