Practical differentially private online advertising
COMPUTERS & SECURITY(2022)
摘要
A B S T R A C T Powered by machine learning technology, online advertising achieves accurate advertise-ment delivery to potential customers according to online user profiles. However, it raises serious privacy concerns since the learning process may reveal sensitive information in the profiles. It is highly desirable to provide high-quality advertisement recommendations while respecting an individual's privacy. To address the privacy issues, we propose a practical privacy-preserving system that pre-dicts advertisement Click Through Rate (CTR) accurately without revealing any sensitive in-formation of individuals. Our system combines both offline and online training to achieve the goal. In the offline training phase, we develop two differentially private algorithms built upon Gradient Boosting Decision Tree (GBDT) and Field-aware Factorization Machine (FFM) algorithms, respectively, to transform features and train a privacy-preserving offline model. In the online training phase, we propose a privacy-preserving online learning algorithm to compensate for the decline of offline performance when online feature changes occur. We perform extensive experiments to evaluate the performance of our system based on three real-world datasets. The results demonstrate that our system can protect user pri-vacy without compromising the accuracy of CTR prediction, with reasonable computation overhead. (c) 2021 Published by Elsevier Ltd.
更多查看译文
关键词
Differential privacy,Online advertising,Data perturbation,Learning and optimization,Recommendation system
AI 理解论文
溯源树
样例
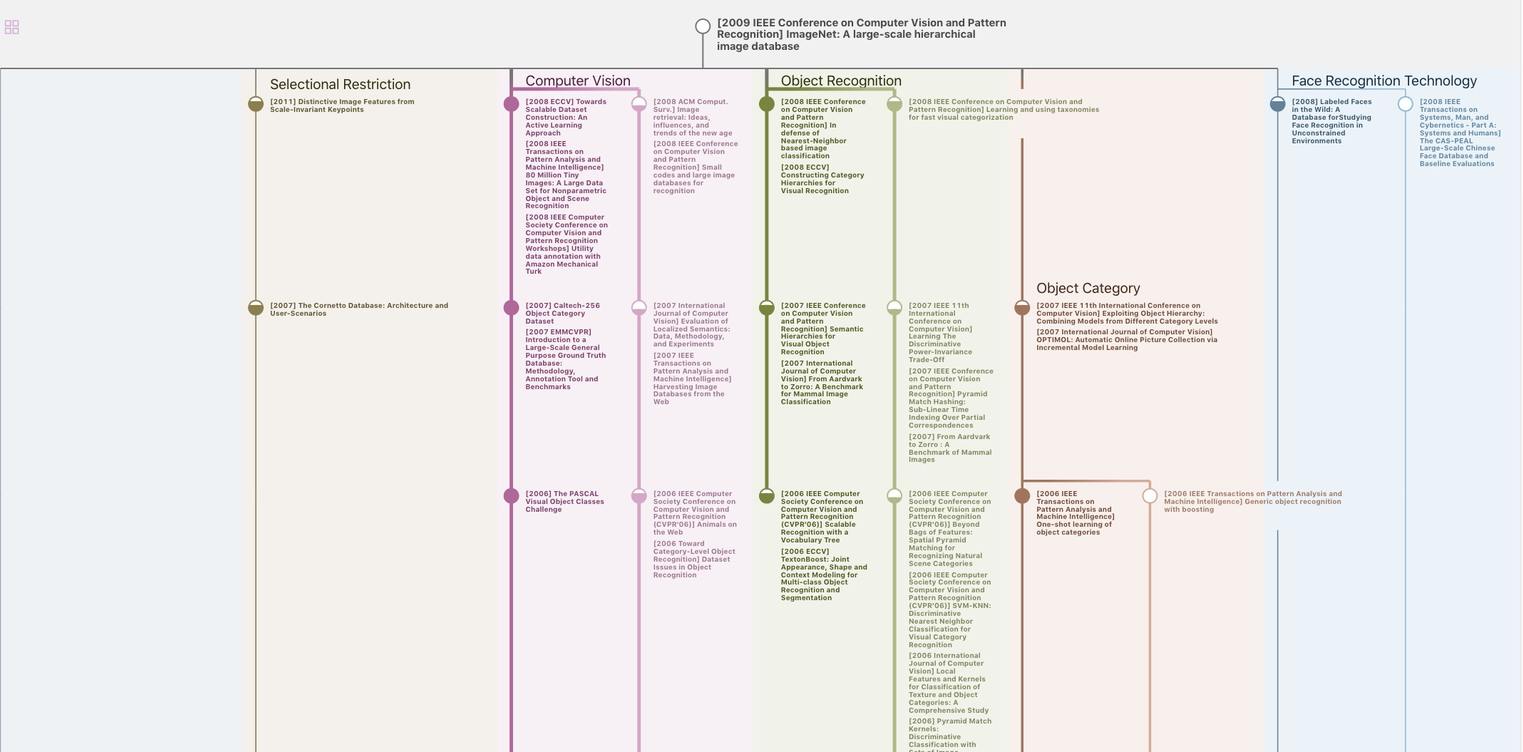
生成溯源树,研究论文发展脉络
Chat Paper
正在生成论文摘要