ML-WiGR: a Meta-Learning-based Approach for Cross-Domain Device-Free Gesture Recognition
SOFT COMPUTING(2022)
摘要
Accurate sensing and understanding of gestures can improve the quality of human–computer interaction and show great theoretical significance and application potentials in the fields of smart home, assisted medical care and virtual reality. WiFi channel state information (CSI)-based device-free wireless gesture recognition requires no sensors and has a series of advantages such as permission for non-line-of-sight scenario, low cost, preserving for personal privacy and working in the dark night. Although most of the current WiFi CSI-based gesture recognition approaches can achieve good performance, they are difficult to adapt to the new domains. Therefore, this paper proposes ML-WiGR, a novel approach for device-free gesture recognition in cross-domain applications. ML-WiGR applies convolutional neural networks (CNN) and long short-term memory (LSTM) neural networks as the basic model for gesture recognition to extract spatial and temporal features. Combined with the meta-learning training mechanism, ML-WiGR can dynamically adjust the learning rate and meta-learning rate in training process adaptively and optimize the initial parameters of a basic model for gesture recognition, only using a few samples and several iterations to adapt to the new domain. In the experiments, the approach is tested under a variety of scenarios. The results show that ML-WiGR can achieve comparable performance against existing approaches with only a small number of samples for training in cross-domains.
更多查看译文
关键词
Device-free gesture recognition,WiFi channel state information,Meta-learning,Deep learning
AI 理解论文
溯源树
样例
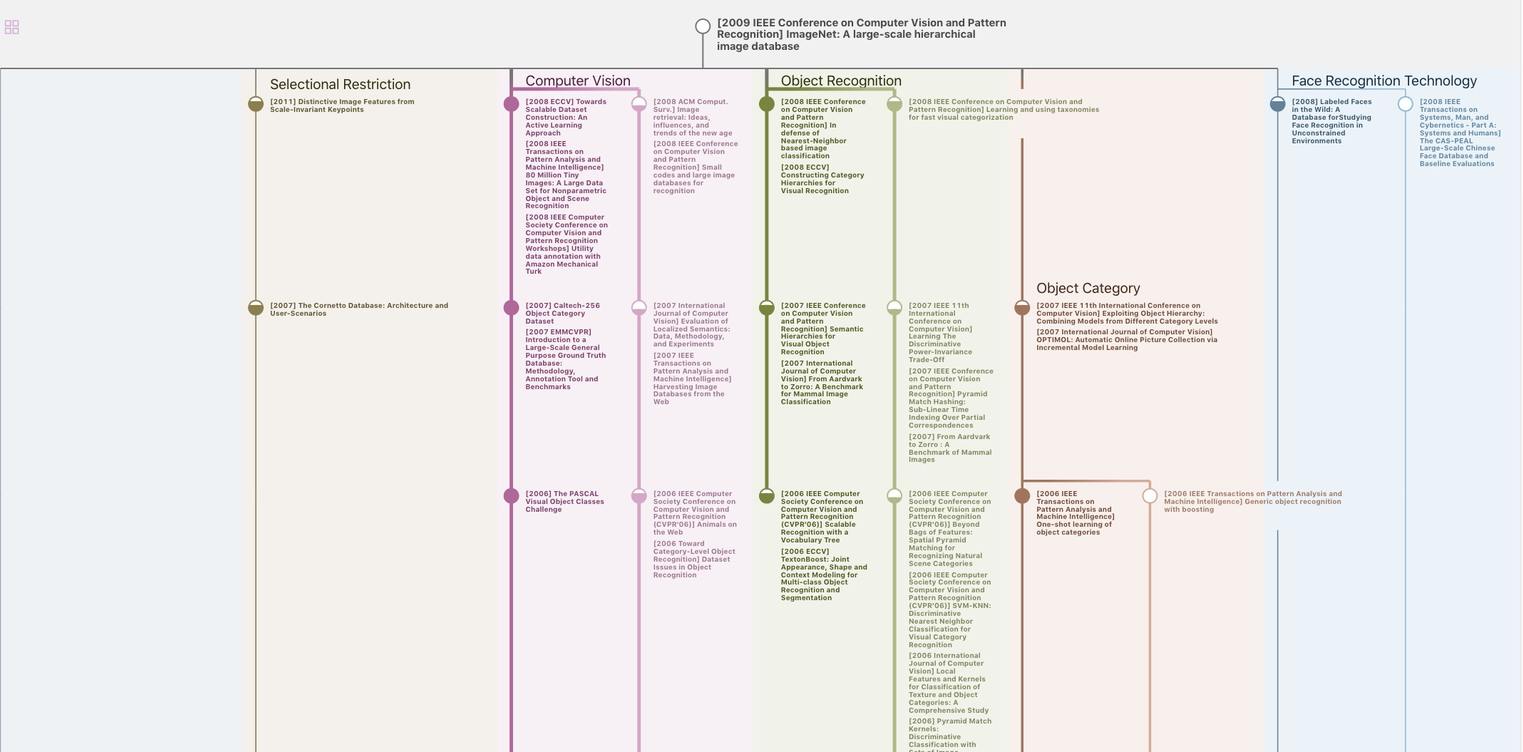
生成溯源树,研究论文发展脉络
Chat Paper
正在生成论文摘要