Gain Enhanced Wide Azimuth Beam Antenna Using Half-Mode Substrate Integrated Waveguide Cavity for Automotive Rear-View Mirror Application
IEEE Transactions on Vehicular Technology(2022)SCI 2区
Korea Adv Inst Sci & Technol | Samsung Elect Mobile Div | Elect & Telecommun Res Inst | Korea Elect Technol Inst
Abstract
This paper presents a gain enhanced wide azimuth beam automotive antenna using half-mode substrate integrated waveguide (HMSIW) cavity for automotive applications. The antenna is separated into two HMSIW cavities by electrical via walls. The two HMSIW cavities are excited through differential feeding, including a 180 degrees delay line in the center of the antenna. The antenna was operated in TM11 modeand investigated using aperture theory based on the cavity model. The antenna size is small enough to mount in the rear-view mirror, which has a long rectangular shape. For comparison, the proposed antenna and a conventional 1 x 2 microstrip square patch antenna array were fabricated to the same dimensions. Then, these antennas were tested to provide validation. The tested results were consistent with the simulation results. The tested results show that the antenna, with the overall dimensions of 70 mm x 35 mm x 1.52 mm (1.38 lambda(0) x 0.69 lambda(0) x 0.03 lambda(0)), operates at 5.9 GHz. The gain of the antenna is 9.49 dB, and the azimuth beam width is 74.0 degrees. The proposed antenna has a wider azimuth beam width (74.0 degrees) than a conventional 1 x 2 square patch array antenna (42.0 degrees) with similar gain and size.
MoreTranslated text
Key words
Wide azimuth beam,HMSIW,TM11 mode,automotive antenna,V2X communications
求助PDF
上传PDF
View via Publisher
AI Read Science
AI Summary
AI Summary is the key point extracted automatically understanding the full text of the paper, including the background, methods, results, conclusions, icons and other key content, so that you can get the outline of the paper at a glance.
Example
Background
Key content
Introduction
Methods
Results
Related work
Fund
Key content
- Pretraining has recently greatly promoted the development of natural language processing (NLP)
- We show that M6 outperforms the baselines in multimodal downstream tasks, and the large M6 with 10 parameters can reach a better performance
- We propose a method called M6 that is able to process information of multiple modalities and perform both single-modal and cross-modal understanding and generation
- The model is scaled to large model with 10 billion parameters with sophisticated deployment, and the 10 -parameter M6-large is the largest pretrained model in Chinese
- Experimental results show that our proposed M6 outperforms the baseline in a number of downstream tasks concerning both single modality and multiple modalities We will continue the pretraining of extremely large models by increasing data to explore the limit of its performance
Upload PDF to Generate Summary
Must-Reading Tree
Example
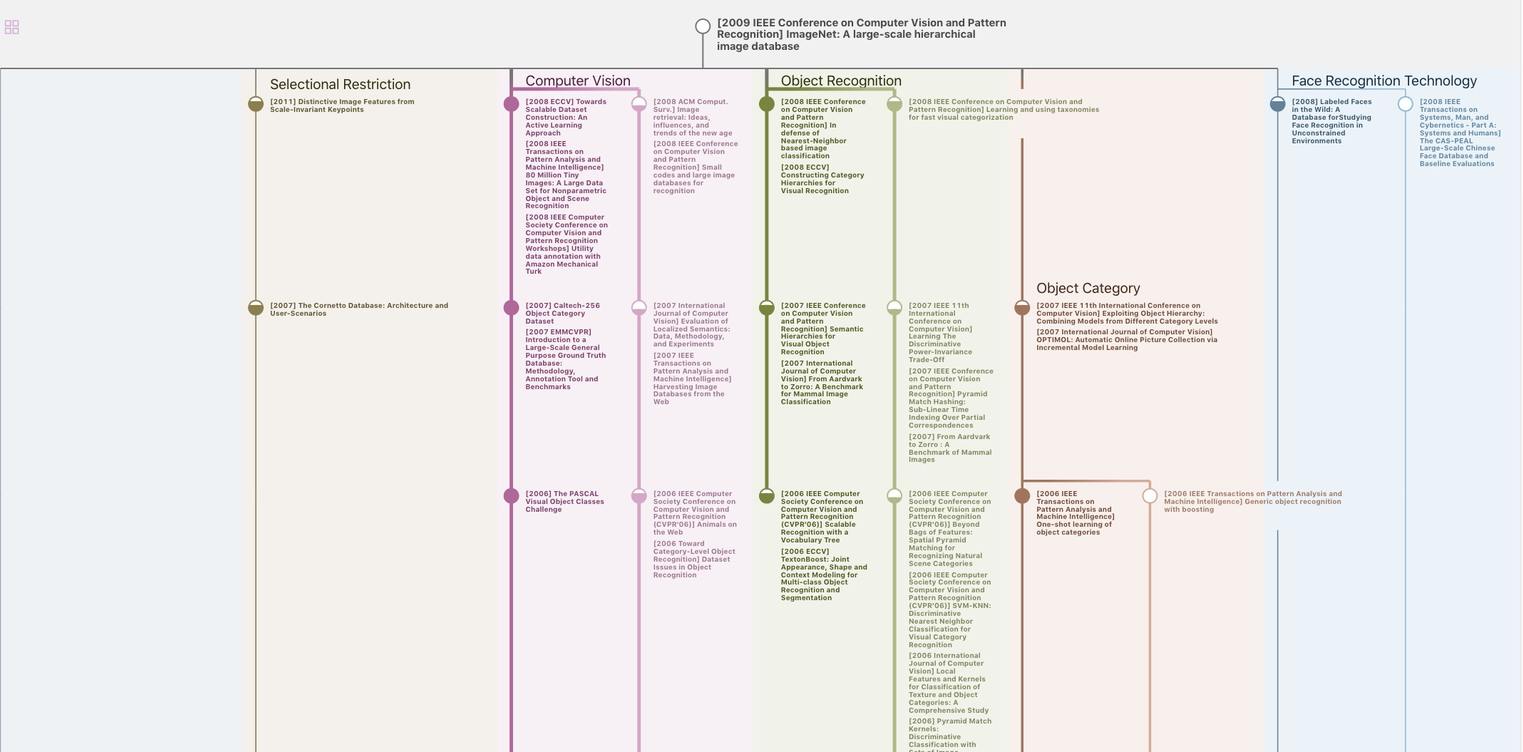
Generate MRT to find the research sequence of this paper
Related Papers
IEEE Transactions on Vehicular Technology 2021
被引用4
The Journal of Engineering 2022
被引用0
Improved HMSIW Cavity for Differential Antenna with High Common-Mode Rejection
IEEE Antennas and Wireless Propagation Letters 2022
被引用1
2022 International Conference on Microwave and Millimeter Wave Technology (ICMMT) 2022
被引用0
Compact High-Gain and Wide-Azimuth-Beam Filtenna for V2X Communication
IEEE Transactions on Antennas and Propagation 2023
被引用0
Widebeam Coverage Antenna Solution for Low-Complexity Mmwave Indoor IoT Network
IEEE INTERNET OF THINGS JOURNAL 2024
被引用0
Compact Planar Phased Array Antenna for Extended V2X Communication Coverage
Alexandria Engineering Journal 2024
被引用1
Data Disclaimer
The page data are from open Internet sources, cooperative publishers and automatic analysis results through AI technology. We do not make any commitments and guarantees for the validity, accuracy, correctness, reliability, completeness and timeliness of the page data. If you have any questions, please contact us by email: report@aminer.cn
Chat Paper
GPU is busy, summary generation fails
Rerequest