Differential Immunogenicity of Homologous Versus Heterologous Boost in Ad26.COV2.S Vaccine Recipients.
Duke NUS Med Sch | Queen Mary Univ London
Abstract
Protection offered by COVID-19 vaccines wanes over time, requiring an evaluation of different boosting strategies to revert such a trend and enhance the quantity and quality of Spike-specific humoral and cellular immune responses. These immunological parameters in homologous or heterologous vaccination boosts have thus far been studied for mRNA and ChAdOx1 nCoV-19 vaccines, but knowledge on individuals who received a single dose of Ad26.COV2.S is lacking. We studied Spike-specific humoral and cellular immunity in Ad26.COV2.S vaccinated individuals (n=55) who were either primed with Ad26.COV2.S only (n=13), or boosted with a homologous (Ad26.COV2.S, n=28) or heterologous (BNT162b2, n=14) second dose. We compared our findings with the results found in individuals vaccinated with a single (n=16) or double (n=44) dose of BNT162b2. We observed that a strategy of heterologous vaccination enhanced the quantity and breadth of both, Spike-specific humoral and cellular immunity in Ad26.COV2.S vaccinated. In contrast, the impact of homologous boost was quantitatively minimal in Ad26.COV2.S vaccinated and Spike-specific antibodies and T cells were narrowly focused to the S1 region. Although a direct association between quantity and quality of immunological parameters and in vivo protection has not been demonstrated, the immunological features of Spike-specific humoral and cellular immune responses support the utilization of a heterologous strategy of vaccine boost in individuals who received Ad26.COV2.S vaccination.
MoreTranslated text
Key words
COVID-19,vaccines,antiviral immunity,Adenovirus vector,heterologous immunity
PDF
View via Publisher
AI Read Science
AI Summary
AI Summary is the key point extracted automatically understanding the full text of the paper, including the background, methods, results, conclusions, icons and other key content, so that you can get the outline of the paper at a glance.
Example
Background
Key content
Introduction
Methods
Results
Related work
Fund
Key content
- Pretraining has recently greatly promoted the development of natural language processing (NLP)
- We show that M6 outperforms the baselines in multimodal downstream tasks, and the large M6 with 10 parameters can reach a better performance
- We propose a method called M6 that is able to process information of multiple modalities and perform both single-modal and cross-modal understanding and generation
- The model is scaled to large model with 10 billion parameters with sophisticated deployment, and the 10 -parameter M6-large is the largest pretrained model in Chinese
- Experimental results show that our proposed M6 outperforms the baseline in a number of downstream tasks concerning both single modality and multiple modalities We will continue the pretraining of extremely large models by increasing data to explore the limit of its performance
Try using models to generate summary,it takes about 60s
Must-Reading Tree
Example
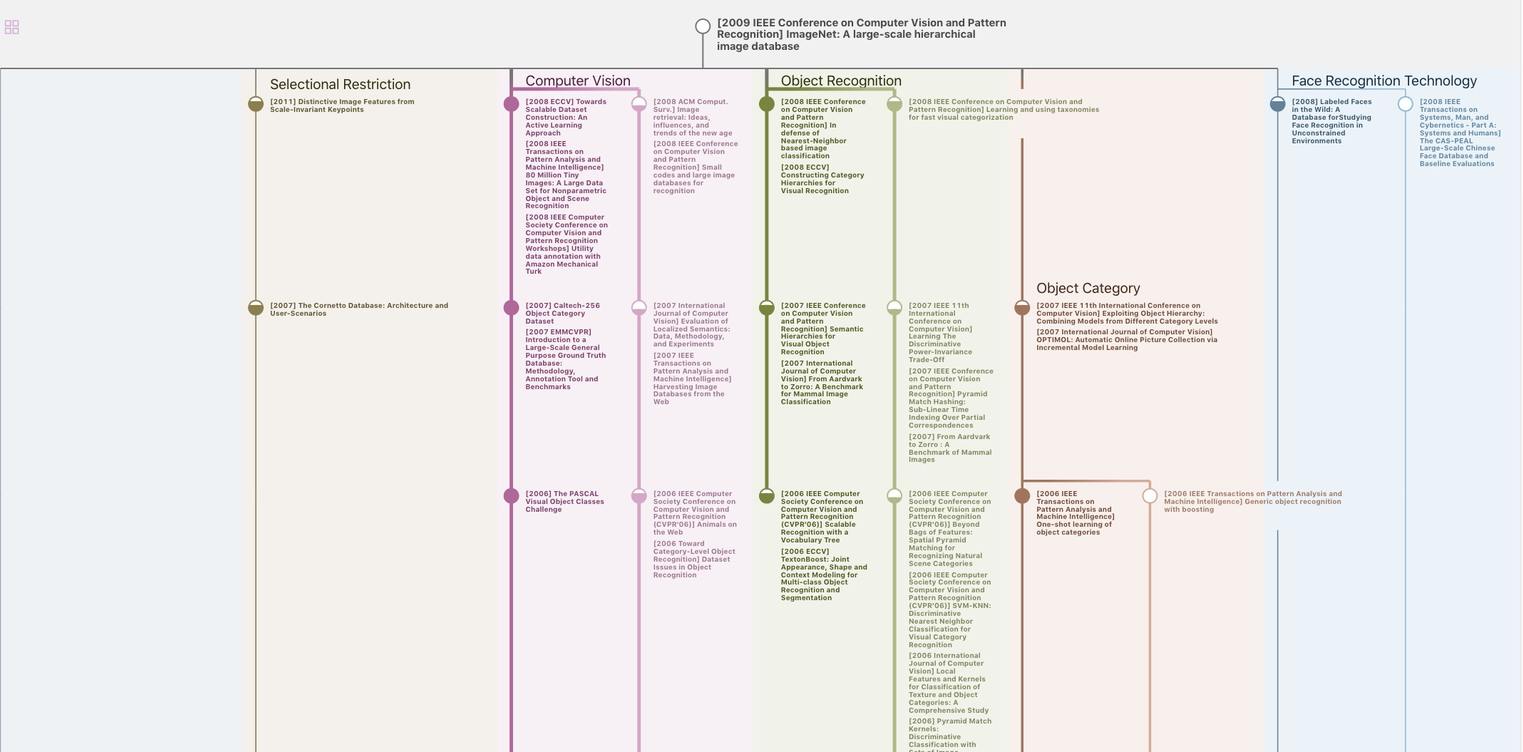
Generate MRT to find the research sequence of this paper
Data Disclaimer
The page data are from open Internet sources, cooperative publishers and automatic analysis results through AI technology. We do not make any commitments and guarantees for the validity, accuracy, correctness, reliability, completeness and timeliness of the page data. If you have any questions, please contact us by email: report@aminer.cn
Chat Paper
去 AI 文献库 对话