A Novel Approach to Passively Informed Mental Health Risk Detection in People with Diabetes: Rationale, Methods, and Early Results (Preprint)
semanticscholar(2021)
摘要
BACKGROUND
Proactive detection of mental health (MH) needs among people with diabetes mellitus (DM) could facilitate early intervention, improve overall health and quality of life, and reduce individual and societal health and economic burdens. Passive sensing and ecological momentary assessment are newer methods that may be leveraged for proactive detection.
OBJECTIVE
The primary aim of this study was to conceptualize, develop, and evaluate a novel machine learning approach for predicting MH risk in people with DM.
METHODS
A retrospective study was designed to develop and evaluate a machine learning model, utilizing data collected on 142,432 individuals with DM enrolled in the Livongo for Diabetes program. First, participants’ MH statuses were verified using prescription and medical and pharmacy claims data. Next, passive sensing signals were extracted from participant behavior in the program. Data sets were then assembled to create participant-period instances and descriptive analyses were conducted to understand the correlation between MH status and passive sensing signals. Passive sensing signals were then entered into the model to train and test its performance. The model was evaluated on seven measures: sensitivity, specificity, precision, area under the curve, F1 score, accuracy, and confusion matrix.
Results: In training and 3 subsequent test sets, the model achieved a confidence score of greater than 0.5 in all but three measures.
RESULTS
In training and 3 subsequent test sets, the model achieved a confidence score of greater than 0.5 in all but three measures.
CONCLUSIONS
Results demonstrate the utility of a passively informed MH risk algorithm and invite further exploration.
更多查看译文
AI 理解论文
溯源树
样例
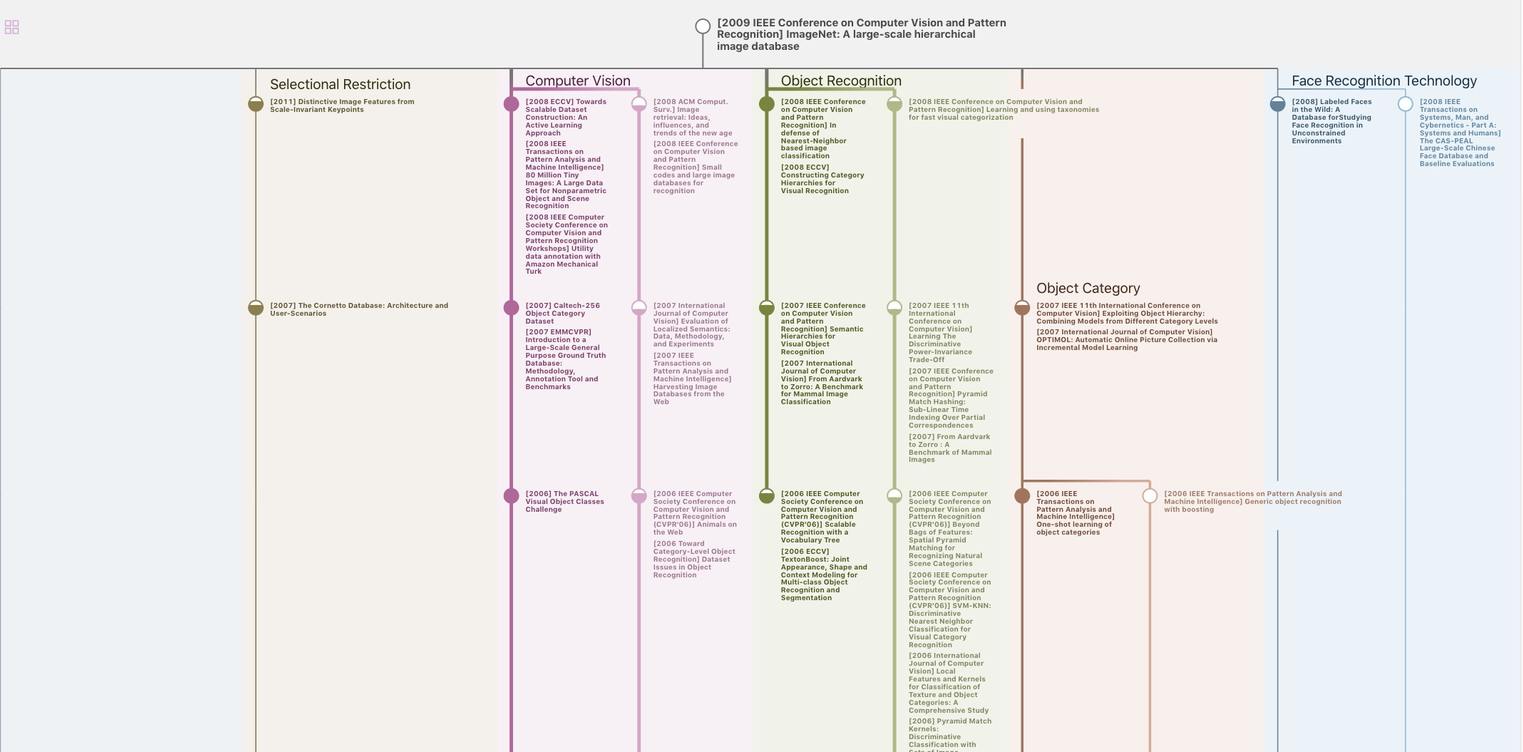
生成溯源树,研究论文发展脉络
Chat Paper
正在生成论文摘要