Development of a Hybrid Machine Learning Model for Asphalt Pavement Temperature Prediction
IEEE ACCESS(2021)
摘要
Machine learning (ML) models are excellent alternative solutions to model complex engineering issues with high reliability and accuracy. This paper presents two extensively explored ensemble models for predicting asphalt pavement temperature, the Markov chain Monte Carlo (MCMC) and random forest (RF). The RF and multiple MCMC (RF-MCMC) were used to hybridise the proposed algorithms for the optimal prediction of asphalt pavement temperature. This study used thermal instruments to measure the asphalt pavement temperature in Gaza Strip, Palestine. The temperature measurements were made at a two-hour interval from March 2012 to February 2013. The temperature data was used to model the pavement temperature. More than 7200 measured pavement temperatures were used to train and validate the proposed models. The validation showed that the ML models are satisfactory. The modelling results ensured the value of the proposed hybridisation models in predicting the asphalt pavement temperature levels. The developed hybrid algorithms regression model achieved acceptable and better prediction results with a coefficient of determination (R2) of 0.96. Generally, the results confirmed the significance of the proposed hybrid model as a reliable alternative computer-aided model for predicting asphalt pavement temperature.
更多查看译文
关键词
Geophysical monitoring,hybridisation algorithms,machine learning,measurement,pavement temperature profile
AI 理解论文
溯源树
样例
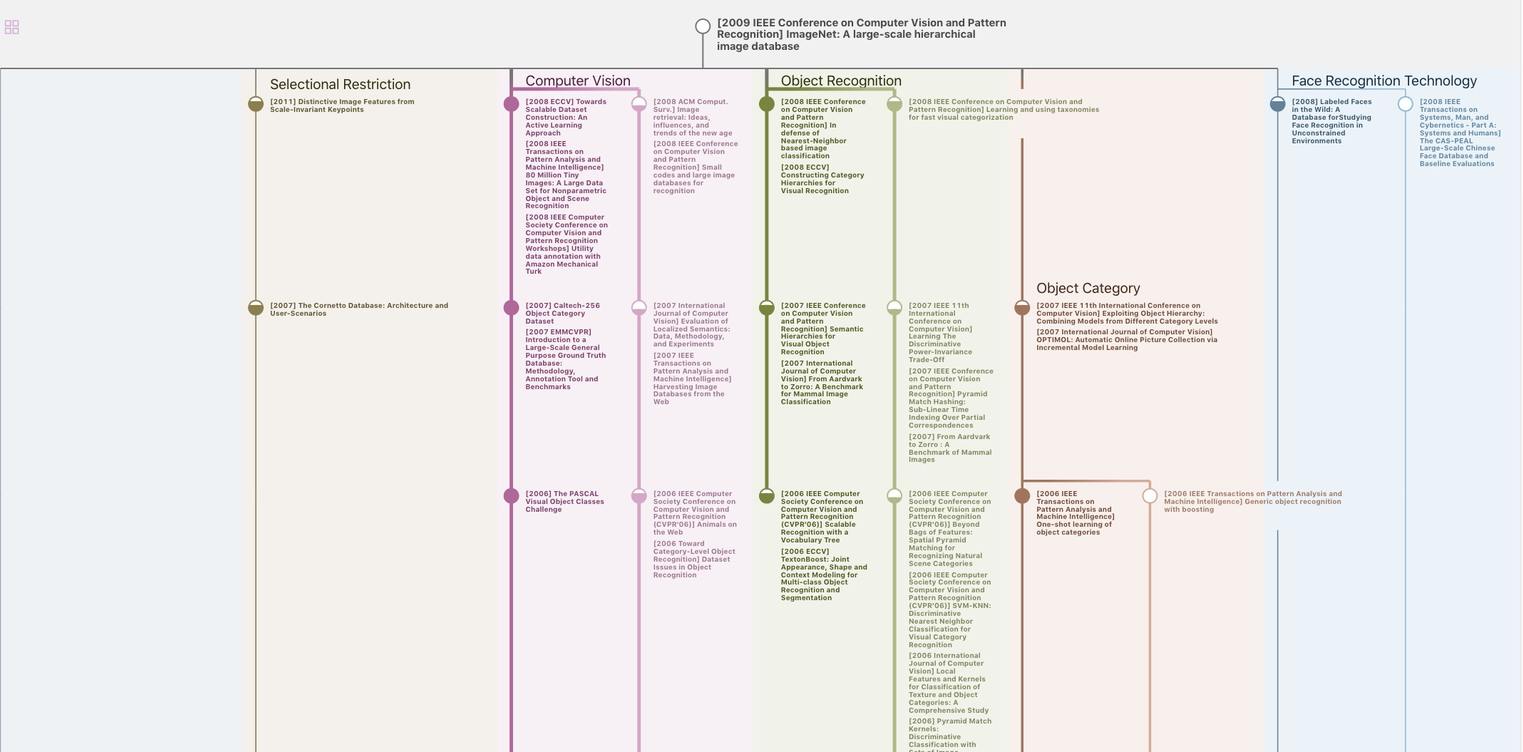
生成溯源树,研究论文发展脉络
Chat Paper
正在生成论文摘要