Evolutionary Dual-Ensemble Class Imbalance Learning for Human Activity Recognition
IEEE transactions on emerging topics in computational intelligence(2022)
摘要
Human activity recognition is an imbalance classification problem in essence since various human actions may occur at different frequencies. Traditional ensemble class imbalance learning methods integrate resampling technique with multi-classifier models, which obtain better generalization than single ones. However, the number of base classifiers is often determined in advance, resulting in the redundant structure of a multi-classifier and larger computational cost. Moreover, various combinations of base classifiers may have similar recognition accuracy, forming a multimodel optimization problem. To address the issue, a dual-ensemble class imbalance learning method is presented, in which two ensemble learning models are nested each other. For each sub-dataset, three heterogeneous sub-classifiers compose of internal ensemble learning model, and the one with the highest recognition accuracy is selected as base classifier. In external ensemble learning model, an optimal combination that contains the smallest number of base classifiers and identifies human actions most exactly is found by multimodal evolutionary algorithms. Based on seven imbalanced activity datasets derived from localization data for person activity, statistical experimental results show that the proposed evolutionary dual-ensemble class imbalance learning method provides the simplest ensemble structure with the best accuracy for imbalanced data that outperforms other five widely-used ensemble classification methods on all metrics.
更多查看译文
关键词
Training,Data models,Learning systems,Feature extraction,Evolutionary computation,Costs,Computational modeling,Class imbalance learning,ensemble learning,under-sampling,multi-modal evolutionary algorithm,human activity recognition
AI 理解论文
溯源树
样例
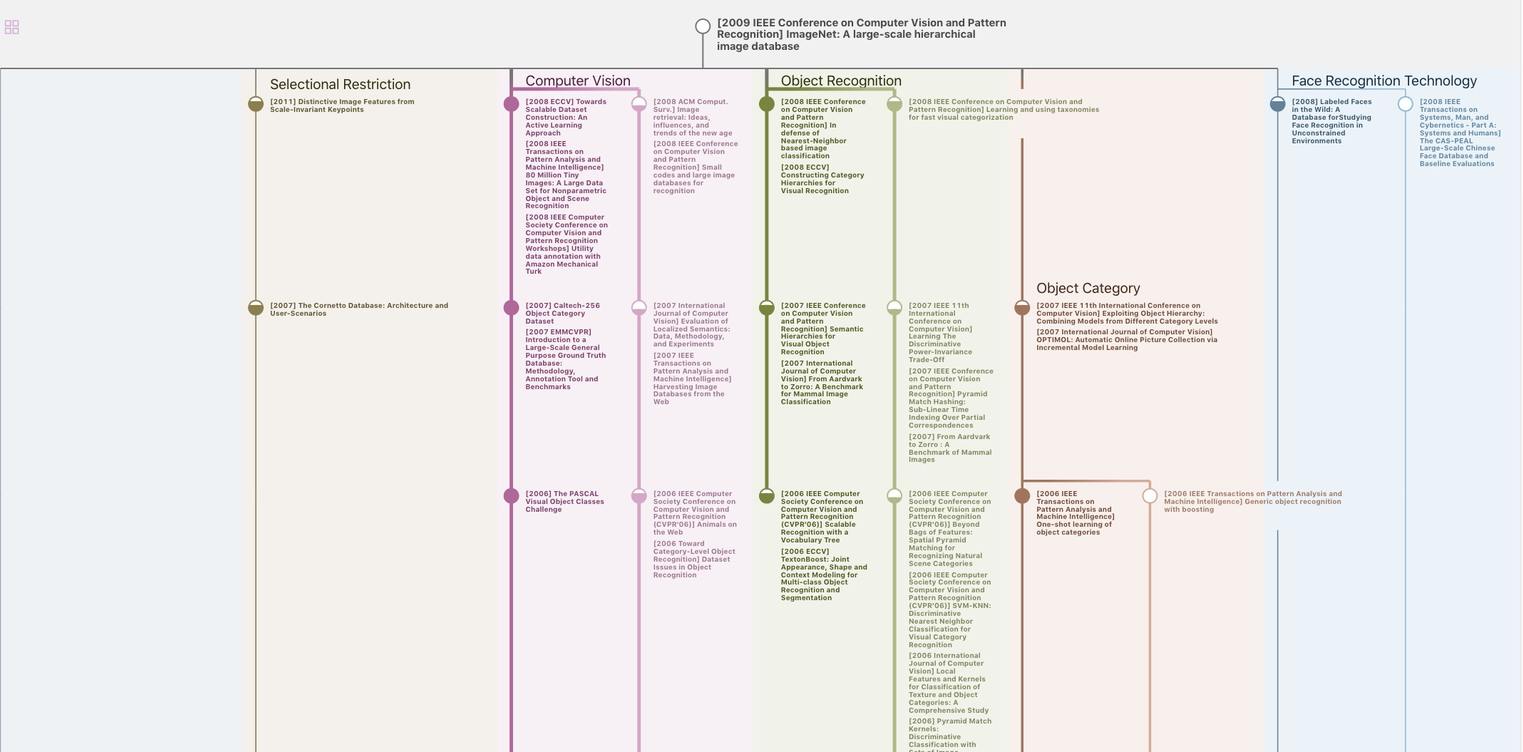
生成溯源树,研究论文发展脉络
Chat Paper
正在生成论文摘要