Sensitivity Analysis of Random Forest Hyperparameters
2021 18th International Conference on Electrical Engineering/Electronics, Computer, Telecommunications and Information Technology (ECTI-CON)(2021)
摘要
Hyperparameter tuning is the process of choosing an optimal set of parameters that govern the learning process of machine learning models. Most modelers spend a lot of time executing hyperparameter tuning on their model. Moreover, there might be no improvement in the performance of the model. This study, thus, performs the sensitivity analysis of the random forest’s popular hyperparameters, namely n-estimator, max depth, min sample leaf, and min sample split, to determine the effect of changes in the hyperparameters on the accuracy and F1-score of the model. One-at-a-time (OAT) and Latin hypercube sampling (LHS) are used together with the analysis of variance (ANOVA). Four datasets with different characteristics are examined. The ANOVA results display both supporting and opposing outputs from the OAT and LHS experiments. The results reported in both experiments suggest that the accuracy and F1-score of the studied models using four distinct datasets are mainly sensitive to min sample leaf.
更多查看译文
关键词
OAT,Latin Hypercube Sampling,Sensitivity Analysis,Random Forest,ANOVA
AI 理解论文
溯源树
样例
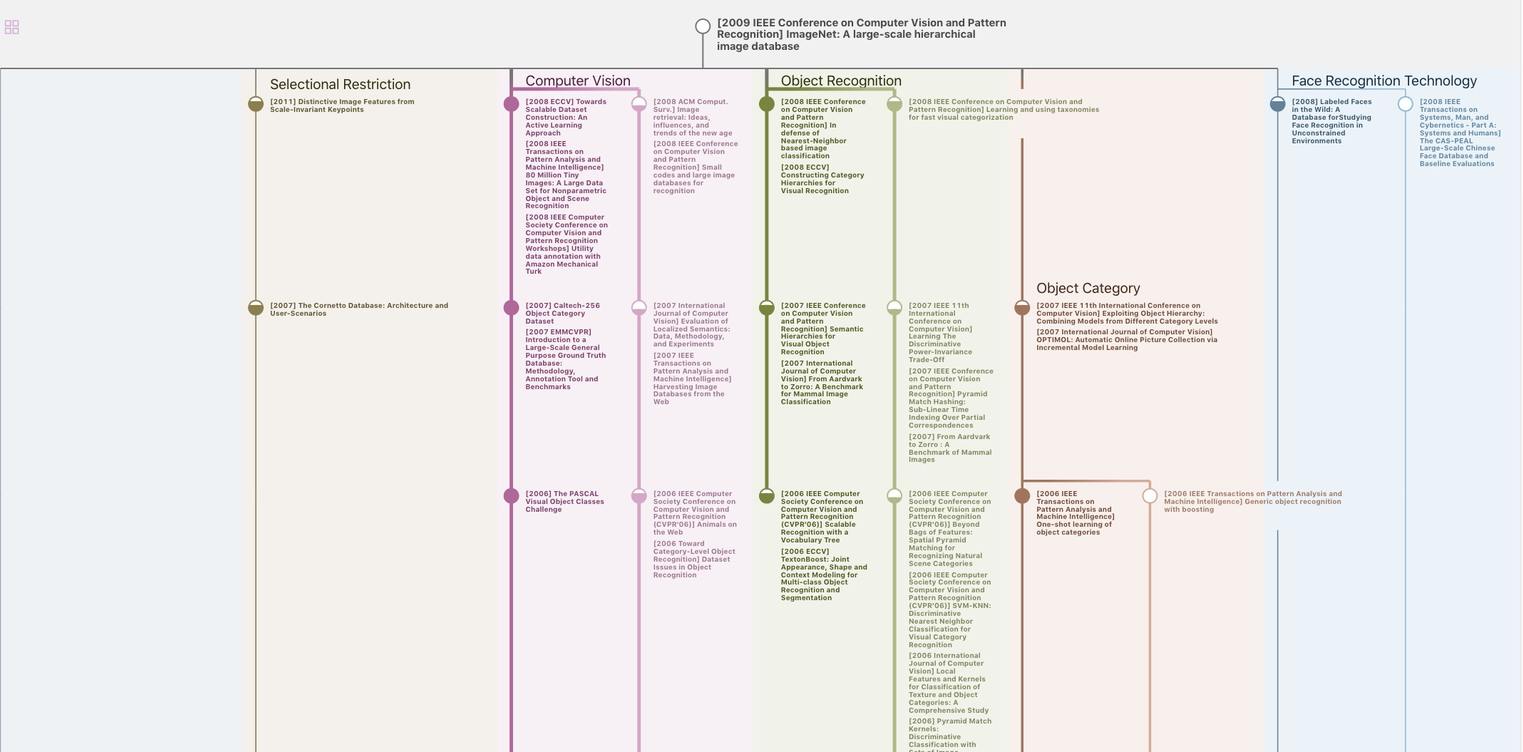
生成溯源树,研究论文发展脉络
Chat Paper
正在生成论文摘要