Learning ECG Representations for Multi-Label Classification of Cardiac Abnormalities.
2021 Computing in Cardiology (CinC)(2021)
摘要
The goal of PhysioNet/Computing in Cardiology Challenge 2021 was to identify clinical diagnoses from 12-lead and reduced-lead ECG recordings, including 6-lead, 4-lead, 3-lead, and 2-lead recordings. Our team, snu-adsl, have used EfficientNet-B3 as the base deep learning model and have investigated methods including data augmentation, self-supervised learning as pre-training, label masking that deals with multiple data sources, threshold optimization, and feature extraction. Self-supervised learning showed promising results when the size of labeled dataset was limited, but the competition's dataset turned out to be large enough that the actual gain was marginal. In consequence, we did not include self-supervised pre-training in our final entry. Our classifiers received scores of 0.48, 0.48, 0.47, 0.47, and 0.45 (ranked 12th, 10th, 11th, 11th, and 13th out of 39 teams) for the 12-lead, 6-lead, 4-lead, 3-lead, and 2-lead versions of the hidden test set with the Challenge evaluation metric.
更多查看译文
关键词
ecg representations,cardiac abnormalities,classification,multi-label
AI 理解论文
溯源树
样例
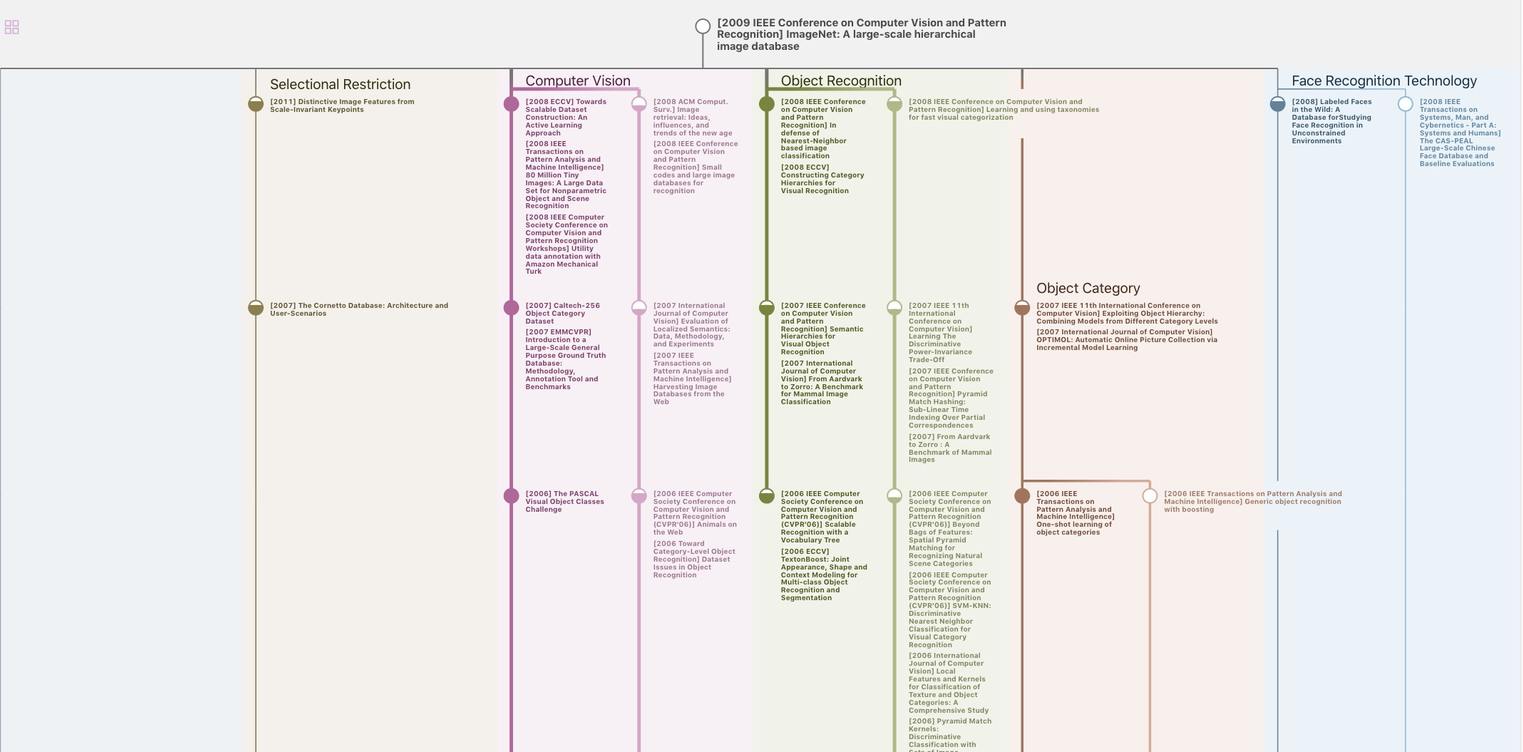
生成溯源树,研究论文发展脉络
Chat Paper
正在生成论文摘要