Self-supervised Learning for Semantic Sentence Matching with Dense Transformer Inference Network
semanticscholar
摘要
Semantic sentence matching concerns predicting the relationship between a pair of natural language sentences. Recently, many methods based on interaction structure have been proposed, usually involving encoder, matching, and aggregation parts. Although some of them obtain impressive results, the simple encoder training from scratch cannot extract the global features of sentences effectively, and the transmission of information in the stacked network will cause certain loss. In this paper, we propose a Densely-connected Inference-Attention network (DCIA) to maximize the use of the feature from each layer of the network by dense connection mechanism and to get robust encoder by self-supervised learning (SSL) based on contrastive method, which can maximize the mutual information between global features and local features of input data. We have conducted experiments on Quora, MRPC, and SICK dataset, the experimental results show that our method owns competitive results on these dataset where we drive 89.13%, 78.1% and 87.7% accuracies respectively. In addition, the accuracy of DCIA with SSL will surpass the one of DCIA without SSL by about 2%.
更多查看译文
关键词
Semantic sentence matching,Self-supervised learning,Dense connection mechanism,Mutual information
AI 理解论文
溯源树
样例
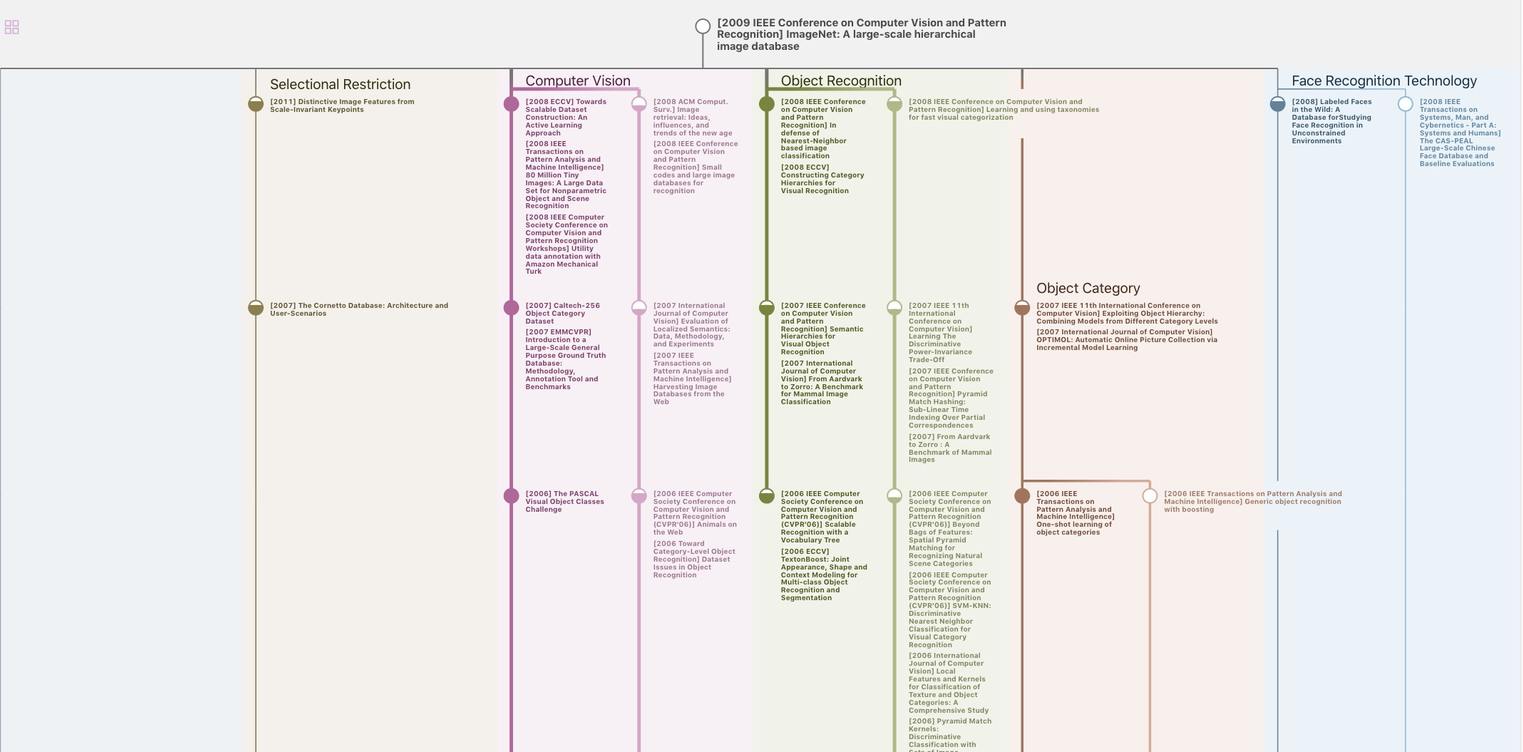
生成溯源树,研究论文发展脉络
Chat Paper
正在生成论文摘要