Methodological Issues to Analyse Real-World Longitudinal Occupational Health Data (Preprint)
semanticscholar(2021)
摘要
UNSTRUCTURED Ever greater technological advances and democratization of digital tools such as computers and smartphones offer researchers new possibilities to collect large amounts of health data in order to conduct clinical research. Such data, called real-world data (RWD), appears to be a perfect complement to traditional randomized clinical trials (RCTs) and has become more important in health decisions. Due to its longitudinal nature, RWD is subject to well-known methodological issues that can occur when collecting this type of data. In this article, we present the three main methodological problems encountered by researchers, these include, the longitudinal data itself, missing data (not available - NA) and cluster-correlated data. These concepts have been widely discussed in the literature and many methods and solutions have been proposed to cope with these issues. As examples, mixed and trajectory models have been developed to explore longitudinal data sets, imputation methods can resolve NA issues, and multilevel models facilitate treating cluster-correlated data. This article reviews the various solutions proposed and attempts to analyze all three in detail. Although solutions exist to meet these data collection challenges, solutions are not always correctly exploited, especially in cases where data collection issues overlap. In an attempt to solve this problem, we have conceived a process that considers all three issues simultaneously. This process can be divided into two parts: the first part of data management comprises of several phases such as definition of data structure, identification of suspect data and application of imputation methods. The second part of the analysis relates to the application of different models for repeated data using the modified data set. As a result, it should be possible to facilitate work with data sets and provide results with higher confidence levels. To support our proposal, we have used results from the “Wittyfit” database, which is an epidemiological database of occupational health data.
更多查看译文
关键词
Cohort Study
AI 理解论文
溯源树
样例
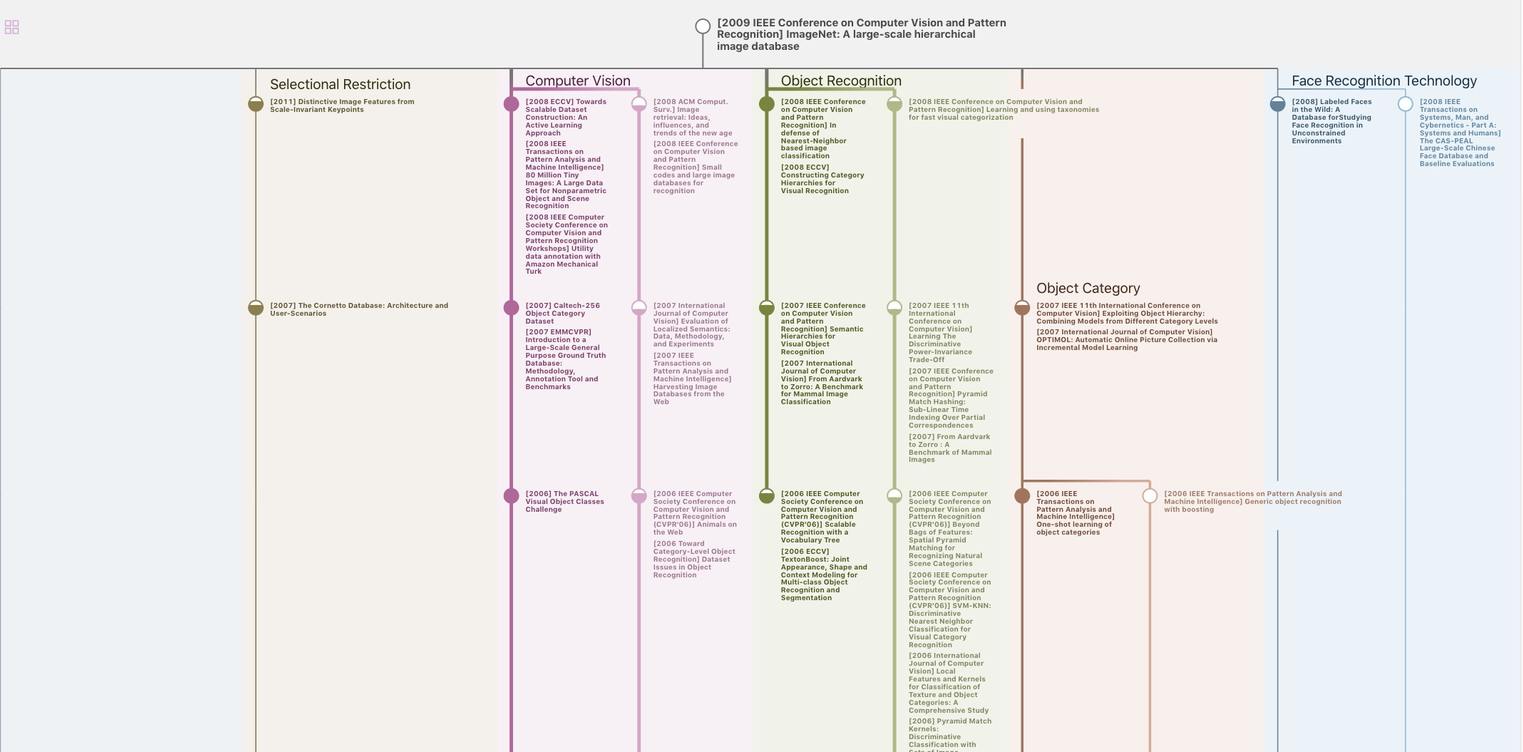
生成溯源树,研究论文发展脉络
Chat Paper
正在生成论文摘要