A neural architecture generator for efficient search space
Neurocomputing(2022)
摘要
Neural architecture search (NAS) has made significant progress in recent years. However, the existing methods usually search architectures in a small-scale, well-designed architecture space, discover only one architecture in a single search, and hardly rework, which severely limits their potential. In this paper, we propose a novel neural architecture generator (NAG) that can efficiently sample architectures in a large-scale architecture space. Like a generative adversarial network (GAN), our model consists of two components: (1) a generator that can generate directed acyclic graphs (DAGs) as cells or blocks of neural architectures and (2) a discriminator that can estimate the probability that a DAG comes from cells of real architectures rather than the generator. Furthermore, we employ a random search with NAG (RS-NAG) to discover the optimal architecture according to the customized requirements. Experimental results show that the NAG can generate diverse architectures with our customized requirements multiple times after one adversary training. Furthermore, compared with the existing methods, our RS-NAG achieves the competitive results with 2.50% and 25.5% top-1 accuracies on two benchmark datasets – CIFAR-10 and ImageNet.
更多查看译文
关键词
Neural architecture search,Large-scale architecture space,Generative adversarial network,Neural architecture generator,Graph neural network
AI 理解论文
溯源树
样例
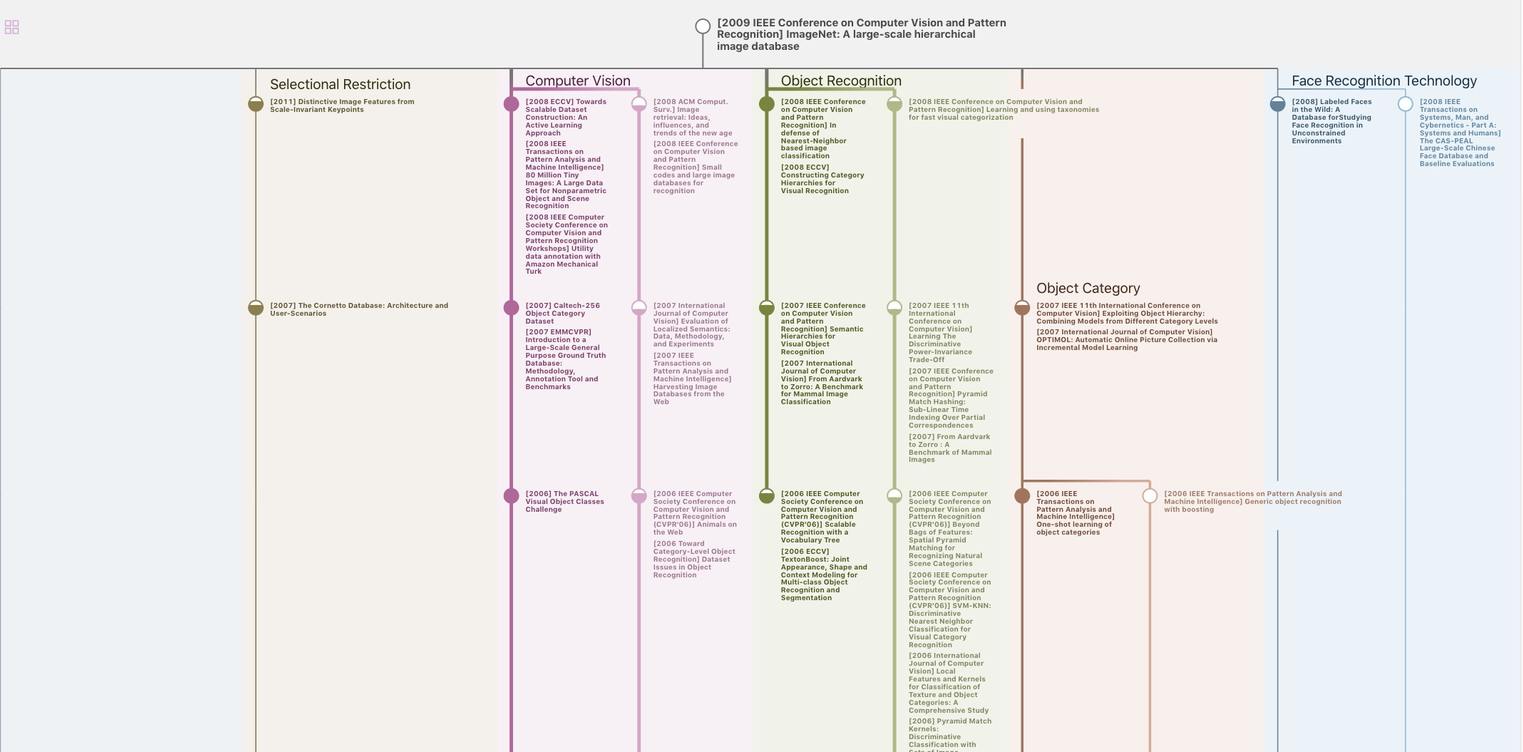
生成溯源树,研究论文发展脉络
Chat Paper
正在生成论文摘要