Efficient method for accelerating line searches using a combined Schur complement domain decomposition and Born series expansions in photonic-based adjoint optimization
arxiv(2021)
摘要
A line search in gradient-based optimization algorithm solves the problem of determining the optimal learning rate for a given gradient or search direction in a single iteration. For most problems, this is determined by evaluating different candidate learning rates to find the optimum, which can be expensive. Recent work has provided an efficient way to perform a line search with the use of the Shanks transformation of a Born series derived from the Lippman-Schwinger formalism. In this paper we show that the cost for performing such a line search can be further reduced with the use of the method of the Schur complement domain decomposition, which can lead to a 10-fold total speed-up resulting from the reduced number of iterations to convergence and reduced wall-clock time per iteration.
更多查看译文
AI 理解论文
溯源树
样例
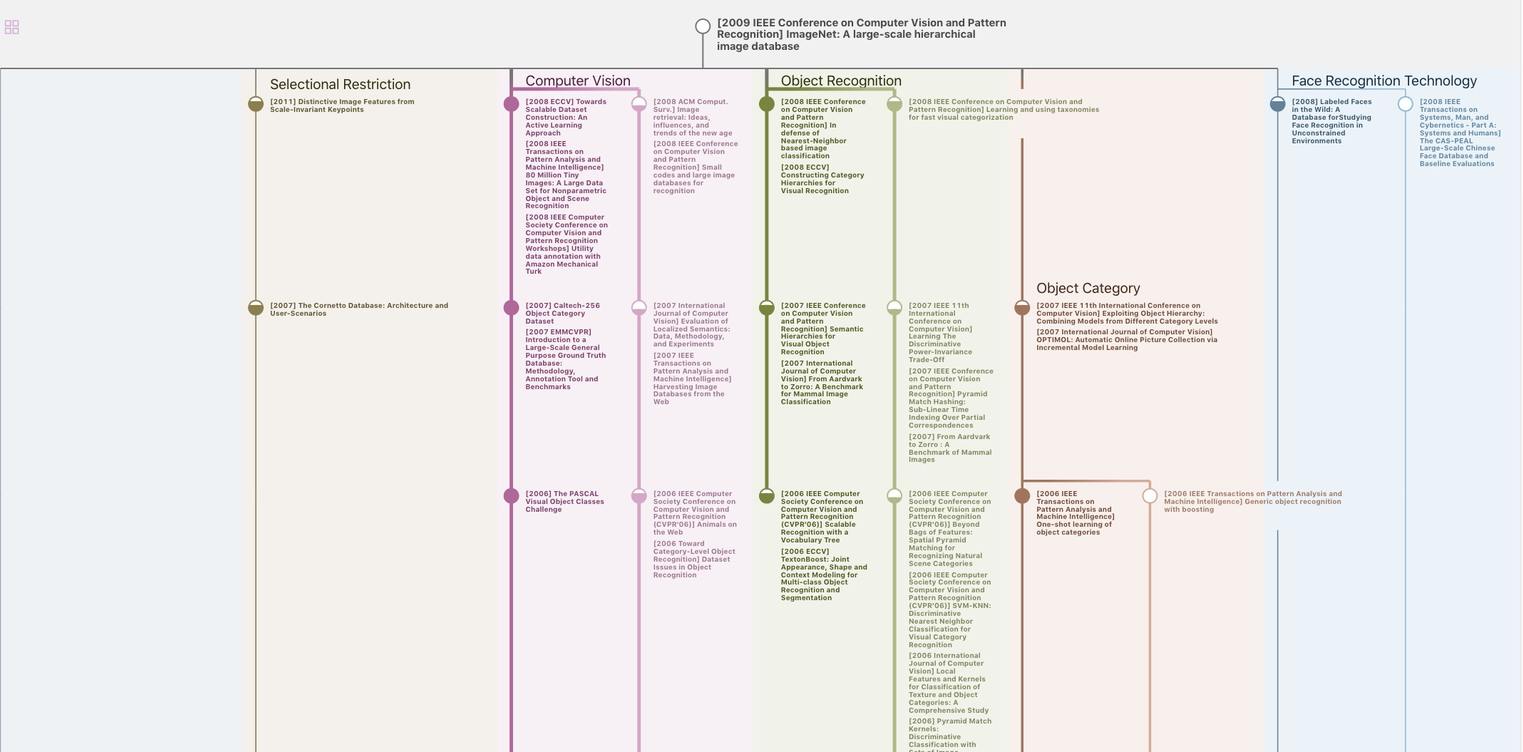
生成溯源树,研究论文发展脉络
Chat Paper
正在生成论文摘要