Applying Machine Learning to Large Databases to Predict Nonresponse to Conventional Treatment in Patients with Crohn's Disease
American Journal of Gastroenterology(2021)
摘要
Introduction: Despite the availability of advanced biologic treatment, patients with Crohn’s disease (CD) continue to cycle through conventional therapies (CTs). We aimed to identify the patients with CD at risk of not responding to CTs using health insurance claims data and a machine learning (ML) approach. Methods: Patients aged ≥18 years with a CD diagnosis and continuous health coverage for 1 year prior and 1 year after CT initiation were identified from Optum Clinformatics claims data (2010-2019). Patients with autoimmune diseases, a diagnosis of ulcerative colitis, or prior exposure to biologic drugs were excluded. The CTs included 5-aminosalicylates, corticosteroids, and immunomodulators. Nonresponse to CT was defined as cessation of CT for any reason, initiation of biologic therapy, prolonged corticosteroid use, CD-related procedure, or CD-related inpatient/emergency visit during follow-up. Over 52,779 baseline features including patient clinical care, specialist visits, diagnoses, medications, procedures, laboratory tests, and demographics for each patient were analyzed. Using an ML approach, models (logistic regression, random forest, gradient boosting, and self-attention and reverse distillation [SARD]) were trained to predict patient nonresponse to CT. The models were trained on data in the 1 year before CT initiation to predict nonresponse to CT within the year after. Cross-validation was carried out across patients to train, validate hyperparameters, and compute holdout performance. Results: In total, 6794 patients with CD who initiated CT were included and 80.9% were categorized as nonresponders. The mean age was 54.4 years and 55.7% were female. The SARD and logistic regression models performed better than random forest and gradient boosting, with an area under the curve of 0.65 (Table 1). Features most predictive of patient nonresponse to CT included, among others, complications of CD, procedures such as computed tomography of the abdomen, outpatient/emergency room visits, and laboratory tests such as C-reactive protein and bilirubin evaluation. Conclusion: Applying an ML approach, nonresponse to CT was predicted with moderate performance from insurance claims data. The results are encouraging for early detection of patients with CD who may not respond to CT. Future research using additional data elements such as comprehensive laboratory values, genetic information, and patient-reported outcomes might help improve the model performance.Table 1.: Clinical Characteristics of IMDC Stratified by Type of Treatment for the Initial IMDC Event.
更多查看译文
关键词
crohns,machine learning,large databases,patients
AI 理解论文
溯源树
样例
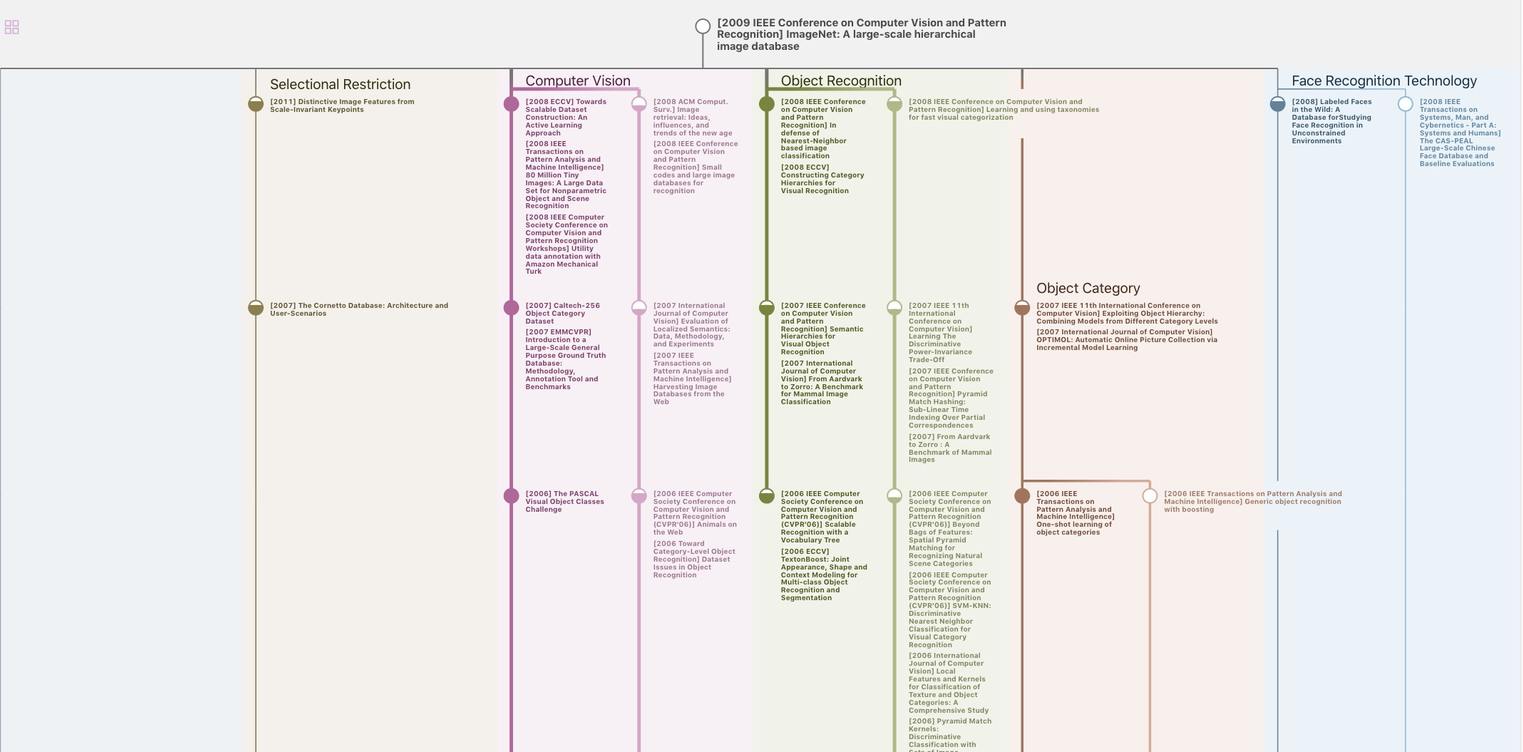
生成溯源树,研究论文发展脉络
Chat Paper
正在生成论文摘要