IDENTIFYING POTENTIAL SOURCES OF BIAS IN DEEP LEARNING MODELS FOR EMBRYO ASSESSMENT
Fertility and sterility(2021)
摘要
To identify and reduce potential sources of bias when training deep learning models for analyzing images of human embryos. Historical, de-identified images of blastocyst-stage embryos were collected from 11 IVF clinics in the United States between 2015-2020. Each laboratory captured a single image using their existing inverted microscope, stereo zoom microscope, or time-lapse microscope. Approximately 8,000 images were matched to positive clinical pregnancy, negative clinical pregnancy, or PGT-A aneuploid result. We trained a series of deep convolutional neural networks (CNNs) to rank embryo images according to their likelihood of having a positive or negative outcome. Experiments were performed using different techniques for combining images from the clinical sites, including naive and balanced methods. For each experiment, the aggregated data was split into 70% train and 30% test. The area under the receiver operating curve (AUC) was used for evaluating the ability of the models to rank embryos according to their likelihood of achieving a positive outcome. Total and per-clinic AUCs, as well as total and per-clinic inference probabilities, were evaluated for each experiment to identify and reduce potential sources of bias. Using a naive approach for combining data together from all clinics achieved the highest total AUC for the test set (0.75) but also the lowest per-clinic AUC (0.51). Investigation of this discrepancy revealed two strong sources of bias, which artificially inflated the total AUC and significantly limited per-clinic performance. The biases included the unique optical signature of each type of microscope, and the presence of foreign objects, such as a holding micropipette present in the image. If a certain optical signature or foreign artifact appeared more in the positive training class compared to the negative training class, the CNN models were found to learn these biases and give a higher score to those images regardless of the embryo morphology. With these insights, a new dataset was prepared that balanced the ratios of positive-to-negative samples for each type of microscope and for each group containing foreign objects. This provided a non-inflated total AUC of 0.72 and significantly raised the lowest per-site AUC from 0.51 to 0.61. There has been significant recent interest in using deep learning for analyzing images of embryos at the blastocyst stage. The black-box nature of deep learning models such as CNNs makes it difficult to recognize when potential sources of bias have been introduced during the training process. We performed a series of experiments that identified and reduced two sources of bias, and improved per-clinic performance of the CNN. Future work will continue to search for other sources of bias and address them accordingly.
更多查看译文
AI 理解论文
溯源树
样例
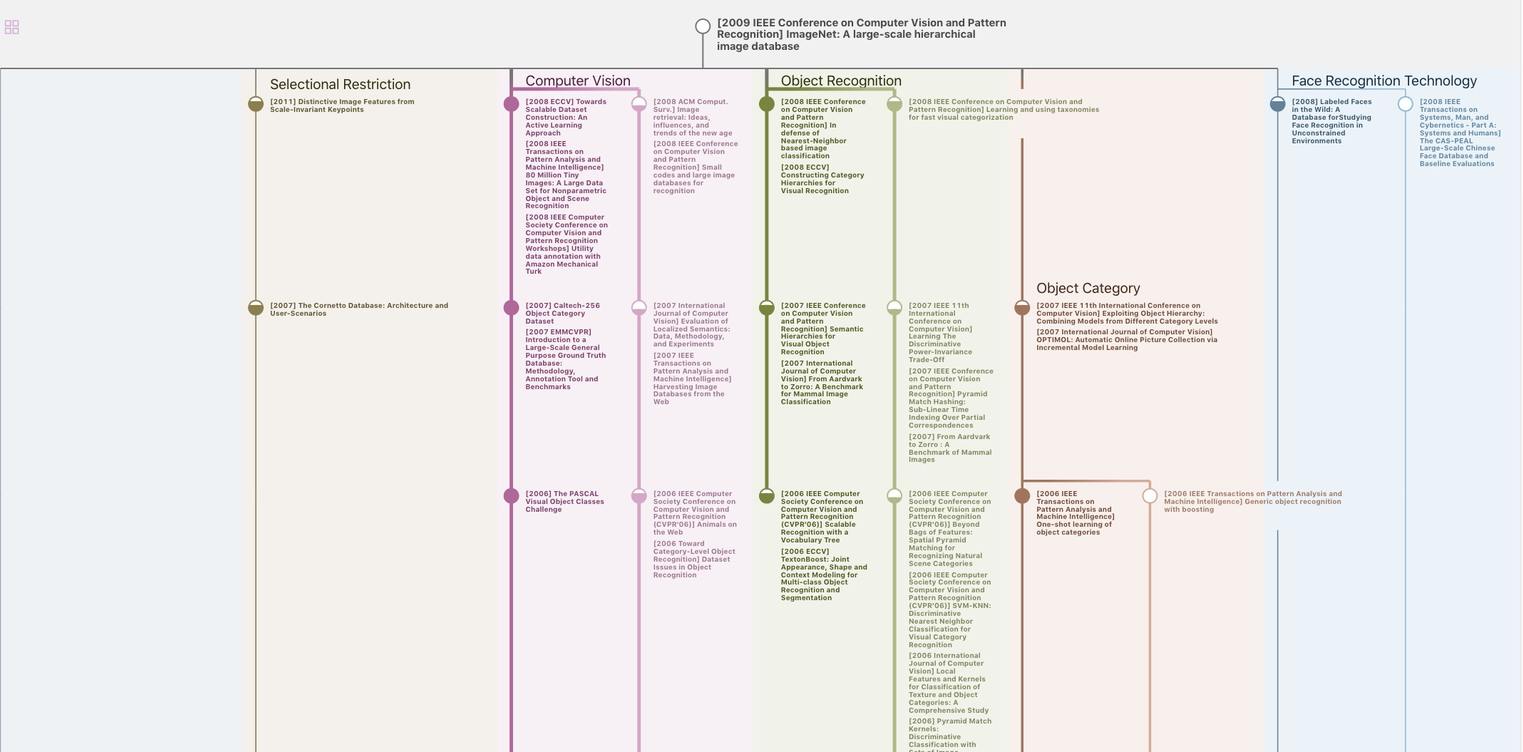
生成溯源树,研究论文发展脉络
Chat Paper
正在生成论文摘要